AI agents for finance: Capabilities, applications and use cases, implementation, and benefits
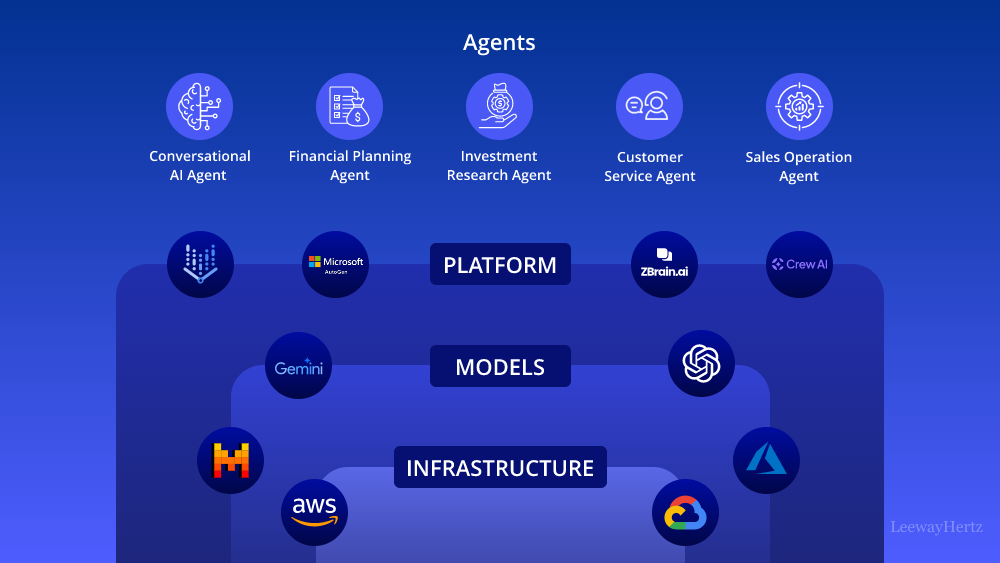
Artificial intelligence is rapidly transforming the finance industry, automating routine tasks and enabling new data-driven capabilities. At the cutting edge of this transformation are AI agents – intelligent software programs that can independently perceive, learn, make decisions, and take actions to achieve specific goals. These agents are not just streamlining operations but are shaping a new era of financial automation that experts believe could reduce operational costs by up to 90%.
Currently, only about 32% of financial institutions harness AI for functions like predictive analytics and speech recognition. However, LLM-based AI agents are set to expand AI’s role across banking, investing, lending, and more. Today’s finance businesses face intense competition and challenges, including significant difficulties in filling roles—with 75% of employers reporting recruitment struggles—and productivity losses of up to 40% due to frequent task switching.
Autonomous AI agents, equipped with vast knowledge and specialized expertise, promise to transform these challenges into opportunities. These self-operating AI systems can collaboratively work together, each with vast knowledge and codified expertise, to handle complex financial operations and customer interactions with little to no human involvement required.
AI agents could enable a new paradigm of “AI-run” financial institutions – self-sufficient digital operations with AI taking on the roles of manager, analyst, accountant, and more. Each agent operates independently based on its training data and programming while also coordinating with other agents to achieve overarching business objectives and tasks.
This autonomous AI revolution holds immense opportunities for the finance industry. Early adopters can gain a competitive advantage through increased efficiencies, elevated customer experiences, and innovative AI-driven services and business models. However, firms must carefully develop and govern these systems to ensure they operate securely, ethically, and in compliance with regulations.
As we explore this new frontier, the insights provided here will guide you through the nuances of AI agents in finance—their types, applications, benefits, and the future trends shaping the sector. You will also discover strategic approaches to implementing AI agents effectively within the financial domain.
- Understanding AI agents and their types
- What are AI agents in finance?
- How ZBrain’s generative AI agents are transforming enterprise operations
- Applications and use cases of AI agents in finance
- Financial AI agents: A detailed look at their architecture and execution
- How are AI financial agents different from standard LLMs
- Key benefits of AI agents in finance
- Building LLM-based AI agents for finance: A step-by-step guide
- How can LeewayHertz help you build AI agents for finance?
- Future trends for AI agents in finance
Understanding AI agents and their types
An AI agent, often called an intelligent agent, is a highly efficient, intelligent virtual assistant that autonomously performs tasks by leveraging artificial intelligence. It is designed to sense its environment, interpret data, make informed decisions, and execute actions to achieve predefined objectives.
A fundamental characteristic of AI agents is their ability to adapt and enhance their capabilities. Utilizing technologies like Large Language Models (LLMs), these agents progressively refine their skills through ongoing interactions, becoming more advanced and effective over time.
Within autonomous AI systems, collaboration is key. Multiple agents work together, each with distinct roles reflecting a specialized team. This cooperative strategy leads to more effective problem resolution, as each agent applies its unique expertise towards a shared goal, streamlining the approach to complex challenges.
Core functions of AI agents
AI agents are designed to handle a variety of tasks that range from simple to highly complex processes:
- Environmental perception: They continuously scan their operational environment to detect and analyze changes, allowing them to respond to new data in real time. Specifically, LLM agents harness the inherent language understanding abilities of LLMs to interpret instructions, context, and objectives. This empowers them to function autonomously or semi-autonomously based on prompts from humans.
- Tool utilization: AI agents employ a diverse array of tools, such as calculators, APIs, and search engines, to gather information crucial for decision-making and task execution.
- Decision-making: AI agents make calculated decisions based on data-driven insights, ensuring actions align with business objectives. Leveraging the deep language understanding of LLMs, AI agents make data-driven decisions and interpret complex instructions and contextual cues. This enables them to perform tasks with a higher degree of autonomy and alignment with strategic business objectives.
- Adaptive learning: They learn from outcomes and refine their strategies, enhancing their efficiency and effectiveness with each task. Beyond basic learning, AI agents utilize LLMs to employ advanced reasoning techniques such as chain-of-thought and tree-of-thought reasoning. These methods allow them to draw logical connections and develop solutions to intricate problems, enhancing their learning from outcomes and refining strategies over time.
- Problem resolution: AI agents excel at identifying solutions to emerging challenges, often preempting issues before they escalate. Their problem-solving capabilities are augmented by LLMs’ ability to understand and manipulate text to create specific outputs like reports, emails, or marketing content.
- Strategic planning: AI agents’ ability to forecast and plan significantly contributes to long-term business planning and resource allocation.
Types of AI agents
Although this article focuses on LLM-powered AI agents such as conversational and task-oriented agents, we will briefly cover all other types of AI agents.
- Conversational agents: Conversational agents leverage advanced natural language processing to simulate engaging human conversations. These agents understand context and nuances, allowing them to generate responses that mimic human interaction. In the finance sector, conversational agents are transforming customer service by handling inquiries such as account balances, transaction history, and loan application processes with high efficiency and personalization.
- Task-oriented agents: Task-oriented agents are designed to achieve specific goals, focusing on efficiency and effectiveness in executing predefined tasks. These AI agents are adept at breaking down complex objectives into actionable steps, utilizing advanced algorithms to navigate workflows and execute tasks. Task-oriented agents in finance focus on specific functions such as automating data entry for transaction processing, executing trades, or managing regulatory compliance reporting.
- Reactive agents: These are the simplest form of AI agents, which operate based on the current state of their environment without retaining any memory of past interactions. They are quick and reliable for tasks that require immediate response to environmental changes. A typical example of a reactive agent includes an automated HVAC system that adjusts temperatures based on current readings. Another example is a fraud detection system in banking that blocks a transaction if it matches certain criteria of fraudulent activity, such as unusual location or high amount.
- Model-based agents: A model-based agent operates by using a model to understand and predict its environment. In partially observable environments, the agent relies on this internal model to handle incomplete information. It keeps track of an internal state that is continuously updated based on new perceptions and the history of previous perceptions. This internal state represents parts of the world that are not directly observable. By maintaining and adjusting this state, the agent can make informed decisions about future actions.
- Goal-oriented agents: These agents are designed to achieve specific objectives. They evaluate the potential consequences of their actions to make the best decisions that align with their goals. For example, a portfolio management AI agent allocates assets and rebalances investment portfolios to maximize returns according to the investor’s risk preference and financial goals. Another example includes a chess-playing agent in AI that aims to win the game.
- Utility-based agents: Utility-based agents are highly effective in complex decision-making environments because they evaluate the desirability of different states based on a utility function. In decision-making contexts, “states” refer to the various conditions or scenarios that could result from different actions taken by an agent. For example, in finance, a state could be the particular mix of bonds and other assets in a portfolio at a given time. This function helps select actions that optimize outcomes, such as maximizing profit or satisfaction. For example, utility-based agents can be employed in portfolio management. Here, an agent might analyze vast amounts of market data and use a utility function to balance the trade-off between risk and return, maximizing the investor’s utility. This could involve adjusting the portfolio by buying or selling assets in real-time as market conditions, risk assessments, and investor preferences evolve.
- Interactive agents: Designed to engage with users, these agents can interpret human input and provide responsive outputs, enhancing customer service and user experience. For example, customer service chatbots on financial institute’s websites assist users by responding to queries about financial products, processing purchases, or guiding them through the entire process.
- Learning agents: Perhaps the most advanced, these agents improve their performance over time, based on experience, adapting their responses based on the outcomes of previous actions. Customer service chatbots in banking learn from each interaction to better understand customer queries and provide more accurate responses over time.
- Knowledge-based agents: Knowledge-based agents in artificial intelligence utilize a repository of structured information and rules to make informed decisions. These agents apply reasoning techniques to this knowledge to solve complex problems and provide expert advice. For instance, in finance, a knowledge-based agent could analyze many investment opportunities and regulatory requirements to offer tailored financial advice or manage compliance. The capability to explain their reasoning processes makes these agents particularly valuable in settings requiring transparency and accountability.
- Cognitive agents: These agents are equipped with advanced decision-making capabilities, utilizing machine learning to analyze and interpret complex datasets. They are ideal for roles requiring analytical depth, such as financial analysis or market predictions. For example, advanced fraud detection systems are used by banks that analyze transaction patterns across vast datasets to identify unusual behavior indicative of fraud.
AI agents are not merely automated tools but are central to developing intelligent systems that mimic human decision-making processes. They offer a blend of adaptability, efficiency, and intelligence crucial for businesses looking to leverage technology to stay competitive in the digital era. As these agents continue to evolve, their potential to transform various industries grows, making them fundamental to the future of AI-driven automation.
Optimize Your Operations With AI Agents
Optimize your workflows with ZBrain AI agents that automate tasks and empower smarter, data-driven decisions.
What are AI agents in finance?
AI agents are becoming indispensable tools in the finance sector, equipped with capabilities that mimic human intuition and analytical prowess. Their application ranges from simple tasks like monitoring asset prices to complex analyses such as scrutinizing detailed financial reports. Unlike standard language models that primarily generate text, AI agents designed for finance are tailored to navigate and interpret vast arrays of financial data, offering insights that are both timely and relevant.
AI agents present a transformative solution by automating a wide range of activities, from routine tasks to complex decision-making processes. This not only enhances decision quality but also accelerates execution, significantly improving overall productivity and reducing human error. Recent studies indicate that 37% of consumers have already experienced benefits from AI-powered financial services, such as assistance from chatbots. Moreover, 86% of CEOs in financial institutions believe that AI is becoming essential to their operations.
Capabilities of AI agents in finance
- Data collection and analysis: AI agents efficiently gather, cleanse, and integrate data from multiple sources, including ERP and CRM systems, social media, and market feeds. They are not mere data processors but act as advanced analysts, providing forecasts and strategic recommendations essential for decision-making. Conversational agents utilize data from various sources to understand context and user preferences, which enables them to provide more personalized and informed responses. For instance, they might analyze transaction histories and customer interactions to offer tailored financial advice.
- Process automation and optimization: Beyond automating routine tasks like invoice processing and compliance reporting, AI agents also optimize these processes. They manage exceptions, errors, and anomalies, enhancing the processes they automate through continuous learning and adaptation. Task-oriented agents excel in automating and optimizing complex financial processes such as loan processing, compliance reporting, and risk management.
- Decision-making and execution: AI agents in finance serve as sophisticated decision-makers. They handle critical financial decisions regarding budget allocation, cash management, risk assessment, and investment strategies, ensuring these decisions are based on robust, data-driven models. Furthermore, they provide transparent explanations for their actions, promoting accountability.
- Collaboration and communication: AI agents facilitate seamless communication and collaboration across various departments and external partners. By serving as a central hub for interaction, they enhance the collective intelligence within the financial ecosystem, ensuring that all stakeholders are aligned and informed. Conversational agents enhance customer service by facilitating effective communication between the financial service providers and customers, ensuring that all interactions are coherent and contextually relevant.
By automating complex processes, enhancing decision-making, and fostering a collaborative environment, AI agents are pivotal in helping financial institutions navigate the challenges of today and seize the opportunities of tomorrow.
How ZBrain’s generative AI agents are transforming enterprise operations
ZBrain AI agents are transforming business operations with intelligent automation and insights. By leveraging large language models (LLMs) and integrating seamlessly into enterprise ecosystems, these agents transcend traditional AI capabilities to offer creative, strategic, and operational advantages.
ZBrain AI agents: Key features and benefits
1. Seamless system integration
ZBrain AI agents integrate smoothly with financial tools like ERP systems, accounting software, and data analytics platforms, ensuring compatibility with your existing workflows.
2. Continuous learning
With a human feedback loop, these agents refine their performance over time, improving accuracy in financial forecasting, compliance checks, and decision-making.
3. Proprietary data utilization
They incorporate your organization’s financial data, enabling precise insights and customized reporting aligned with your business objectives.
4. Low-code workflow customization with Flow
Flow allows you to build logic for tasks like budget allocation, expense tracking, and audit management through multi-step, automated workflows.
5. End-to-end process automation
From data consolidation to financial reporting, ZBrain AI agents handle complete financial workflows autonomously, freeing up your team for strategic planning.
6. Cloud and model agnostic
Designed for flexibility, these agents operate seamlessly on any cloud platform, including AWS, Azure, Google Cloud, or private infrastructure, ensuring scalability and reliability.
By automating critical financial tasks, ZBrain AI agents enhance accuracy, streamline operations, and enable data-driven decision-making in finance.
Applications and use cases of AI agents in finance
AI agents are transforming finance by automating routine tasks, enhancing decision-making, and providing actionable insights. They streamline operations like financial analysis, compliance monitoring, and risk assessment, enabling organizations to focus on strategic growth. Here’s an overview of how these agents are being applied across various financial operations and how ZBrain helps:
Investment advisory
Use case | Description | How ZBrain helps |
Personalized investment plans | Creates tailored investment strategies based on an individual’s financial goals, risk tolerance, and market insights. | ZBrain can analyze user data to generate customized investment plans that align with specific goals and preferences. |
Liquidity planning | Provides insights into optimal payment schedules to maintain liquidity. | The liquidity planning optimization agent can analyze cash reserves, obligations, and payment schedules, automating cash flow assessments. This ensures precise cash management and frees finance teams for strategic decision-making. |
Portfolio optimization | Identifies ideal asset allocations to maximize returns while minimizing risks. | ZBrain can evaluate multiple portfolio options and suggest the best allocations for balanced risk-return outcomes. |
Market analysis | Analyzes real-time market data to deliver insights on stocks and sectors. | ZBrain can monitor market conditions and deliver actionable insights for informed investment decisions. |
Real-time performance tracking | Tracks and reports investment portfolio performance to ensure alignment with financial objectives. | ZBrain can provide dynamic performance dashboards, enabling users to monitor and adjust their investments promptly. |
Insurance claims
Use case | Description | How ZBrain helps |
Claim filing automation | Automates the process of filing insurance claims, reducing manual intervention. | ZBrain can streamline claim filing by extracting and submitting relevant data. |
Fraud detection | Identifies fraudulent claims through pattern recognition and anomaly detection. | ZBrain can analyze historical claims data to detect unusual patterns and flag potential fraud. |
Claim validation | Speeds up the processing of claims by automating decision-making steps. | The insurance claims validation agent can validate healthcare claims, ensuring accurate patient, diagnosis, treatment, and billing information. By detecting discrepancies, it reduces manual workload, improves accuracy, and integrates seamlessly with existing claims systems. |
Document management | Automates the collection and categorization of documents for claims. | ZBrain can organize claim documents from multiple sources, ensuring quick access. |
Claims verification | Verifies the validity of claims through automated cross-checking with databases. | ZBrain cross-references claim information with internal and external databases to verify accuracy. |
Personalized claims assistance | Provides tailored assistance based on customer profile and claim history. | ZBrain delivers personalized claim advice and recommendations based on the customer’s data. |
Settlement optimization | Helps calculate accurate and fair settlements based on claim details. | ZBrain analyzes claim data and historical trends to recommend optimal settlement amounts. |
Invoicing
Use case | Description | How ZBrain helps |
Invoice generation | Automates the creation of invoices based on purchase orders and contracts. | ZBrain’s invoice generation agent automates the creation of customized invoices based on customer billing details, payment terms, and adjustments. This reduces manual errors, speeds up the billing cycle, and improves cash flow management. |
Overdue invoice alert | Automates timely, customized payment reminders and provides insights to reduce accounts receivable balances. | ZBrain’s overdue invoice alert agent can automate timely payment reminders for overdue invoices at preset intervals, ensuring consistent cash flow. It customizes alerts and provides insights into customer responses, helping reduce accounts receivable balances. |
Invoice validation | Automates the invoice-to-purchase order process, ensuring real-time data accuracy and minimizing errors in financial operations. | ZBrain invoice validation agent automates invoice-to-purchase order validation, ensuring accuracy and reducing manual effort. It processes emails, validates data, and updates ERP records in real time, minimizing errors and supporting timely financial operations. |
Invoice collection | Streamline invoice prioritization, automate reminders, and improve collection efficiency, ensuring timely payments and better cash flow management. | The automated invoice collection agent can prioritize and automate invoice tracking and payment reminders, improving cash flow and financial operations. It reduces manual effort, enhances collection rates, and ensures timely follow-ups with clients. |
Invoice reconciliation | Compares invoices against payments and flags discrepancies for review. | ZBrain can reconcile invoices with payment records, quickly identifying discrepancies automatically. |
Invoice summarization | Automates invoice categorization and summarization, improving accuracy and operational efficiency. | The client invoice summarization agent can automatically categorize and summarize client invoices, eliminating manual reviews. This enhances accuracy, speeds up invoice processing, and improves cash flow management, boosting overall operational efficiency. |
Client payment scheduling
Use case | Description | How ZBrain helps |
Payment schedule automation | Automatically suggests payment schedules based on terms, cash flow forecasts, and client history. | ZBrain’s client payment scheduling agent can create optimal payment schedules by analyzing client terms, payment history, and cash flow needs. This reduces manual effort, ensures timely payments, and enhances cash flow predictability. |
Payment status | Automates real-time payment updates, improving accuracy, cash flow management, and system integration. | The customer payment status agent can automatically update payment statuses in real-time, reducing manual tracking and enhancing cash flow management. |
Late payment follow-up | Automates overdue invoice classification and personalized follow-ups, enhancing collections and reducing bad debt. | The late payment follow-up agent automatically classifies overdue invoices and generates personalized follow-up messages, improving collections and reducing bad debt. This automation streamlines collections management, allowing finance teams to focus on strategic tasks and strengthen client relationships. |
Client payment tracking | Automates payment classification and real-time tracking, improving efficiency and cash flow management. | The client payment tracking agent automatically classifies payment statuses, eliminating manual updates and enabling efficient cash flow management. Its real-time tracking ensures timely financial reporting and enhances overall payment monitoring efficiency. |
Payment dispute resolution | Automates invoice dispute classification and resolution, improving efficiency and vendor relations. | The payment dispute resolution agent automatically classifies disputed invoices and suggests resolutions, streamlining the dispute resolution process. This improves efficiency, accelerates issue resolution, and enhances vendor relations by reducing manual intervention. |
Regulatory filing
Use case | Description | How ZBrain helps |
Regulatory filing | Automates preparing and submitting financial reports to comply with regulatory requirements. | The regulatory filing automation agent can categorize financial data into relevant sections, eliminating manual data entry and ensuring precision. This streamlines reporting, allowing finance teams to focus on strategic analysis while ensuring timely compliance and avoiding penalties. |
Data verification | Verifies the accuracy and completeness of financial data before filing. | ZBrain can cross-check financial data against regulatory standards to ensure compliance. |
Compliance risk assessment | Automatically categorizes data and detect risks, enhancing accuracy and operational efficiency in compliance management. | The compliance risk assessment agent can automatically categorize transaction data, financial contracts, and activities into risk categories, improving accuracy and efficiency. This automation allows compliance teams to focus on risk mitigation while reducing compliance failures. |
Capital expenditure monitoring | Classifies project data into budget and schedules categories, automating CAPEX analysis. This enables finance teams to focus on strategic planning while ensuring better control over capital spending and accurate financial planning. | The CAPEX compliance monitoring agent can classify project data into budget and schedule categories, automating CAPEX analysis. This enables finance teams to focus on strategic planning while ensuring better control over capital spending and accurate financial planning. |
VAT compliance monitoring | Automates VAT reporting, ensuring accuracy and reducing compliance errors. | The VAT compliance monitoring agent can automate the classification and organization of transaction data and tax filings, ensuring accurate VAT reporting. This reduces compliance errors, enabling finance teams to focus on strategic planning while streamlining VAT management. |
Taxation
Use case | Description | How ZBrain helps |
Tax compliance | Ensures businesses adhere to tax laws and regulations by automatically monitoring and updating tax requirements. | ZBrain’s tax compliance validation agent can automatically categorize and validate tax information (e.g., VAT, GST) for compliance, streamlining the process and reducing manual tasks. It ensures accuracy by cross-referencing tax details with legal requirements and flagging discrepancies for timely resolution. |
Tax auditing | Analyzes financial records and identifies discrepancies for audits. | ZBrain can analyze transaction data to flag inconsistencies and potential areas of concern, streamlining the audit process. |
Tax filing automation | Automates the process of preparing and filing tax returns, reducing human error. | ZBrain automates tax form preparation and filing, ensuring accuracy and timely submissions. |
Tax optimization | Identifies tax-saving opportunities and suggests strategies to minimize liabilities. | ZBrain uses data analytics to suggest tax-efficient strategies, helping businesses optimize their tax position. |
Corporate tax review | Automates tax compliance reviews, ensuring accuracy, reducing audit risks, and optimizing tax positions. | ZBrain’s corporate tax review agent can automate tax document categorization, ensuring compliance and reducing manual reviews. It identifies discrepancies and unclaimed deductions, optimizing tax positions while minimizing audit risks and enhancing financial reporting accuracy. |
Treasury management
Use case | Description | How ZBrain helps |
Cash flow monitoring | Analyzes cash inflows and outflows to optimize liquidity management. | ZBrain’s cash flow monitoring agent can classify and track cash inflows and outflows, automating manual monitoring tasks. It ensures accurate real-time cash flow insights, enhancing liquidity management and enabling strategic financial planning. |
Investment management | Manages short-term investments to maximize returns while maintaining liquidity. | ZBrain can evaluate investment options and suggest optimal strategies to enhance returns while meeting liquidity needs. |
Debt management | Tracks and manages debt obligations to minimize costs and risks. | ZBrain can automate debt tracking and provide insights on refinancing or repayment options to reduce financial burdens. |
Risk mitigation | Identifies and mitigates financial risks related to currency, interest rates, and liquidity. | ZBrain can monitor financial risks in real time and offers actionable insights to protect against potential losses. |
Treasury reporting | Generates detailed reports for treasury operations and performance. | ZBrain automates the preparation of treasury reports, providing real-time insights for decision-making. |
Financial AI agents: A detailed look at their architecture and execution
The architecture of a financial AI agent is designed as a multi-layered system, each dedicated to specific aspects of AI processing and application in the financial sector. The key layers include the financial AI agents layer, financial LLMs algorithms layer, LLMOps and DataOps layers, and a multi-source LLM foundation models layer. These layers work in concert to provide robust, real-time financial analysis and decision-making support.
Here’s a brief overview of each layer:
Financial AI agents layer
This layer incorporates financial Chain-of-Thought (CoT) prompting to enhance decision-making capabilities. Agents like market forecasting agents and document analysis agents utilize CoT to break down financial problems into logical steps, providing precise and actionable insights that align with the latest market dynamics.
Financial LLMs algorithms layer
Here, specifically tuned LLMs such as FinGPT are employed for targeted financial tasks and regional market analyses. This layer also integrates multimodal models that combine textual and visual data processing with traditional machine learning methods to optimize functions like market forecasting and financial document analysis.
LLMOps and DataOps layers
These layers manage the selection and integration of various LLMs based on their performance. The LLMOps layer dynamically adjusts model deployment to ensure the most effective solutions for given financial scenarios. Concurrently, the DataOps layer handles the real-time processing of financial data, which is essential for maintaining responsiveness to market changes.
Multi-source LLM foundation models layer
This foundational layer supports the seamless integration and updating of diverse LLMs, facilitating the system’s adaptability to rapid shifts in financial technologies and market demands. This foundational layer ensures that all models are current, optimized, and aligned with the latest financial technologies and data standards, supporting a seamless plug-and-play functionality for various LLMs. This facilitates the system’s adaptability to evolving financial market demands and enhances operational efficiency.
Understanding the working of a financial AI agent
This workflow is orchestrated within the financial AI agents layer, which serves as the core operational environment for executing sophisticated financial tasks. The AI agents layer consists of domain-specific AI agents tailored to enhance financial analysis through advanced data perception, cognitive processing, and dynamic action execution:
User interaction
- A user or an automated proxy, UserProxy, interacts with the system by initiating a query or request. This interaction is the starting point for the financial AI agent’s operational workflow.
- The system employs preliminary NLP techniques to comprehend the user’s intent and ensure the query is adequately understood, which allows for a tailored analytical process.
Perception layer
- Data sourcing: Financial data is collected from various APIs, including OpenBB, FinnHub, and others, encompassing market news and financial records.
- Data processing: Initial processing to discern critical textual and visual information, preparing it for deeper analysis.
Cognitive processing layer (Brain)
The core ‘Brain’ of the system utilizes advanced Large Language Models (LLMs) to analyze the structured data.
- Analysis and decision making: Utilizes advanced LLMs for deep analysis. Chain-of-thought processes are applied here to formulate structured instructions based on the financial data interpreted.
- Instruction generation: Generates detailed instructions that direct subsequent actions within the financial landscape.
Action layer
Role-based execution: Directed by structured instructions from the Brain, various roles, such as Director, Assistant, and LLM Analysts, implement specific actions. These include portfolio adjustments and financial report generation.
- Director: Oversees the strategic management of tasks and resources.
- Assistant: Assists in preliminary data management and supports analysts by preparing datasets.
- LLM analysts: Conduct detailed financial analyses and synthesize findings to support decision-making processes.
Inner reflection
Allows for self-assessment and continuous improvement of the system
- Self-assessment mechanism: The system evaluates the accuracy and effectiveness of its actions or reports using predefined metrics or feedback loops.
- Adaptive learning: This critical phase allows the AI to learn from past actions, optimizing algorithms and decision-making processes for improved future performance.
- Error correction and optimization: The system identifies and corrects errors or inefficiencies, adjusting operational parameters and updating models to enhance reliability.
Response
The system provides the analyzed information or executed actions back to the user.
- Output delivery: Once the processing is complete, the system formulates a response that may include textual information, such as financial advice or market analysis or actions, like processed transactions.
- Feedback incorporation: Users can provide feedback on the adequacy and accuracy of the response received. This feedback is crucial for the system’s learning and adaptation.
The seamless integration and functionality within this layered architecture ensure financial AI agents can efficiently process, analyze, and respond to complex financial data, enhancing decision-making and operational efficiency in real-time financial environments. This sophisticated architecture enables AI agents to efficiently handle complex, dynamic financial environments. These systems provide precise, actionable insights crucial for modern financial decision-making by leveraging advanced AI techniques and a structured multi-agent approach.
How are AI financial agents different from standard LLMs?
AI financial agents are transforming the finance sector with capabilities that extend well beyond those of standard Large Language Models (LLMs). Designed specifically for financial applications, these agents don’t just interpret data; they provide expert advice and deliver strategic insights in real-time.
Standard LLMs, trained on extensive datasets, are typically employed for content generation, translation, and simple question-answering. While adept in these areas, they often lack the necessary financial context and expertise, struggling with the nuances of financial data, which can lead to inaccurate responses.
In contrast, AI financial agents are equipped with advanced reasoning and decision-making skills tailored specifically for financial contexts. They analyze vast amounts of data, interpret complex market conditions, and offer actionable financial advice using sophisticated algorithms crafted for financial decision-making. Moreover, these agents prioritize compliance and security, ensuring that their operations align with current financial regulations and standards—critical for anyone involved in financial planning or management.
Aspect | Standard LLMs | AI Financial Agents |
---|---|---|
Focus | Content generation, translation, and basic question-answering. | Providing expert financial advice, strategic insights, and actionable recommendations. |
Expertise | Trained on vast datasets across various domains, offering broad knowledge. | Tailored for financial contexts with specialized knowledge of market dynamics, investment strategies, and regulatory frameworks. |
Capabilities | Capable of handling a wide range of general tasks, including text generation and simple data processing. | Analyze complex market conditions, interpret financial data, and offer personalized financial planning, portfolio management, and risk assessment. |
Decision-making | Performs well in pattern recognition and information retrieval across diverse topics. | Employs advanced reasoning and decision-making algorithms specifically designed for nuanced financial contexts. |
Compliance | General compliance features are suitable for a wide range of applications. | Prioritize compliance and security, ensuring operations align with strict financial regulations and industry standards. |
Advantages | Broad applicability in diverse fields beyond finance, providing flexibility and general utility. | Real-time insights, personalized recommendations, increased efficiency in financial operations, and reduced risk through advanced algorithms and specialized financial data analysis techniques. |
Optimize Your Operations With AI Agents
Optimize your workflows with ZBrain AI agents that automate tasks and empower smarter, data-driven decisions.
As the finance sector evolves, the role of AI financial agents is becoming increasingly indispensable, driving innovation and efficiency where accuracy and timeliness are paramount. On the other hand, Large Language Models (LLMs) continue to serve as a versatile foundation for a broad range of applications, complementing specialized agents by providing extensive general knowledge and capabilities.
Key benefits of AI agents in finance
Here’s a detailed exploration of the substantial advantages AI agents offer within the financial sector:
Enhanced operational efficiency: Manual processes often bog down finance teams, with about 24% of them struggling with inefficiencies and 27% lacking desired skills that affect overall productivity. AI agents tackle this issue head-on by automating both front-end customer-facing services and back-end operations such as loan processing and regulatory compliance. This automation frees up human resources for more strategic work and ensures that more tasks are completed accurately and swiftly, significantly boosting operational efficiency.
Cost-effective lead generation: Generating leads in the financial sector can be quite costly, with an average investment of $160 per financial service lead. AI agents can dramatically reduce this cost by employing predictive analytics to identify and engage with potential customers who are most likely to convert, optimizing the entire lead generation process. Furthermore, AI agents help tailor marketing strategies to ensure targeted leads receive relevant information, increasing the likelihood of conversion.
Reduced operational costs: Reliance solely on human resources for delivering services often leads to high costs associated with hiring, training, and skill development. AI agents mitigate these costs by automating low-risk and repetitive tasks, reducing the need for extensive human intervention. This lowers operational costs and enhances accuracy and efficiency across critical workflows such as marketing, and customer management, further minimizing errors and delays that could result in financial losses.
Improved customer satisfaction: With 57% of investors preferring to interact with a live agent before making a purchase, the quality of customer interaction becomes crucial. AI agents enhance this interaction by processing vast amounts of data to provide AI agents with comprehensive insights into a customer’s past investments, preferences, and patterns. This enables personalized and informed interactions, reducing response times and fostering a customer-centric support system where clients feel valued and understood.
Advanced risk management: Effective risk management is pivotal in finance, yet many organizations struggle with it due to inadequate data analysis and inherent biases. AI agents excel in extracting data-driven insights from extensive financial datasets, allowing for precise risk assessment. This objective analysis helps financial institutions and their clients make informed decisions regarding credit, loans, and other investment areas, significantly enhancing the accuracy of risk management.
Streamlined compliance and monitoring: The financial sector is heavily regulated, and compliance with these regulations is both critical and challenging. AI agents automate and streamline compliance processes, such as monitoring transactions for suspicious activities and maintaining up-to-date records for audits.
24/7 availability and support: AI agents operate continuously without the constraints of human operational hours, providing round-the-clock service that enhances customer experience and satisfaction. Whether it’s responding to inquiries, executing transactions, or updating accounts, AI agents deliver prompt and reliable service at any time of the day, any day of the week.
Enhanced data processing: AI agents automate routine financial operations and excel in processing and analyzing large volumes of data rapidly and accurately. Their ability to work without fatigue or bias results in highly informed decision-making. By leveraging advanced machine learning techniques, AI agents can detect patterns, trends, and anomalies crucial for risk assessment, investment strategies, and detecting fraud, thereby significantly enhancing operational efficiency.
Personalized financial services: AI agents personalize financial services by analyzing individual preferences, risk tolerances, and financial goals to offer tailored investment options and banking services. This customization enhances consumer experiences by ensuring that the financial advice and product offerings are relevant and optimally aligned with each customer’s unique needs.
Automated operations: AI agents efficiently handle routine and repetitive tasks such as document processing, customer inquiries, and data retrieval, reducing the operational costs associated with manual labor. For example, conversational AI agents can handle customer service interactions, significantly improving response times and reducing the workload on human staff.
Reduced errors and false positives The accuracy of AI agents significantly reduces the likelihood of errors commonly associated with human operations, such as data entry and calculations. Additionally, AI agents help minimize false positives in fraud detection.
AI agents bring a transformative edge to the finance industry, addressing operational challenges beyond simple task automation. By enhancing efficiency, reducing costs, improving risk management and customer satisfaction, AI agents streamline current processes and pave the way for new capabilities and innovations within the financial sector.
Building LLM-based AI agents for finance: A step-by-step guide
Large Language Models (LLMs) are transforming the financial industry by automating tasks, enhancing decision-making, and providing personalized financial guidance. With LLM-powered AI agents, financial institutions can optimize investment strategies, improve risk management, and offer tailored financial advice to clients. This section provides a detailed walkthrough of building your own LLM-powered AI agent specifically designed for financial applications.
Define the business objectives
Scope definition: Clearly delineate the specific financial tasks or challenges your AI agent is designed to address. This may include automating high-volume transactions, personalizing financial advice for clients, or optimizing asset management strategies. Specify the particular segment of the finance sector you aim to target—such as personal banking, investment management, or insurance—and outline the principal challenges the AI agent will help overcome.
Task-oriented approach: Identify the precise functionalities your AI agent should perform in the financial environment:
- Client interaction: Automate and personalize client interactions to enhance engagement and increase client satisfaction.
- Transaction analysis: Analysis of transaction data to optimize financial operations and risk assessment.
- Market research and forecasting: Analyze market data, predict market movements, and identify investment opportunities.
- Investment optimization: Improve investment strategies through personalized portfolio recommendations and real-time market adjustments.
- Risk assessment: Utilize predictive models to assess and mitigate financial risks more effectively.
- Financial planning: Provide tailored financial advice based on individual client profiles and market conditions.
By integrating these AI capabilities, financial institutions can achieve higher operational efficiency and client satisfaction.
Select the right LLM model
Choose a base LLM that aligns with your specific financial service needs. Here are some strategic options:
- OpenAI’s GPT family (GPT-3.5, GPT-4): Renowned for their robust text generation, summarization, translation, and creative writing capabilities. GPT-4, in particular, excels in natural language understanding and generation, making it ideal for complex financial queries and client interaction. Access is primarily through an API.
- Google’s PaLM 2 (Pathway Language Model 2): This model boasts strong performance in reasoning, coding, and multilingual tasks, beneficial for global financial platforms that require handling diverse data sets and regulatory environments. Access is typically via Google’s AI platform or services like Vertex AI.
- Meta’s LLaMA (Large Language Model Meta AI): It is available in different sizes, making it adaptable to various financial tasks. LLaMA can be utilized for financial modeling, risk analysis, and market research. Access is often granted through research partnerships or specific releases.
- BLOOM: A powerful open-source LLM ideal for multilingual tasks. Useful for financial institutions with global operations.
- Hugging Face Transformers: This library and community offer access to a broad collection of pre-trained LLMs, facilitating the experimentation and comparison of different models for specialized financial tasks.
Factors to consider:
- Model size: Larger models are generally more capable but require more computational resources.
- Performance: Evaluate the model’s accuracy and efficiency on tasks similar to your needs.
- Licensing: Based on your budget and usage requirements, consider open-source options (e.g., BERT) or commercial APIs (e.g., OpenAI’s GPT-3 API).
Data collection and preparation
- Quality data is crucial: Gather relevant, high-quality datasets specific to the financial domain. This might include:
- Financial market data: Historical and real-time data on assets, bonds, currencies, commodities, and other financial instruments.
- Company data: Financial statements, earnings reports, and other relevant information about publicly listed companies.
- Client data: Financial profiles, investment goals, risk tolerance, and transaction history.
- Regulatory data: Financial regulations, compliance standards, and reporting requirements.
- Data cleaning and preprocessing: Ensure the data is clean, organized, and formatted correctly to train the LLM effectively.
- Data cleaning: Remove irrelevant information, correct errors, and handle missing data.
- Data formatting: Ensure consistent structure and formatting (e.g., using JSON, CSV) to make it compatible with the LLM.
Train the LLM (for the specific financial domain)
- Domain adaptation: Fine-tune the pre-trained LLM on your specific financial data to enhance its understanding of the financial domain and the nuances of your business.
- Prompt engineering: Experiment with different ways of phrasing prompts to elicit the most accurate and relevant responses for financial scenarios. For example, providing context about market conditions, client preferences, or specific investment goals can improve the quality of financial advice.
Develop the AI agent architecture: Building the brain and body
- Modular design: Design the AI agent as a system with distinct modules, each responsible for a specific function:
- Input processing: Handles user queries and commands, ensuring accurate interpretation of client requests.
- LLM interaction: Interacts with the trained LLM to generate responses, insights, and financial recommendations.
- Output generation: Presents the LLM’s output in a user-friendly format, potentially using natural language generation (NLG) to create conversational responses.
- Memory and context: This enables the agent to remember past interactions and maintain context during multi-turn conversations, allowing personalized and tailored advice.
Implement Natural Language Understanding (NLU)
- Query interpretation: Develop NLU modules to interpret customer queries and commands accurately. For example, identify the intent behind a client’s question (e.g., seeking investment advice, requesting risk assessment, inquiring about account balance).
- Intent recognition: Train the agent to understand the user’s intent (e.g., seeking information, comparing investment options, requesting financial planning).
- Entity extraction: Train the agent to identify and extract key entities from text, such as company names, investment products, financial instruments, and dates. This information can be used to provide targeted recommendations and perform specific tasks.
Integrate knowledge bases and external systems
- Knowledge is power: Integrate external knowledge bases and databases to provide the AI agent with a wider range of information to draw upon.
- Financial data providers: Connect with reputable data providers like Bloomberg, Refinitiv, or FactSet to access real-time market data and financial information.
- Regulatory databases: Integrate with regulatory databases to ensure compliance and identify potential violations.
- External data sources: Leverage external data sources for market insights, economic forecasts, and trend analysis.
- Fact-checking: Implement mechanisms to verify information against trusted sources and flag potential inaccuracies or inconsistencies.
- Continuous learning: Design systems for the AI agent to continuously learn and update its knowledge base with new insights and data, including market updates, and regulatory changes.
Incorporate reasoning and analysis capabilities
- Data analysis: Implement algorithms for data analysis, including statistical analysis, pattern recognition, and trend identification. This can be used for market research, client segmentation, and financial forecasting.
- Sentiment analysis: Analyze market news and social media sentiment to identify potential investment opportunities and risks.
- Risk analysis: Develop systems to assess the risk associated with different investment strategies and generate client risk profiles.
- Portfolio optimization: Implement algorithms for portfolio optimization, maximizing returns while mitigating risk based on client preferences.
Design output generation and interaction
- Natural Language Generation (NLG): Develop capabilities to generate human-like responses, financial reports, and investment recommendations.
- Query refinement: Implement features that allow clients to refine their queries and receive more precise results.
- Personalization: Implement techniques to tailor responses based on client profiles, investment goals, and risk tolerance.
- Multilingual support: Create modules for translating and localizing content for global financial markets.
- Visualization: Create modules that can generate charts, tables, and other visualizations to present data in an easily understandable format.
Implement ethical and bias mitigation measures
- Fairness in recommendations: Ensure investment recommendations are not biased towards certain asset classes, industries, or financial products.
- Bias detection: Develop systems to detect and mitigate potential biases in data, algorithms, and outcomes.
- Transparency: Implement measures to explain investment recommendations and pricing decisions, building client trust.
- Data protection: Ensure compliance with data protection regulations like GDPR and CCPA.
Create user interface and interaction design
- Intuitive interface: Create an easy-to-use interface that allows clients to interact with the AI agent naturally. This could include chatbot interfaces, voice assistants, or personalized financial dashboards within a website or app.
- Omnichannel support: Develop interfaces for various channels (e.g., website chatbot, mobile app, voice assistants).
- Query refinement: Implement features for query refinement, allowing users to iteratively refine their search queries and receive more precise results.
Testing and validation
- Rigorous testing: Conduct thorough testing of the AI agent’s capabilities across various financial tasks and scenarios.
- Validation studies: Compare the AI agent’s outputs to human expert analysis to validate its accuracy and reliability.
- Ongoing monitoring: Implement ongoing monitoring and quality control measures to ensure the agent’s performance remains consistent over time.
Deployment and scaling
- Infrastructure: Set up the necessary infrastructure to deploy the AI agent, considering factors like computational resources, storage capacity, and security.
- Data security: Implement robust security measures to protect client’s confidential data.
- Scalability: Develop strategies to scale the AI agent’s capabilities to accommodate increased transaction volumes and users.
Continuous improvement and updating
- Feedback loops: Establish feedback loops to gather user input and continuously improve the AI agent’s performance.
- Regular updates: Regularly update the agent’s knowledge base with the latest financial research findings, market data, and regulatory changes.
- Performance analytics: Implement systems to track KPIs such as investment returns, risk metrics, and client satisfaction scores.
- Version control: Implement version control and change management processes to track updates and ensure stability.
Documentation and training
- Staff training: Implement training programs for finance professionals to use AI agents effectively, covering their functionalities, regulatory compliance, and ethical use.
- Client education: Provide clients with easy-to-understand guides to enhance their interaction with AI-driven financial services.
- Best practices: Develop guidelines to ensure AI-enhanced financial services uphold client service standards, data security, and optimal decision-making.
Platforms for building AI agents
- AutoGen (from Microsoft): A framework specifically designed for building conversational AI agents using LLMs. It simplifies creating agents to engage in multi-turn conversations, access tools, and perform complex tasks.
- CrewAI: A no-code platform for building and deploying AI agents, including those powered by LLMs. It offers a user-friendly interface for defining agent workflows, integrating data sources, and managing agent interactions.
Key considerations
- Human-AI collaboration: Emphasize that AI agents are tools meant to augment, not replace, human expertise in finance. Encourage a collaborative environment where AI supports teams in achieving strategic goals.
- Customer privacy: Make customer data protection a priority and secure the necessary consent for AI interactions.
- Ethical implications: Address the ethical dimensions of using AI in finance, ensuring responsible, transparent usage that positively impacts society.
Building LLM-powered AI agents for finance is a challenging but rewarding journey. By following this guide, you can develop a powerful financial assistant that enhances investment decisions, improves risk management, and provides personalized financial guidance to clients, contributing to the advancement of the financial industry.
Future trends for AI agents in finance
Let’s explore some of the exciting future trends of AI agents in finance:
AI-enabled Customer Experience (CX)
The future of customer experience in finance is set to be heavily influenced by AI. AI agents will provide personalized recommendations and power intelligent chatbots and virtual assistants capable of offering interactive and immersive experiences. These agents will allow financial institutions to engage with customers more meaningfully and efficiently, enhancing customer satisfaction and loyalty through tailored interactions and responsive service.
Automation and robotics in finance
AI’s role in automation extends beyond simple task automation to encompass complex financial operations and decision-making processes. In finance, AI agents are set to transform traditional practices by automating high-stakes functions like risk management, and fraud detection. This expansion in capabilities will increase operational efficiency, reduce human error, and pave the way for more reliable and secure financial operations.
Generative AI in finance content creation
Generative AI is beginning to take center stage in creating new content within the financial sector. AI agents are becoming key players in financial content creation, from generating financial reports to creating complex investment strategies. This trend will transform financial advising, market analysis, and personalized customer communications.
AI-assisted decision making
In finance, decision-making processes are complex and data-driven. AI agents are increasingly integrated into decision support systems to provide deep insights and analytics that inform better decision-making. Whether it’s in asset management, insurance underwriting, or regulatory compliance, AI agents help parse through complex datasets to identify trends and provide actionable insights, aiding more informed and effective decision-making.
Ethical AI and regulatory compliance
As AI evolves, the emphasis on ethical AI and regulatory compliance becomes more crucial. The financial sector, in particular, faces stringent regulatory requirements that AI agents must adhere to. Future developments will focus on creating AI systems that are not only effective but also transparent, accountable, and aligned with ethical standards. This includes ensuring that AI agents handle data responsibly, make unbiased decisions, and operate within the legal frameworks set by financial regulators.
Integration with IoT and blockchain
Integrating AI agents with IoT devices and blockchain technology will become more prevalent in finance. AI agents will manage and analyze data from IoT devices to optimize operations and enhance security. Similarly, blockchain integration will strengthen transparency and security, particularly in transactions and data management, where AI agents can automate and verify operations efficiently and securely.
Advancements in Natural Language Processing (NLP)
As NLP technology evolves, AI agents will become increasingly sophisticated in understanding and generating human-like responses. This will lead to the development of more advanced virtual assistants and chatbots capable of engaging in more complex conversations and performing a broader range of tasks, thus enhancing the quality of customer service in finance.
Increased adoption across industries
AI agents are set to see widespread adoption beyond traditional finance into sectors such as healthcare, retail, and manufacturing. Businesses are recognizing their potential to streamline operations, enhance productivity, and improve customer experiences. This broadened adoption underscores the versatility and effectiveness of AI agents in diverse operational contexts.
Endnote
As we have explored throughout this article, the integration of AI agents into the financial sector represents a significant leap forward in how businesses manage and interpret vast amounts of financial data, interact with customers, and make crucial operational decisions. These intelligent systems, powered by advanced algorithms and capable of learning and adapting, are not just tools but transformative agents that redefine the boundaries of automation and decision-making in finance.
The potential of AI agents in finance is vast—from personalizing customer experiences to enhancing the accuracy of risk assessments and driving operational efficiencies. As technology progresses, the sophistication and capabilities of AI agents will only grow, making their integration into financial services more widespread and impactful. Businesses looking to remain competitive in this rapidly evolving landscape should consider investing in AI development.
In essence, AI agents are not merely a technological enhancement but a transformative element reshaping the financial industry’s future, marking a significant shift towards more agile and intelligent financial services.
Transform your financial services with the power of AI agents today! Discover how LeewayHertz’s AI agent development services can streamline your operations, enhance customer interactions, and boost efficiency.
Start a conversation by filling the form
All information will be kept confidential.