How to build a financial fraud detection system using machine learning?
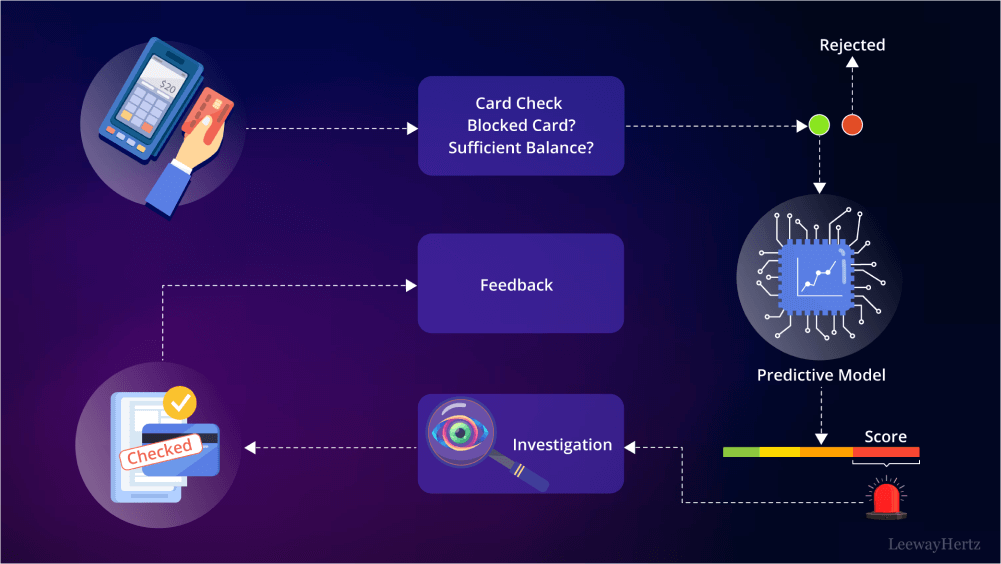
In an era where digital transactions are the norm, the stark rise in online payment fraud, with losses soaring to $41 billion in 2022 and projected to hit $48 billion by 2023, underscores an urgent need for robust countermeasures. The financial repercussions of payment fraud are palpable, but the less quantifiable costs – the erosion of customer trust, reputational damage, and the heightened scrutiny from regulators – can be equally detrimental to businesses. In response to this growing threat, organizations are increasingly harnessing the prowess of machine learning.
As a dynamic subset of Artificial Intelligence (AI), machine learning emerges as a formidable ally in the battle against payment fraud. It adeptly navigates through vast datasets and leverages sophisticated algorithms to find patterns and irregularities synonymous with fraudulent activities. This capacity to pinpoint and preempt fraud in real-time not only fortifies financial transactions but also reinstates a shield of trust and security around them. In navigating the intricate landscape of payment fraud, machine learning stands as a sentinel, safeguarding not just the immediate financial assets but also the enduring trust and reputation of businesses in the digital age.
This comprehensive article navigates through the intricate terrain of financial fraud, offering an in-depth exploration of its multifaceted nature, the vulnerabilities within financial operations, and the limitations of traditional fraud detection mechanisms. We delve into the transformative potential of machine learning in revolutionizing fraud detection, comparing its prowess against conventional statistical methods and shedding light on its varied applications in thwarting diverse fraud scenarios.
Embarking on a journey from the rudiments to the sophisticated nuances of constructing a robust fraud detection system, we dissect each pivotal phase: from meticulous data collection and preprocessing to the strategic selection and training of machine learning models. The discourse extends to a rigorous evaluation and validation of these models, ensuring their precision and reliability.
Deployment strategies are scrutinized, ensuring the seamless integration of machine learning models within existing financial frameworks, followed by crucial post-deployment considerations, including continuous monitoring and iterative model enhancement. Key insights are imparted on optimizing machine learning applications in fraud detection, ensuring that readers are equipped with the knowledge to navigate the complexities of this domain.
As we cast an eye towards the horizon, we also encapsulate the emerging trends and innovations shaping the future of fraud detection in finance. This article is not just a guide but a comprehensive resource for professionals seeking to fortify their financial operations against the ever-evolving threat of fraud through the power of machine learning.
- Types of fraud in finance
- Financial functions and operations susceptible to fraud with examples
- Limitations of traditional fraud detection systems
- Machine learning and its advantages in detecting fraud
- Overview of machine learning vs traditional statistical methods
- Use cases of machine learning in prominent fraud scenarios in finance
- How to build a financial fraud detection system using machine learning models?
- Data collection and preprocessing – sources, techniques and feature engineering
- Choosing the right machine learning model – types of machine learning models
- Training the fraud detection model – steps, techniques and methods
- Model evaluation and validation – Criteria and techniques
- Deployment of the model – strategies
- Post-deployment considerations – monitoring, retraining and updating
- Key considerations when using ML for fraud detection
- Future trends and innovations of fraud detection in finance
Types of fraud in finance
Fraud in finance is a deliberate act of deception or misrepresentation intended to result in financial or personal gain, often at the expense of others. It involves manipulating, concealing, or falsifying essential information to trick individuals, businesses, or institutions. Financial fraud is not limited by scale or scope; it can range from small-scale acts committed by individual perpetrators, like credit card fraud, to highly complex schemes orchestrated by organizations, such as securities fraud or corporate embezzlement.
Financial fraud manifests in a multitude of forms, each characterized by unique tactics and requiring specialized strategies for identification and mitigation. Below is a detailed exploration of the prevalent types of financial fraud, underscoring the intricacies of each and highlighting the importance of tailored approaches to effectively combat these fraudulent activities:
Identity theft: This form of fraud involves the unauthorized acquisition of personal data, such as names, social security numbers, and credit card details. Perpetrators use this stolen information for various illicit activities, including opening fraudulent accounts, obtaining loans, or making unauthorized transactions, all without the victim’s consent. The repercussions for victims are severe, often including financial loss, credit score damage, and a lengthy recovery process.
Credit card fraud: This occurs when individuals illicitly obtain and use another person’s credit card information for unauthorized purchases or cash withdrawals. Techniques such as phishing (tricking individuals into revealing personal information), skimming (copying card information using a concealed device), and exploiting data breaches are common methods used by fraudsters.
Investment fraud: These schemes involve deceiving investors with the promise of high returns with little to no risk. Examples include:
- Ponzi schemes: Where returns for older investors are paid with the capital of newer investors, rather than from profit earned.
- Pyramid schemes: Where participants earn money primarily by recruiting more participants into the scheme.
- Pump-and-dump schemes: Involving artificially inflating the price of owned stock through false and misleading positive statements, then selling the cheaply purchased stock at a higher price.
- Advance fee fraud: Asking for money upfront with the promise of a significant return later.
Corporate fraud: This encompasses unethical and illegal activities conducted within or against a corporation. Common types include:
- Embezzlement: Misappropriation of funds placed in one’s trust or belonging to one’s employer.
- Insider trading: Trading a public company’s stock based on material, non-public information.
- Falsifying financial statements: Manipulating official records to present a more favorable picture of the company’s financial health, profitability, or performance.
- Bribery: Offering, giving, receiving, or soliciting something of value as a means of influencing the actions of an individual holding a public or legal duty.
Mortgage fraud: This involves misstating, misrepresenting, or omitting relevant details about income, liabilities, property, or other elements of a mortgage transaction to obtain or secure a loan. Variants include income fraud (overstating income to secure a larger loan), appraisal fraud (manipulating appraisal reports to misstate a property’s value), and occupancy fraud (lying about the intended use of the property).
Bank fraud: This broad category involves using illegal means to obtain assets, funds, or other property owned or held by a financial institution. Methods include check kiting (exploiting the time required for checks to clear to create fictitious balances) and deposit account fraud (opening accounts with fake information).
Insurance fraud: This type of fraud can be committed by applicants, policyholders, third-party claimants, or professionals who provide services to claimants. Insurance fraud may entail fabricating claims, exaggerating legitimate claims, or staging accidents to collect benefits from insurance policies.
Money laundering: A complex form of financial fraud that involves disguising the origins of illegally obtained money, typically by means of transfers involving foreign banks or legitimate businesses. It’s often associated with organized crime and is executed in stages: placement (introducing illicit funds into the financial system), layering (concealing the source of funds through complex transactions), and integration (retrieving the money from the legitimate economy).
Embezzlement: This involves an individual, often someone in a position of trust or responsibility, misappropriating funds or assets to which they have access. This form of fraud is insidious as it not only involves the theft of funds but also a breach of trust.
Counterfeit checks: The creation and use of fake checks to unlawfully withdraw funds from the victim’s bank account. This type of fraud can be particularly damaging if large sums are withdrawn or if the fraud is part of a broader scheme of identity theft.
Tax evasion: The illegal evasion of taxes by individuals, corporations, and trusts. Tax evasion often involves dishonest tax reporting, such as declaring less income, profits, or gains than the amounts actually earned or overstating deductions.
Wire fraud: A form of fraud that involves the use of electronic communications or an interstate communications facility to defraud individuals or entities of money or property. Common examples include phishing emails, advanced-fee scams, and business email compromise schemes.
Understanding the multifaceted nature of financial fraud is crucial for developing robust detection and prevention systems. Each category requires a nuanced approach, integrating sophisticated technology, regulatory knowledge, and a proactive stance to effectively safeguard against these deceptive practices. As the financial landscape continues to evolve, so too must the strategies and tools employed to combat fraud, ensuring trust and integrity remain at the core of financial interactions.
Strengthen Financial Integrity With Our ML Solutions
Bolster your financial defenses against fraud using machine learning. Dive into our Machine Learning Development Services to implement robust solutions tailored to your security needs.
Financial functions and operations susceptible to fraud with examples
Financial functions and operations are integral to the workings of any organization, yet they are also areas where fraud can and does occur. Understanding these vulnerabilities is key to creating effective safeguards. Below is a detailed exploration of various financial functions and operations that are susceptible to fraud, along with examples for each:
Accounts payable and receivable
- Fraud type: Invoice fraud, double billing, or fictitious vendors.
- Example: An employee might set up a fake company and submit invoices for non-existent goods or services. Alternatively, they might collude with an external vendor to submit inflated invoices, sharing the excess payments.
Payroll
- Fraud type: Ghost employees, unauthorized bonuses, or inflated expense claims.
- Example: A payroll manager might add a non-existent employee to the payroll (a “ghost employee”) and divert these payments to their account. Or, employees might claim expenses for travel or meals that never occurred.
Procurement and purchasing
- Fraud type: Kickbacks, bid rigging, or conflict of interest.
- Example: An employee in the procurement department might accept bribes from a vendor in exchange for awarding them a contract, even if they aren’t the best or most cost-effective option (kickbacks).
Asset misappropriation
- Fraud type: Theft of company assets, inventory shrinkage, or misuse of company resources.
- Example: An employee might steal office supplies or products from company inventory for personal use or resale.
Financial statement fraud
- Fraud type: Earnings management, improper revenue recognition, or hidden liabilities.
- Example: A company might artificially inflate its sales revenue by recognizing revenue prematurely or by keeping certain liabilities off the balance sheet to make the company appear more financially stable than it is.
Banking and wire transfers
- Fraud type: Unauthorized transfers, account takeover, or payment diversion.
- Example: A fraudster might use phishing tactics to gain access to a company’s banking credentials and make unauthorized wire transfers.
Tax compliance and reporting
- Fraud Type: Tax evasion, false deductions, or underreporting income.
- Example: A company might underreport its income or exaggerate its expenses to reduce its tax liability.
Loan and credit functions
- Fraud type: Application fraud, identity theft, or collateral fraud.
- Example: An individual might use stolen identity information to apply for a loan or credit card, or they might inflate the value of assets used as collateral for a loan.
Insurance functions
- Fraud type: False claims, policyholder fraud, or underwriter fraud.
- Example: An individual might exaggerate the extent of damage in an insurance claim to receive a higher payout or might stage an event, such as a car accident, to claim insurance money.
Fraud in these areas can have devastating effects, not just financially but also on an organization’s reputation and trustworthiness. It’s crucial for companies to implement strong internal controls, regular audits, and robust fraud detection systems to mitigate these risks. This includes leveraging advanced technologies such as AI and machine learning for anomaly detection, ensuring compliance with legal and regulatory standards, and fostering an organizational culture of ethics and transparency.
Limitations of traditional fraud detection systems
Traditional fraud detection systems have been foundational in identifying and preventing fraudulent activities. However, as fraudsters’ methods become more sophisticated and technology evolves, these systems face several limitations. Understanding these limitations is crucial for developing more effective fraud prevention strategies. Here’s an in-depth look at the challenges and limitations of traditional fraud detection systems:
Rule-based systems
- Traditional systems often rely on predefined rules or heuristics to identify fraudulent activities. While rules are essential for catching known fraud patterns, they are less effective against new or evolving schemes.
- Rule-based systems can generate a high volume of false positives, leading to unnecessary investigations and resource allocation, potentially overlooking genuine cases of fraud in the process.
Reactivity rather than proactivity
- Traditional systems are typically reactive, designed to identify and respond to fraud after it has occurred. This approach can result in significant losses before the fraud is detected and stopped.
- The lack of predictive capabilities means that traditional systems are not equipped to anticipate or adapt to new types of fraud quickly.
Scalability and adaptability Issues
- As transaction volumes and the variety of transaction types increase, traditional systems can struggle to scale effectively, potentially leading to system overloads or missed fraud attempts.
- Adapting these systems to new types of fraud, changing consumer behavior, or emerging technologies often requires extensive manual effort and system reconfiguration.
Data silos and integration challenges
- Traditional systems may not be well-integrated with other data systems within an organization, leading to fragmented data and an incomplete view of customer activities.
- The inability to consolidate and analyze data across different channels and systems can result in missed connections and overlooked patterns indicative of fraud.
Limited learning and evolution
- Traditional systems do not learn from new data. Updating these systems to understand new fraud patterns requires manual intervention, making the process slow and resource-intensive.
- The lack of continuous learning means that as fraudsters evolve their tactics, traditional systems become increasingly ineffective.
User experience trade-offs
- The need to balance fraud detection with user experience can be challenging. Stringent rules can lead to legitimate transactions being declined (false positives), frustrating customers and potentially driving them away.
- Conversely, too lenient rules can increase the risk of fraud, leading to financial losses and reputational damage.
Complexity and cost of maintenance
- Maintaining and updating rule-based systems, especially in large organizations with high transaction volumes, can be complex and costly.
- The need for specialized staff to manage and update these systems adds to the operational overhead.
Lack of contextual and behavioral understanding
- Traditional systems may not effectively consider the context of transactions or user behavior, leading to an inability to distinguish between legitimate anomalies and actual fraud.
- The lack of behavioral analysis means these systems might miss subtle cues that could indicate fraudulent activity.
In response to these limitations, many organizations are turning to advanced technologies, such as machine learning and AI, to complement or replace traditional systems. These advanced systems can learn from new data, identify complex patterns, and adapt to evolving fraud tactics, offering a more dynamic and effective approach to fraud detection and prevention. However, the transition requires careful planning, investment, and a strategic approach to data management and analysis.
Strengthen Financial Integrity with ML Solutions
Bolster your financial defenses against fraud using machine learning. Dive into our Machine Learning Development Services to implement robust solutions tailored to your security needs.
Machine learning and its advantages in detecting fraud
Leveraging Machine Learning (ML) for fraud detection presents a transformative approach for businesses seeking to fortify their defenses against financial deceit. The integration of ML in fraud detection mechanisms offers numerous advantages, reshaping the landscape of financial security with its inherent capabilities:
Cost-effectiveness and efficiency
- Machine learning significantly reduces the financial footprint of fraud detection by automating processes that traditionally required extensive manual oversight. This automation translates to decreased expenditures on labor, technology, and operational time, allowing for a more efficient allocation of resources and a reduction in overall fraud management costs.
- The scale and speed of ML-based systems enable the processing of vast volumes of transactions, delivering accurate responses in real-time, often within milliseconds. This instantaneous processing capability not only enhances transaction security but also improves customer experience, a crucial aspect in today’s fast-paced financial environment.
Enhanced accuracy and pattern recognition
- Unlike traditional methods that rely heavily on human analysis, ML algorithms thrive on vast datasets, extracting meaningful patterns and identifying anomalies with a level of precision unattainable by human operators. This advanced pattern recognition significantly diminishes the rate of false positives and negatives, key metrics in the evaluation of fraud detection accuracy.
- The robust analytical prowess of ML models ensures that even the most subtle and sophisticated fraudulent activities do not go unnoticed, safeguarding businesses against evolving threats.
Relentless and adaptive fraud monitoring
- Machine learning models operate tirelessly, offering 24/7 monitoring without the constraints of human fatigue or the need for breaks. This continuous vigilance ensures that data is analyzed in real time, providing an unbreakable shield against fraudulent activities.
- As dynamic systems, ML models continuously evolve by learning from new data. This perpetual learning mechanism allows them to swiftly adapt to emerging fraud tactics, ensuring that businesses remain at the forefront of fraud prevention.
Reduced operational costs
- The automation inherent in ML-driven fraud detection systems minimizes the necessity for manual intervention, leading to a significant reduction in operational costs. With the continuous refinement of ML models through exposure to increasing data volumes and varied scenarios, the precision and reliability of these systems progressively improve, further enhancing their cost-effectiveness.
Continuous and proactive fraud detection
- ML systems embody a proactive approach to fraud detection. Their capacity to incessantly learn and adapt enables them to identify new fraud patterns and trends promptly and accurately. This agility ensures that organizations are not merely reacting to fraudulent activities but are equipped to anticipate and neutralize threats before they manifest, maintaining a state of perpetual preparedness.
In essence, the deployment of machine learning in fraud detection heralds a new era in financial security, characterized by heightened accuracy, relentless analysis, and proactive threat identification. This paradigm shift not only empowers businesses to protect their assets and reputation more effectively but also fosters an environment of trust and safety, crucial for sustainable growth and customer satisfaction.
Overview of machine learning vs traditional statistical methods
Here’s a detailed comparison table that outlines the key differences between machine learning and traditional statistical methods:
Feature | Machine Learning | Traditional Statistical Methods |
Approach to Data | Often works well with large and complex datasets. | Best suited for smaller, structured datasets with fewer variables. |
Model Complexity | Can handle and thrive on more complex, non-linear relationships. | Focuses on simpler, often linear relationships. |
Assumptions | Makes fewer assumptions about the structure of data. | Makes more strict assumptions about data structure (e.g., normality, linearity). |
Predictive vs. Inferential | Primarily predictive; focuses on making accurate predictions. | Primarily inferential; focuses on understanding relationships between variables. |
Feature Selection | Automated feature selection and can handle irrelevant features. | Requires careful feature selection and preprocessing. |
Outcome Focus | Outcome-oriented; cares more about the performance of the model. | Focuses on the significance of variables and the model. |
Transparency and Interpretability | Often seen as a ‘black box’ due to complex algorithms. | More transparent and interpretable due to simpler models and clear statistical significance. |
Adaptability and Evolution | Continuously improves as more data is fed into the system. | Static; does not improve or adapt after the model is built. |
Handling of Nonlinearity and Interactions | Excellently handles non-linear relationships and interactions between variables. | Struggles with non-linearity and complex interactions unless specifically modeled. |
Computation Time and Power | Requires more computational resources and time, especially with large datasets. | Generally less computationally intensive. |
Strengthen Financial Integrity with ML Solutions
Bolster your financial defenses against fraud using machine learning. Dive into our Machine Learning Development Services to implement robust solutions tailored to your security needs.
This table provides an overview of the contrasting characteristics of machine learning and traditional statistical methods. The choice between the two often depends on the specific requirements of the task, the nature and size of the dataset, and the ultimate goal of the analysis.
Use cases of machine learning in prominent fraud scenarios in finance
Predictive analytics in fraud detection
Machine learning algorithms are pivotal in the fight against financial fraud, utilizing historical transaction data to unearth trends and patterns indicative of fraudulent activities. The essence of predictive analytics lies in its capacity to analyze past data, identifying correlations and behaviors linked to fraud.
This analytical approach involves extracting relevant information from historical transactions to train sophisticated models. These models are designed to adapt and evolve, continuously refining their ability to foresee and mitigate potential fraud risks. Through predictive analytics, financial institutions are equipped to proactively implement security measures, drawing on insights from historical data. This not only helps in preempting future fraudulent schemes but also plays a crucial role in the real-time identification of ongoing fraudulent activities, thereby safeguarding the assets and trust of financial institutions and their customers.
Transaction monitoring in fraud detection
Transaction monitoring involves the systematic, real-time scrutiny of financial activities as they transpire. This continuous surveillance encompasses a wide array of financial operations, including but not limited to credit card transactions, fund transfers, and deposits.
Information for this comprehensive monitoring is sourced from various channels such as banks, credit card issuers, online payment platforms, and other financial entities, which collectively amass detailed transaction data. Machine learning algorithms then process this data promptly and meticulously.
In the realm of transaction monitoring, these ML algorithms excel in discerning patterns and trends within extensive datasets. They establish normative behavioral profiles for each account or business entity, encapsulating details like usual transaction frequencies, typical transaction amounts, favored locations for transactions, and common transaction timings.
When a transaction markedly strays from these established norms, or a series of transactions occur in rapid succession, the system flags it as an anomaly. For instance, an alert is triggered if an account, generally characterized by small, sporadic transactions, suddenly executes a substantial and atypical transaction.
Moreover, transaction monitoring systems extend their vigilance to the network of account relationships. Activities such as transferring funds to previously unknown accounts or a sudden increase in transfers between accounts may signal fraudulent intentions. By keeping a vigilant eye on these aspects, transaction monitoring plays an integral role in detecting and averting financial fraud, thereby safeguarding the interests of financial institutions and their clientele.
Anomaly detection in fraud detection
Machine learning algorithms are adept at learning to recognize deviations in transactional and operational patterns. When a transaction significantly diverges from a customer’s typical activity or established norms, the system triggers an alert. For instance, an unusually large withdrawal from an account with a history of modest transactions might activate an anomaly warning.
Beyond transaction monitoring, anomaly detection also finds significant application in financial auditing, where maintaining the integrity of financial records is paramount. A study exploring the challenges faced by financial auditors in identifying discrepancies highlighted the vital role of accurate financial data for business operations.
The research employed two unsupervised machine learning methods, namely isolation forest and autoencoders, alongside seven supervised techniques, including deep learning. These models were trained and tested on an authentic general ledger dataset, employing data vectorization to manage the variability in journal entry sizes.
Results from the study underscored the substantial potential of both supervised and unsupervised machine learning models in pinpointing higher-risk journal entries and recognizing specific anomaly categories. By facilitating effective data sampling, these models offer promising avenues to elevate the efficiency of financial audits. This advancement in machine learning presents a significant opportunity to bolster the reliability and effectiveness of financial audits, contributing to more secure and trustworthy financial operations.
Voice recognition in fraud detection
Voice biometrics stands at the forefront of AI-powered speech recognition technology, offering a secure method to verify an individual’s identity through their unique vocal imprint. This sophisticated technology delves into various vocal characteristics, including enunciation, pitch, intonation, accent, and speech patterns, to perform user verification and authentication.
The financial industry, with its ever-increasing emphasis on security, is recognizing the immense potential of voice biometrics. This recognition is driving the growth and adoption of voice recognition technologies, positioning them as key players in the ongoing enhancement of fraud detection and prevention strategies in finance.
Identification verification in fraud detection
Machine learning technologies are revolutionizing identification verification in the finance sector by facilitating user authentication. These technologies compare the information provided by users during account creation with external database records. Financial institutions are increasingly adopting biometric identification techniques, including voice, facial, and fingerprint recognition. Machine learning models meticulously analyze these biometric inputs to confirm user identity, thereby merging heightened security with user convenience.
In addition, during account setup, customers can submit identity documents such as passports, ID cards, and driver’s licenses. Machine learning algorithms then autonomously validate these documents, cross-referencing them against established templates. They employ sophisticated image recognition algorithms to detect any discrepancies, modifications, or fraudulent characteristics, further bolstering the integrity of the identification verification process.
Geolocation tracking in fraud detection
Geolocation tracking, powered by machine learning, plays a crucial role in enhancing the security of financial transactions. This technology continuously records the geographical locations of transactions and compares them against a customer’s historical transaction data. By analyzing patterns and identifying transactions occurring in atypical locations, machine learning algorithms can swiftly pinpoint potential irregularities that may suggest fraudulent activity.
The real-time analysis capability of geolocation tracking allows financial institutions to proactively monitor and protect customer accounts. For instance, if a transaction is made in a location that is significantly distant from a customer’s usual transaction sites or in a region known for high fraud rates, the system can immediately flag it as suspicious. Financial institutions can then take swift action, such as temporarily freezing the account or contacting the customer to verify the transaction’s legitimacy.
Moreover, geolocation tracking can be integrated with other fraud detection mechanisms, such as transaction monitoring and anomaly detection, to form a comprehensive, multi-layered defense system. This integration enhances the accuracy of fraud detection by providing a more holistic view of a customer’s transaction behavior and significantly reducing the likelihood of false positives. As a result, financial institutions can offer a safer transaction environment, fostering trust and confidence among their customers.
Data enrichment in fraud detection
Data enrichment, bolstered by machine learning, significantly enhances the detection of fraudulent activities by integrating additional data sources, such as public records or social media profiles, into the analysis framework. The aim is to augment the existing datasets with these external insights, thereby achieving a deeper understanding of client behavior and characteristics.
Social media profiles, for instance, are rich repositories of information regarding an individual’s connections, interests, and activities. Machine learning models can sift through this data, identifying inconsistencies or patterns that may signal fraudulent behavior. For example, discrepancies between a client’s claimed employment status and their social media activity might trigger a closer examination.
Similarly, public records offer a wealth of pertinent information. Details from court cases, bankruptcy filings, or criminal history can be invaluable in constructing a comprehensive profile of an individual. Machine learning algorithms meticulously cross-reference this information with existing consumer data, pinpointing any irregularities or signs of fraudulent behavior.
By intelligently integrating and analyzing this enriched data, financial institutions can achieve a more nuanced risk assessment, enhancing their ability to proactively identify and mitigate potential fraud. This comprehensive approach not only protects the institutions but also preserves the integrity of the financial system and the trust of its participants.
Strengthen Financial Integrity with ML Solutions
Bolster your financial defenses against fraud using machine learning. Dive into our Machine Learning Development Services to implement robust solutions tailored to your security needs.
How to build a financial fraud detection system using machine learning models?
Data collection and preprocessing – sources, techniques and feature engineering
Data collection and preprocessing are crucial initial steps in the application of machine learning (ML) for fraud detection in finance. These steps lay the foundation for the effectiveness of subsequent analysis and the accuracy of the predictions.
- Data collection: The data collection phase involves gathering a comprehensive set of data that reflects a wide array of financial transactions and user behaviors. Sources for this data can vary widely and might include transaction logs, user account information, payment histories, customer service records, and even data from external sources like credit bureaus or public records. In the context of fraud detection, it’s imperative to collect not just the transactions themselves but also metadata that might capture the context of the transactions, such as time stamps, device information, and location data. High-quality data is instrumental in training robust ML models; hence, ensuring data is relevant, accurate, and up-to-date is paramount.
- Preprocessing: Once the data is collected, the preprocessing phase begins. This phase involves cleaning and transforming raw data into a format that can be effectively used to train ML models. Key steps in this phase include:
- Data cleaning: Identifying and correcting errors or inconsistencies in the data, such as missing values, duplicate records, or outliers. This step is crucial as inaccurate data can lead to misleading patterns and predictions.
- Normalization/Standardization: Transforming numeric columns to a common scale without distorting differences in the ranges of values. This is important because ML algorithms might perform poorly if individual features do not more or less look like standard normally distributed data (e.g., Gaussian with 0 mean and unit variance).
- Encoding categorical data: Converting categorical variables into a form that can be provided to ML algorithms to do a better job in prediction. Techniques such as one-hot encoding or label encoding are commonly used.
- Feature engineering: Feature engineering is perhaps the most important aspect in the application of ML for fraud detection. This step involves creating additional relevant features from the existing data to improve the performance of the ML model. It requires domain knowledge to create features that capture the underlying patterns in the data that might signify fraudulent behavior. For example, in transaction data, rather than just looking at individual transactions, one might look at features like the frequency of transactions in a certain time period, the average transaction amount, or the variation in transaction amounts from the usual pattern. Advanced techniques can also be used to create features based on the relationships between different entities, like the similarity of a user’s behavior to known patterns of fraudulent behavior. Feature engineering can significantly boost the predictive power of ML models, making it a critical step in the ML pipeline for fraud detection.
In summary, data collection and preprocessing form the bedrock of any ML-based fraud detection system in finance. Collecting comprehensive and relevant data, ensuring its cleanliness and usability through preprocessing, and ingeniously engineering features to highlight potential fraudulent patterns are steps that, when executed meticulously, can greatly amplify the ability of ML models to identify and prevent fraudulent activities.
Choosing the right machine learning model – types of machine learning models
In the realm of finance, selecting an appropriate Machine Learning (ML) model for fraud detection is pivotal, as each model offers specific strengths and caters to different aspects of fraud identification and prevention. Here’s a more finance-focused analysis of the four key machine learning models used in fraud detection:
Supervised learning
- Overview: Supervised learning models are particularly suited to financial datasets that are richly labeled and well-documented. These models learn from transactions historically tagged as ‘fraudulent’ or ‘legitimate.’
- Finance applications: Ideal for credit card fraud detection, loan default prediction, and other scenarios where patterns of fraud closely follow historical trends.
- Limitations: Their dependency on labeled historical data may limit their ability to detect novel or evolving fraudulent schemes not present in the training dataset.
Unsupervised learning
- Overview: In environments where labeling is scarce or fraud patterns are not clearly defined, unsupervised learning models excel by identifying hidden structures or anomalies in transaction data.
- Finance applications: Useful in detecting unusual transaction patterns, money laundering activities, or new, sophisticated fraud strategies that deviate from known patterns.
- Limitations: While adept at uncovering new types of fraud, these models may yield higher false positives due to the absence of labeled guidance.
Semi-supervised learning
- Overview: This model leverages both labeled and unlabeled data, making it a versatile choice in financial contexts where comprehensive labeling is impractical or too costly.
- Finance applications: Beneficial for enhancing the detection capabilities of models by incorporating a broader spectrum of transaction data, which may not be fully labeled but still contains valuable patterns.
- Limitations: The model’s performance hinges on the quality and representativeness of the labeled data used in training.
Reinforcement learning
- Overview: Reinforcement learning’s dynamic nature allows it to adapt and optimize decision-making processes based on feedback, making it a powerful tool for evolving financial fraud detection strategies.
- Finance applications: Applicable in scenarios requiring adaptive decision-making, such as real-time transaction approval or dynamic risk assessment, where the system must learn from the outcomes of its previous actions.
- Limitations: Implementing reinforcement learning in finance can be challenging due to the complexity of defining reward systems and the requirement of a simulated environment for training.
In the financial sector, the effectiveness of a machine learning model for fraud detection is largely determined by the specific nature of the transactions, the patterns of fraud involved, and the availability of qualitative data. A nuanced understanding of each model’s capabilities and constraints, combined with a strategic blend of different models, can lead to a highly effective and responsive fraud detection system. This system not only safeguards assets but also adapts to new threats, ensuring financial integrity in an ever-evolving landscape.
Strengthen Financial Integrity with ML Solutions
Bolster your financial defenses against fraud using machine learning. Dive into our Machine Learning Development Services to implement robust solutions tailored to your security needs.
Training the fraud detection model – steps, techniques and methods
Model training and parameter tuning
- Training: Train the model on the training set while monitoring its performance on the validation set. This process involves feeding the model with the input features and the corresponding labels, allowing it to learn the patterns associated with fraudulent and legitimate transactions.
- Parameter tuning: Use techniques like grid search or random search to find the optimal set of parameters for the model. Cross-validation can be used during this process to ensure that the model performs well across different subsets of the data.
Handling imbalanced data
- Challenge: Financial fraud datasets are typically imbalanced, with the number of fraudulent transactions being much lower than legitimate ones. This imbalance can bias the model towards predicting the majority class.
- Strategies: Employ techniques like resampling (oversampling the minority class or undersampling the majority class), using anomaly detection algorithms, or applying advanced methods like SMOTE to synthetically generate new samples in the minority class.
Training a machine learning model for fraud detection is a complex but rewarding task. It requires a careful approach to data preparation, feature engineering, model selection, and evaluation. By paying close attention to each step of the process and employing the right techniques and methods, it’s possible to develop a robust, accurate, and interpretable model that effectively identifies and prevents fraudulent transactions in the financial sector.
Model evaluation and validation – Criteria and techniques
Model evaluation and validation are critical stages in the development of a machine learning model for fraud detection in finance. These steps ensure that the model not only performs well on the training data but also generalizes well to new, unseen data, thereby providing reliable and accurate predictions in real-world scenarios. Here’s a detailed overview of the criteria and techniques used for evaluating and validating machine learning models in the context of financial fraud detection:
Evaluation metrics
- Accuracy: Measures the proportion of correct predictions (both true positives and true negatives) among the total number of cases examined. While it’s a straightforward metric, accuracy may not be the best indicator in imbalanced datasets where fraudulent transactions are much rarer than legitimate ones.
- Precision (Positive Predictive Value): Measures the proportion of true positive predictions among all positive predictions. High precision indicates a low false positive rate but doesn’t consider false negatives.
- Recall (Sensitivity or True Positive Rate): Measures the proportion of actual positives correctly identified. In fraud detection, a high recall is crucial as it reflects the model’s ability to catch fraudulent transactions.
- F1 Score: The harmonic mean of precision and recall, providing a balance between the two. An ideal fraud detection model would have a high F1 score, indicating both high precision (not many legitimate transactions are flagged as fraudulent) and high recall (most fraudulent transactions are caught).
- ROC-AUC Score (Area Under the Receiver Operating Characteristic Curve): This metric measures the model’s ability to distinguish between classes. A higher AUC indicates a better-performing model. The ROC curve is plotted with True Positive Rate against the False Positive Rate, showing the trade-off between sensitivity and specificity.
Cross-validation
- Technique: Involves dividing the dataset into k-subsets (or ‘folds’), training the model on k-1 folds, and validating it on the remaining fold. This process is repeated k times, with each fold being used once as the validation set. The results are then averaged to produce a single estimation.
- Purpose: Helps in assessing how the model’s results will generalize to an independent dataset. It’s particularly useful in scenarios like fraud detection, where the dataset might be imbalanced or the cost of a wrong prediction is high.
Confusion matrix
- Components: A confusion matrix displays the number of true positives, false positives, true negatives, and false negatives. It provides a clear picture of the model’s performance, especially in binary classification tasks like fraud detection.
- Insights: Enables the examination of the model’s ability to correctly classify fraudulent and legitimate transactions, helping in understanding the trade-offs between different types of errors (e.g., misclassifying a legitimate transaction as fraud versus missing a fraudulent transaction).
Handling imbalanced data
- Challenge: Financial fraud datasets are typically imbalanced, which can skew the model’s performance metrics.
- Strategies: Techniques like precision-recall curves are more informative than ROC curves in such scenarios. Additionally, using evaluation metrics that focus more on the minority class (like F1 Score or Matthews Correlation Coefficient) can provide a better understanding of the model’s performance.
Model calibration
- Concept: Model calibration refers to the relationship between the predicted probabilities and the observed probabilities. In fraud detection, a well-calibrated model ensures that the predicted probabilities of fraud are reflective of the true risk.
- Techniques: Calibration plots or reliability diagrams can be used to assess calibration. Models can be calibrated post-training using methods like Platt scaling or isotonic regression.
Threshold tuning
- Importance: The decision threshold determines the point at which a transaction is classified as fraudulent. Adjusting this threshold is crucial in managing the trade-off between precision and recall.
- Technique: Use validation data to identify the threshold that aligns with the business’s risk tolerance and operational requirements. Tools like precision-recall curves can help in visualizing this trade-off and selecting an optimal threshold.
In conclusion, model evaluation and validation are indispensable in ensuring that a machine learning model for fraud detection performs effectively and consistently. By utilizing a combination of the right metrics, validation techniques, and a thorough understanding of the business context, practitioners can develop, assess, and deploy models that significantly bolster the fraud prevention mechanisms in the finance sector.
Deployment of the model – strategies
Deploying a machine learning model for fraud detection in finance is a critical step that requires strategic planning and meticulous execution to ensure the model operates effectively in a real-world environment. Here are the key strategies to consider when deploying such models:
Model integration
- Seamless integration: Ensure the model integrates seamlessly with existing banking or financial systems. This involves aligning the model’s input and output format with the systems in place and ensuring compatibility with the current IT infrastructure.
- APIs and microservices: Use APIs or microservices architecture for model deployment to facilitate easy integration and scalability. This approach allows different components of the financial system to communicate efficiently with the fraud detection model.
Real-time processing
- Latency considerations: Financial transactions often require real-time or near-real-time processing. Ensure the model can handle the required transaction throughput with minimal latency to not impede the transaction flow.
- Streaming data: Implement mechanisms to handle streaming data, allowing the model to make predictions on transactions as they occur. Technologies like Apache Kafka or Amazon Kinesis can be used to manage high-throughput, real-time data streams.
Continuous monitoring and model updating
- Performance monitoring: Continuously monitor the model’s performance to detect any degradation over time. Set up alerts for significant changes in key performance metrics (like precision, recall, or F1-score).
- Regular updates: Fraud patterns evolve, and the model must adapt to these changes. Regularly retrain the model with the latest data and update it to reflect the current fraud landscape.
Fail-safe mechanisms
- Redundancy: Implement redundancy in the deployment architecture to ensure the model remains operational even if one instance fails. This is crucial to maintain uninterrupted fraud detection capabilities.
- Fallback strategies: Develop fallback strategies for scenarios where the model might be uncertain. This could involve flagging transactions for manual review or implementing secondary checks.
Scalability and resource management
- Scalability: Ensure the deployment infrastructure can scale to handle peak loads, especially during high-transaction periods. Cloud services can offer scalable solutions that adjust resources based on demand.
- Resource optimization: Monitor resource utilization and optimize model performance to balance computational costs with detection accuracy. Techniques like model quantization or pruning can reduce resource requirements without significantly impacting performance.
Compliance and data security
- Regulatory compliance: Adhere to all relevant financial regulations and data protection laws, such as GDPR, CCPA, or PCI DSS. This involves ensuring data privacy, secure data handling, and transparent model operations.
- Data encryption: Implement robust encryption protocols for data at rest and in transit to protect sensitive financial information and maintain customer trust.
User feedback loop
- Feedback mechanism: Establish a feedback loop where fraud analysts can provide input on the model’s predictions. This feedback can be used to refine the model and improve its accuracy over time.
- User education: Educate end-users and stakeholders about the model’s capabilities and limitations, ensuring they understand how to interpret and act on the model’s predictions effectively.
Deploying a machine learning model for fraud detection in finance is not just about the technical implementation but also involves strategic considerations regarding integration, monitoring, compliance, and continuous improvement. A well-planned deployment strategy ensures that the model not only performs efficiently but also aligns with the broader operational, regulatory, and security frameworks of the financial institution.
Post-deployment considerations – monitoring, retraining and updating
Post-deployment is a critical phase in the lifecycle of a machine learning model used for fraud detection in finance. It involves diligent monitoring, periodic retraining, and timely updating of the model to ensure its continued effectiveness and relevance. Here’s a detailed look at the key post-deployment considerations:
Continuous monitoring and performance evaluation
- Real-time monitoring: Establish real-time monitoring systems to track the model’s performance continuously. Monitor key metrics such as precision, recall, F1-score, and the number of false positives and false negatives.
- Anomaly detection: Set up anomaly detection mechanisms to alert you to sudden changes in the model’s performance or unusual patterns in the predictions, which could indicate emerging fraud tactics or changes in transaction behavior.
Model retraining and updating
- Periodic retraining: Schedule regular retraining of the model with the latest data. This is crucial because fraud patterns can evolve rapidly, and a model trained on outdated data may lose its effectiveness.
- Data drift and concept drift handling: Be vigilant about data drift (changes in the input data distribution) and concept drift (changes in the statistical properties of the target variable). Implement strategies to detect these drifts and retrain the model accordingly to maintain its predictive power.
Feedback loop and model refinement
- Incorporate analyst feedback: Establish a feedback loop where fraud analysts and end-users can provide insights on the model’s predictions. Analysts’ expertise can offer valuable context and help in identifying areas where the model might need refinement.
- Iterative improvement: Use the feedback and insights gathered from the model’s performance and from the users to iteratively improve the model. This might involve adjusting the features, redefining the thresholds, or even changing the model architecture.
Regulatory compliance and reporting
- Compliance monitoring: Continuously monitor the model’s compliance with relevant financial regulations and data privacy laws. Ensure that all data handling and processing are in line with these regulations.
- Transparent reporting: Maintain transparent records of the model’s performance, the changes made to the model, and the rationale behind these changes. This is important not only for regulatory compliance but also for maintaining stakeholder trust.
Infrastructure and resource optimization
- Scalability checks: Regularly evaluate the infrastructure supporting the model to ensure it can handle the current and projected transaction volumes without compromising on speed or accuracy.
- Resource optimization: Monitor and optimize the computational resources being utilized for model operation. Efficient resource utilization can help in reducing operational costs while maintaining high performance.
Stakeholder communication and education
- Stakeholder updates: Keep all stakeholders, including management, fraud analysts, and IT teams, informed about the model’s performance and any significant changes or updates.
- User education: Continually educate the users of the model, ensuring they understand how to interpret the model’s predictions and the actions to be taken based on these predictions.
Post-deployment is not a passive phase; it requires active and ongoing efforts to ensure the machine learning model remains a robust, accurate, and compliant tool in the fight against financial fraud. Through vigilant monitoring, regular retraining, and continuous improvement, the model can adapt to evolving fraud patterns and maintain its relevance and effectiveness in safeguarding financial assets and interests.
Strengthen Financial Integrity with ML Solutions
Bolster your financial defenses against fraud using machine learning. Dive into our Machine Learning Development Services to implement robust solutions tailored to your security needs.
Key considerations when using ML for fraud detection
Using machine learning for fraud detection in banking is a sophisticated endeavor that promises efficiency but also presents challenges. The effectiveness of ML models hinges significantly on the quality and quantity of data used. To optimize the ML process for fraud detection, it’s essential to consider several critical aspects:
Optimal selection of input variables
- Consideration: While ample data is beneficial, it’s crucial to judiciously select input variables. Overloading models with excessive or irrelevant features can lead to inefficiencies and inaccuracies.
- Strategy: Focus on features that have significant predictive power for fraud detection, such as IP addresses, email addresses, shipping addresses, and average transaction values. This approach not only streamlines algorithm training times but also minimizes the risk of overfitting and ensures that the model remains focused on the most impactful indicators of fraudulent activity.
Regulatory compliance and data privacy
- Consideration: Adherence to data privacy and security laws is paramount. Regulations like China’s Personal Information Protection Law (PIPL), the California Consumer Privacy Act (CCPA), and the European Union’s General Data Protection Regulation (GDPR) set strict guidelines for data collection, usage, and storage.
- Strategy: Ensure that your ML processes are aligned with these regulations, especially in terms of notice/consent practices. Partnering with technical partners who are well-versed in regulatory compliance can be beneficial. These partners should demonstrate a thorough understanding of how to maintain data privacy and security, particularly in the context of banking applications.
Setting appropriate thresholds for decision-making
- Consideration: Establishing the right thresholds for transaction validation is a delicate balance. Overly stringent thresholds may lead to false positives, unnecessarily blocking legitimate transactions and affecting the user experience. Conversely, too lenient thresholds can increase the risk of fraud.
- Strategy: Determine your institution’s risk appetite and set thresholds accordingly. Different financial products or services may require different levels of scrutiny. For instance, a micro-lending institution might afford to set higher thresholds for low-value loans, whereas commercial banks might require stricter controls for products like mortgage loans. Regularly reviewing and adjusting these thresholds based on evolving fraud patterns and changing business objectives is also crucial.
Continuous model training and evaluation
- Consideration: Fraudulent tactics are constantly evolving, and static ML models can quickly become outdated.
- Strategy: Implement a system for continuous learning and model improvement. Regularly update the model with new data, and reassess its performance to ensure it adapts to new fraud patterns and methods. Regularly evaluating model performance through metrics like precision, recall, and the area under the ROC curve (AUC) can provide insights into its effectiveness and guide adjustments.
Integration with overall security architecture
- Consideration: ML models should not operate in isolation but as part of a comprehensive security strategy.
- Strategy: Ensure that ML models for fraud detection are seamlessly integrated with other security systems within the organization. This holistic approach allows for a multi-layered defense strategy, enhancing the overall effectiveness of fraud prevention mechanisms.
In conclusion, while ML provides powerful tools for fraud detection in finance, its success is contingent on thoughtful implementation, mindful of data quality, regulatory compliance, strategic threshold setting, continuous improvement, and integration within the broader security framework. These considerations form the bedrock of a robust, responsive, and reliable fraud detection system that protects financial institutions and their customers from the perils of fraudulent activities.
Future trends and innovations of fraud detection in finance
Integration of artificial intelligence and machine learning: Advanced AI and machine learning technologies are increasingly being integrated into fraud detection systems. These technologies are capable of analyzing vast amounts of data to identify patterns and anomalies that may indicate fraudulent activity. AI algorithms continue to improve over time, learning from new data and adapting to evolving fraud tactics.
Behavioral biometrics: Behavioral biometrics is emerging as a powerful tool in fraud detection. This technology analyzes patterns in human activities, such as typing rhythms, device handling, and navigation patterns, to authenticate users. As this technology matures, it’s expected to become more prevalent in detecting identity theft and account takeover fraud.
Predictive analytics: Predictive analytics use historical data to predict future events. In the context of fraud detection, predictive models analyze past transactions to identify patterns that may indicate future fraudulent activities. These models help in proactively identifying and preventing fraud before it occurs.
Blockchain technology: Blockchain technology, known for its security and transparency, is being explored as a means of preventing fraud, particularly in the realm of identity verification and transaction integrity. The decentralized nature of blockchain makes it difficult for fraudsters to manipulate transaction data.
Robotic Process Automation (RPA): RPA involves using software robots or ‘bots’ to automate routine tasks. In fraud detection, RPA can automate tasks such as data collection and analysis, allowing human analysts to focus on more complex investigations. RPA can improve efficiency and reduce the time taken to detect and respond to fraudulent activities.
Real-time data analysis: The ability to analyze data in real-time is becoming increasingly important in fraud detection. Real-time analysis allows organizations to detect and respond to fraudulent activities as they occur, minimizing potential losses.
Cross-industry collaboration: Fraud detection is benefiting from increased collaboration across different industries and sectors. Sharing information and best practices among organizations can help in identifying new fraud patterns and tactics more quickly and efficiently.
Adoption of advanced security measures: As fraudsters employ more sophisticated methods, financial institutions are responding by adopting advanced security measures. These measures include multi-factor authentication, encryption, and secure access management, among others.
Regulatory Technology (RegTech): The growing complexity of regulatory environments is driving the adoption of RegTech solutions. These solutions use technology to help organizations comply with regulatory requirements more efficiently and effectively, including those related to fraud detection and prevention.
The future of fraud detection in finance is characterized by the adoption of advanced technologies and a proactive approach to identifying and mitigating fraudulent activities. As these trends continue to evolve, they are expected to significantly enhance the ability of organizations to protect their assets and maintain the trust of their customers.
Endnote
As we navigate the complexities of financial fraud, the role of machine learning emerges not just as a tool but as a transformative force, reshaping the landscape of fraud detection and prevention. This exploration has taken us through the nuanced intricacies of fraud in finance, revealing the pivotal role of data precision, the discernment in model selection, and the meticulous craftsmanship involved in training and refining these intelligent systems.
The journey through the evolving dynamics of machine learning in fraud detection underscores a truth – that in the realm of finance, vigilance is not just a practice but a principle. The post-deployment phase, with its emphasis on continuous improvement and adaptability, mirrors the relentless nature of the threats we face, driving home the importance of a proactive stance in safeguarding financial ecosystems.
As we stand at the frontier of innovation, it’s evident that the path forward is one of partnership and collaboration. In this spirit, you need to collaborate with a company, an expert in the domain of machine learning model development. With a dedicated focus on crafting bespoke solutions for fraud detection, the company should stand ready to empower your financial operations, offering a fusion of expertise, innovation, and unwavering commitment to security.
Empower your financial security with cutting-edge machine learning models for fraud detection. Get in touch with LeewayHertz’s ML experts and take the first step towards impenetrable financial security!
Start a conversation by filling the form
All information will be kept confidential.