AI-powered dynamic pricing solutions: Use cases, architecture and future trends
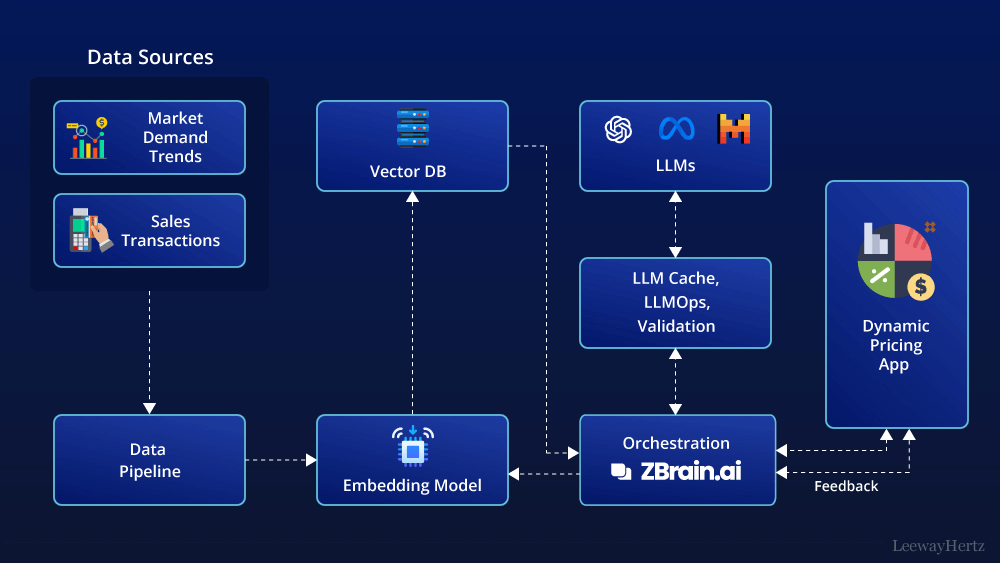
In today’s fast-paced digital marketplace, businesses constantly seek innovative strategies to optimize their pricing strategies and stay ahead of the competition. One such advanced approach is integrating AI into pricing models, giving rise to AI-powered dynamic pricing solutions. These solutions leverage advanced algorithms and data analytics to dynamically adjust prices in real time based on various factors such as demand, competitor pricing, and customer behavior.
Implementing an AI-powered dynamic pricing solution requires a comprehensive understanding of both AI technologies and pricing strategies. This fusion of disciplines empowers businesses to achieve greater profitability, enhance customer satisfaction, and maintain competitiveness in an increasingly dynamic market landscape.
At its core, building an AI-powered dynamic pricing solution involves several key components, including data collection and analysis, algorithm development, and integration with existing pricing systems. Furthermore, considerations such as ethical implications and regulatory compliance must also be taken into account to ensure responsible and sustainable implementation.
Throughout this article, we will explore the fundamental principles behind AI-powered dynamic pricing solutions, examine the benefits and challenges associated with their implementation, and provide practical insights and best practices for businesses looking to harness the power of AI in their pricing strategies.
- An overview of dynamic pricing and its significance
- Challenges in traditional pricing approaches
- Role of AI for precision in dynamic pricing mechanisms
- How does AI for dynamic pricing work?
- Traditional pricing solution vs AI-powered dynamic pricing solution
- Building an AI-powered dynamic pricing system
- LeewayHertz’s development services for AI-powered dynamic pricing solutions
- Key considerations in building AI-powered dynamic pricing solutions
- AI-based dynamic pricing in action: Use cases across diverse industries
- Future trends and innovations in building AI-powered dynamic pricing systems
An overview of dynamic pricing and its significance
Dynamic pricing is a strategy that continuously adapts product or service prices based on factors like demand, competition, and market conditions. This method allows businesses to set agile prices responsive to market fluctuations, optimizing revenue and profit margins. Here, we will delve into the fundamentals of dynamic pricing, its significance, types of strategies, and challenges associated with traditional pricing approaches.
Dynamic pricing, also referred to as surge pricing, time-based pricing, or demand pricing, is a strategy employed by businesses to adjust prices in real-time according to fluctuations in market conditions. Unlike fixed pricing, where prices remain constant regardless of changes in demand or market conditions, dynamic pricing allows businesses to optimize revenue by setting prices that reflect current market dynamics.
The significance of dynamic pricing lies in its ability to maximize revenue and profit margins. By adjusting prices based on demand, seasonality, competitor pricing, and customer behavior, businesses can maximize their product or service value. Dynamic pricing helps businesses stay competitive, adapt to consumer preferences, and seize revenue opportunities.
Types of dynamic pricing strategies
There are different types of dynamic pricing strategies, each tailored to suit different business objectives and market conditions:
- Time-based pricing: Prices vary based on the time of day, day of the week, or season. For example, movie theaters may offer discounted tickets for matinee showings or higher prices for evening screenings.
- Demand-based pricing: Prices fluctuate based on demand levels. During periods of high demand, prices increase to capitalize on revenue opportunities, while prices might be reduced during times of low demand to stimulate sales.
- Competitive-based pricing: Prices are adjusted in response to competitor pricing actions. Businesses monitor competitors’ pricing strategies and adjust their own prices accordingly to remain competitive.
- Segment-based pricing: Pricing is customized for various customer segments, considering factors like buying habits, demographic information, or geographical location. For example, airlines may offer different prices for economy, business, and first-class seats.
Challenges in traditional pricing approaches
Challenges in traditional pricing approaches include:
- Manual analysis: Traditional pricing approaches frequently depend on manual examination of market data, a process that consumes time and is susceptible to inaccuracies.
- Lack of real-time insights: Traditional methods may lack the capability to offer immediate insights into market trends, customer behavior, or competitor pricing strategies, thus impeding the ability to promptly adjust pricing strategies.
- Limited flexibility: Traditional pricing models may lack the flexibility to adjust prices quickly in response to changes in demand, supply, or market conditions.
- Inability to personalize: Traditional pricing methods may struggle to personalize pricing strategies based on individual customer preferences and behaviors, potentially leading to missed revenue opportunities.
- Difficulty in competitor analysis: Traditional approaches may face challenges in effectively analyzing competitor pricing strategies and market dynamics, hindering the ability to stay competitive.
- Pricing inconsistencies: Traditional pricing approaches may result in inconsistencies across different channels or regions, leading to customer confusion or dissatisfaction.
- Reactive approach: Traditional pricing methods often involve a reactive approach, where pricing decisions are made in response to immediate needs or pressures rather than proactive, data-driven strategies.
Overall, the impact of these challenges can result in reduced revenue, decreased market share, damaged brand reputation, and, ultimately, diminished competitiveness. To mitigate these challenges, businesses must embrace more agile, data-driven pricing strategies that prioritize real-time insights, personalization, and proactive decision-making. This may involve leveraging advanced analytics, automation technologies, and AI-powered dynamic pricing optimization tools to stay ahead of the competition and drive sustainable growth.
Optimize Your Operations With AI Agents
Optimize your workflows with ZBrain AI agents that automate tasks and empower smarter, data-driven decisions.
Role of AI for precision in dynamic pricing mechanisms
AI has emerged as an advanced technology in the realm of dynamic pricing, redefining how businesses optimize their pricing strategies. AI-driven dynamic pricing harnesses sophisticated algorithms and machine learning techniques to analyze extensive data sets, facilitating real-time pricing decisions. Unlike traditional pricing methods that rely on manual analysis and predetermined rules, AI-powered dynamic pricing systems can adapt and learn from data, continuously optimizing prices to maximize revenue and profit margins.
AI enables businesses to take a more proactive and data-driven approach to pricing, allowing for greater adaptability and responsiveness to changes in market conditions. Through the integration of AI into dynamic pricing solutions, enterprises can discover fresh avenues for revenue expansion and competitive superiority.
Advantages of AI in dynamic pricing optimization
There are several key advantages of using AI in pricing optimization:
- Real-time insights: AI algorithms can analyze vast amounts of data in real-time, providing businesses with up-to-the-minute insights into market trends, competitor pricing strategies, and customer behavior. This enables organizations to make more informed pricing decisions on-the-fly, maximizing profitability and responsiveness.
- Personalized pricing: AI-powered pricing solutions can leverage customer data and segmentation techniques to offer personalized pricing strategies tailored to individual preferences and purchasing behaviors. This level of customization enhances customer satisfaction and loyalty while optimizing revenue generation for the business.
- Dynamic pricing adjustments: AI algorithms recognize patterns and trends in data, allowing businesses to automatically adjust prices based on changes in demand, supply, or market conditions. This agility enables companies to capitalize on revenue opportunities and minimize the risk of lost sales or excess inventory.
- Competitive advantage: Utilizing AI for pricing optimization empowers businesses to outpace competitors through enhanced pricing precision, responsiveness, and nimbleness. AI algorithms can analyze competitor pricing strategies and market dynamics in real time, enabling organizations to fine-tune their pricing strategies for maximum competitiveness.
- Cost efficiency: AI-powered pricing solutions streamline the pricing process, minimizing the need for manual intervention and minimizing operational costs associated with pricing analysis and optimization. This efficiency lets businesses allocate resources more precisely, focusing on strategic initiatives for growth and innovation.
Overall, AI holds immense potential for transforming pricing optimization processes, enabling businesses to achieve greater profitability, enhance customer satisfaction, and maintain competitiveness in dynamic market environments.
How does AI for dynamic pricing work?
AI transforms dynamic pricing strategies by leveraging advanced analytics, machine learning, and real-time data processing to optimize pricing models, respond to market demands, and maximize revenue. Utilizing advanced Large Language Models (LLMs) and connecting them with a company’s comprehensive datasets enables real-time pricing adjustments based on complex factors such as consumer behavior, competitor prices, and market conditions.
The architecture of the AI-powered dynamic pricing system integrates multiple components and operates as follows:
Data sources: Effective dynamic pricing strategies rely on diverse and comprehensive data inputs, including:
- Market demand data: Real-time and historical data on consumer demand trends influencing pricing decisions.
- Competitor pricing: Data acquired by continuously monitoring competitor pricing strategies and price changes.
- Inventory levels: Data on current stock levels that can impact pricing strategies to either clear out excess inventory or capitalize on scarcity.
- Sales transactions: Detailed records of past sales performances for predicting future sales trends and optimal pricing points.
- Consumer behavior data: Insights into how price changes affect purchasing decisions, gathered from customer interactions and sales data.
- External factors: Seasonal influences and special events that affect market dynamics and pricing strategies.
Data pipelines: The data from these varied sources are processed through sophisticated data pipelines that handle their ingestion, cleaning, and structuring, preparing the data for analysis.
Embedding model: An embedding model transforms the textual data into numerical representations called vectors that AI models can understand. Popular embedding models include those from OpenAI, Google, and Cohere.
Vector database: The generated vectors are saved in a vector database that facilitates efficient querying and retrieval. Notable examples of vector databases include Pinecone, Weaviate, and PGvector.
APIs and plugins: APIs and plugins like Serp, Zapier, and Wolfram play a key role by connecting different components and enabling additional functionalities, such as accessing extra data, integrating with external tools or platforms or performing specific tasks with ease.
Orchestration layer: The orchestrating layer is critical in managing the workflow. ZBrain is an example of this layer that simplifies prompt chaining, manages interactions with external APIs by determining when API calls are required, retrieves contextual data from vector databases, and maintains memory across multiple LLM calls. Ultimately, this layer generates a prompt or series of prompts that are submitted to a language model for processing. The role of this layer is to orchestrate the flow of data and tasks, ensuring seamless coordination across all components within the AI-driven dynamic pricing architecture.
Query execution: The data retrieval and generation process begins when the user submits a query to the dynamic pricing app. Pricing managers and marketing teams submit queries related to pricing adjustments, market trends, or inventory levels.
LLM processing: Once received, the app transmits the query to the orchestration layer. This layer retrieves relevant data from the vector database and LLM cache and sends it to the appropriate LLM for processing. The choice of LLM depends on the nature of the query.
Output: The LLM generates an output based on the query and the data it receives. This output can come in various forms, such as recommended price adjustments, forecasts for demand at different price points, and predictions on competitor pricing reactions.
Dynamic pricing app: This specialized app delivers AI-generated insights in an accessible format. It enables businesses to implement pricing strategies swiftly and effectively, ensuring they remain competitive and responsive to market changes.
Feedback loop: User feedback on the LLM’s output is another important aspect of this architecture. The system incorporates user feedback to continuously improve the accuracy and relevance of the LLM’s outputs.
Agent: AI agents step into this process to address complex problems, interact with the external environment, and enhance learning through post-deployment experiences. They achieve this by employing advanced reasoning/planning, strategic tool utilization, and leveraging memory, recursion, and self-reflection.
LLM cache: Tools like Redis, SQLite, or GPTCache are used to cache frequently accessed information, accelerating the response time of the AI system.
Logging/LLMOps: Throughout this process, LLM operations (LLMOps) tools like Weights & Biases, MLflow, Helicone and Prompt Layer help log actions and monitor performance. This ensures the LLMs operate at peak efficiency and evolve consistently through ongoing feedback mechanisms.
Validation: A validation layer is employed to validate the LLM’s output. This is achieved through tools like Guardrails, Guidance, Rebuff, and LMQL to ensure the accuracy and reliability of the information provided.
LLM APIs and hosting: LLM APIs and hosting platforms are essential for executing dynamic pricing tasks and hosting the application. Depending on the requirements, developers can select from LLM APIs offered by firms such as OpenAI and Anthropic or opt for open-source models. Similarly, they can choose hosting platforms from cloud providers like AWS, GCP, Azure, and Coreweave or opt for opinionated clouds like Databricks, Mosaic, and Anyscale. The choice of LLM APIs and cloud hosting platforms depends on the project’s needs.
This structured flow provides a comprehensive overview of how AI works for dynamic pricing solutions. It leverages data analysis and advanced tools to tailor prices dynamically, optimize revenue, and respond agilely to market conditions.
Traditional pricing solution vs AI-powered dynamic pricing solution
Here’s a detailed comparison table illustrating the fundamental disparities between traditional pricing methods and AI-powered dynamic pricing solutions:
Aspect | Traditional pricing methods | AI-powered dynamic pricing solutions |
---|---|---|
Data utilization | Relies on historical data and market research for pricing decisions. | Utilizes vast amounts of structured and unstructured data, including historical sales data, customer behavior, competitor pricing, and external factors. |
Adaptability and real-time adjustments | Adjustments to pricing are often manual and infrequent, lacking real-time responsiveness. | Continuously monitors market dynamics and consumer behavior, enabling rapid adjustments to prices in real-time. |
Personalization | Pricing strategies tend to be uniform across customer segments. | Tailors prices at the individual level, leveraging customer data to offer personalized pricing and promotions. |
Risk management and optimization | Decisions are based on historical trends and human judgment. | Utilizes predictive analytics and optimization algorithms to forecast demand, identify price elasticity, and simulate pricing scenarios to maximize revenue and profit margins. |
Scalability and efficiency | Limited scalability due to manual processes and resource constraints. | Highly scalable and efficient, capable of processing large datasets and performing complex analyses at scale. Automation reduces manual effort and improves operational efficiency. |
Building an AI-powered dynamic pricing system
Building an AI-powered dynamic pricing system involves a systematic approach that integrates advanced technologies to optimize pricing strategies and enhance competitiveness. Here’s the step-by-step process:1. Define objectives and strategy:
To build an AI-powered dynamic pricing system, begin by defining clear objectives and strategy. Understand what you seek to accomplish through dynamic pricing, whether it’s maximizing revenue, optimizing inventory utilization, or increasing market share. By articulating these goals upfront, you can tailor the pricing model and its implementation to align with your overarching business objectives.
2. Data collection and preprocessing
- Data identification: Use SQL queries or data mining techniques to identify relevant data sources. This involves querying databases and exploring various data repositories to find datasets that contain information crucial for dynamic pricing. These sources may include transaction databases, CRM systems, competitor pricing APIs, and external market data providers. The goal is to gather comprehensive and diverse data that can inform pricing decisions.
- Data acquisition: Employ ETL processes (Extract, Transform, Load) or streaming data pipelines to ingest data from different sources into a centralized data repository. ETL processes encompass extracting data from its source, converting it into an appropriate format, and then transferring it into a designated database or data warehouse. Streaming data pipelines enable real-time data ingestion, which is particularly useful for dynamic pricing systems that require up-to-date information. Ensuring data integrity and consistency during the acquisition process is crucial for accurate analysis and decision-making.
- Data preprocessing: Utilize Python libraries such as Pandas and NumPy to preprocess the collected data. This involves cleaning the data to handle missing values, outliers, and inconsistencies. Common techniques include data imputation to fill in missing values, outlier detection to identify and handle extreme data points, and data cleaning algorithms to correct errors or inconsistencies in the dataset. Preprocessing prepares the data for further analysis and modeling.
- Feature engineering: Utilize feature engineering techniques to transform raw data into meaningful features that can be used for pricing optimization. Feature extraction techniques like Principal Component Analysis (PCA), TF-IDF (Term Frequency-Inverse Document Frequency), or one-hot encoding are commonly used to capture relevant information from the data. PCA reduces the dimensionality of the data while preserving important information, TF-IDF is used for text data to represent the importance of words in a document corpus, and one-hot encoding converts categorical variables into binary vectors. Feature engineering helps to extract valuable insights from the data and improve the performance of pricing models.
3. Choosing the right machine learning model
- Model selection: In the phase of model selection for a dynamic pricing system, it’s crucial to choose the right machine learning model that suits the requirements and objectives of the pricing strategy. Commonly employed models encompass regression techniques like linear regression and logistic regression, decision trees, random forests, gradient-boosting machines, and neural networks. When making this selection, factors such as model complexity, interpretability, and scalability must be carefully considered. Linear regression, for instance, offers simplicity and interpretability but may struggle with capturing complex nonlinear relationships, while neural networks can handle intricate patterns but may require more computational resources. By weighing these factors against the specific needs of the pricing system, an optimal model can be chosen to predict price elasticity and optimize pricing decisions in real-time effectively.
- Model optimization: Utilize techniques such as grid search or Bayesian optimization to tune hyperparameters and optimize model performance, leveraging libraries like Scikit-learn or TensorFlow for model implementation and experimentation.
4. Training the dynamic pricing model
In this phase, the selected machine learning model is trained using historical data. This historical data typically includes information such as past sales, pricing strategies, customer behavior, and market conditions. To train the model effectively, the dataset is split into two subsets: a training set and a validation set. The training set is used to teach the model patterns and relationships within the data, while the validation set is utilized to assess how well the model generalizes to unseen data.
Throughout training, the model learns from the patterns and relationships in the training data, adjusting its internal parameters to minimize prediction errors. Hyperparameters, responsible for controlling the behavior of the learning algorithm, are fine-tuned using techniques such as grid search or random search to optimize model performance.
The overarching objective of model training is to develop a predictive model that accurately forecasts price elasticity and predicts how demand will respond to changes in pricing. By iterative training and evaluating the model using historical data, businesses can build a robust pricing model capable of making informed pricing decisions in real-time.
5. Integration with business rules
The pricing model is adapted to incorporate specific constraints and guidelines relevant to the company’s strategy and objectives. These rules could encompass various factors such as setting minimum and maximum price limits (pricing floors and ceilings), ensuring margins meet certain thresholds, defining different pricing strategies for distinct product categories, and accounting for promotional offers or discounts. By incorporating these business rules into the pricing model, companies ensure that pricing decisions align with their overall business strategy, comply with regulatory requirements, and optimize profitability while considering market dynamics and customer preferences. This integration ensures that the dynamic pricing system operates within the framework of the company’s operational and strategic goals.
6. Real-time data processing
This involves implementing systems to swiftly capture and analyze dynamic changes in market conditions, competitor pricing, and other pertinent factors. This entails setting up mechanisms to gather data in real-time from various sources such as external APIs, social media platforms, news feeds, and through web scraping techniques. By continuously monitoring these sources, businesses can swiftly adapt their pricing strategies to reflect current market trends, competitor actions, and customer sentiment. This real-time data processing capability enables agile decision-making and ensures that the dynamic pricing system remains responsive to rapidly evolving market dynamics, thereby enhancing competitiveness and maximizing revenue opportunities.
7. Dynamic pricing algorithm
The dynamic pricing algorithm is designed to calculate and adjust prices in real time, leveraging predictions from the pricing model and incorporating predefined business rules. Its objective is to strike a balance between maintaining competitiveness and maximizing revenue while also considering customer satisfaction. By continuously analyzing market dynamics, competitor prices, and other relevant factors, the algorithm dynamically adjusts prices to optimize outcomes. This ensures that prices remain competitive, responsive to demand fluctuations, and aligned with business objectives, ultimately enhancing profitability and customer satisfaction.
8. Testing and validation
In the testing and validation phase, the dynamic pricing system undergoes comprehensive evaluation in a controlled setting prior to deployment. This involves validating the model’s performance against historical data and conducting A/B testing to assess its impact on key metrics such as revenue, profit, and customer satisfaction. By subjecting the system to rigorous testing, businesses can ensure its accuracy, reliability, and effectiveness in real-world scenarios. This process aids in identifying any potential issues or areas for improvement before the system is rolled out, thereby mitigating risks and maximizing the chances of success in production.
9. Deployment
Once the dynamic pricing system has been thoroughly tested and validated, it’s time for deployment. Deployment involves implementing the system into the production environment, making it operational and accessible for use by relevant stakeholders within the organization.
During deployment, careful attention must be paid to ensure a seamless transition from testing to live operation. This includes coordinating with IT teams to integrate the dynamic pricing system with existing infrastructure and software systems, ensuring compatibility and seamless functionality. Additionally, user training and documentation should be provided to familiarize stakeholders with the system’s features, functionalities, and usage guidelines.
10. Monitoring and iteration
Monitoring and iteration involve ongoing assessment and refinement of the dynamic pricing system once it’s deployed in a production environment. Continuous monitoring of the system’s performance allows businesses to track key metrics and detect any deviations or anomalies. By analyzing these results and gathering feedback from stakeholders, including customers and internal teams, businesses can identify areas for enhancement and opportunities for optimization. This iterative process involves refining the model, algorithms, and business rules to adapt to evolving market conditions, changing customer preferences, and emerging trends. By continuously iterating and improving the dynamic pricing system, businesses can ensure its effectiveness, relevance, and long-term success in driving revenue growth and enhancing competitiveness.
Building an AI-powered dynamic pricing system requires interdisciplinary expertise in data science, ML, software engineering, and business domain knowledge. Collaboration between data scientists, engineers, and business stakeholders is essential for designing, implementing, and maintaining an effective pricing strategy.
LeewayHertz’s development services for AI-powered dynamic pricing solutions
At LeewayHertz, we develop sophisticated AI-powered dynamic pricing solutions tailored to meet the specific needs of businesses across various sectors. Our strategic AI/ML consulting empowers companies to use artificial intelligence to optimize pricing strategies, enhance revenue management, and stay competitive in volatile markets.
Our proficiency in developing Proof of Concepts (PoCs) and Minimum Viable Products (MVPs) allows businesses to evaluate the practical impact of dynamic pricing tools, ensuring that solutions are effective and specifically designed for their unique market dynamics.
Our expertise in generative AI modernizes routine tasks like market data analysis, price adjustment processes, and sales forecasting by automating them, freeing up teams for strategic planning and execution.
By fine-tuning large language models to the nuances of specific market trends and consumer interactions, LeewayHertz enhances the precision and relevance of AI-driven pricing decisions.
Additionally, we ensure these AI systems integrate seamlessly with existing infrastructures, enhancing data interoperability and enabling real-time pricing decisions that react instantly to market changes.
Our AI solutions development expertise
AI-powered dynamic pricing involves creating systems that adjust prices in real time based on changing market conditions, consumer demand, and inventory levels. These systems integrate advanced data aggregation technologies, compiling and analyzing extensive data from market trends, competitive pricing, and consumer interactions. This robust data foundation supports sophisticated machine learning models that enable businesses to identify optimal pricing points dynamically to maximize revenue and profitability. Additionally, machine learning models continually learn from market feedback to ensure pricing strategies adapt and evolve as conditions change. Key areas covered include promotional pricing, discount management, yield management, and multi-channel price optimization.
Overall, AI-powered dynamic pricing solutions aim to optimize revenue outcomes, adapt quickly to market conditions, and improve customer satisfaction through competitive pricing strategies.
AI agent/copilot development for dynamic pricing
LeewayHertz builds custom AI agents and copilots that enhance various aspects of pricing and revenue management, enabling companies to quickly make sophisticated and responsive pricing decisions. Here’s how they help:
Market sensitivity analysis:
- Continuously monitor market conditions and consumer purchase behaviors to anticipate changes in demand.
- Implement predictive analytics to forecast how price adjustments affect sales volume, helping to strategize profitable pricing.
- Analyze competitors’ pricing strategies to ensure competitive positioning in the market.
Real-time pricing optimization:
- AI agents assist in dynamically suggesting pricing adjustments based on real-time data, with human oversight ensuring that all price changes align with broader business strategies and market conditions.
- Enable scenario planning and testing to predict outcomes from different pricing strategies, providing a strategic advantage in decision-making.
- Customize prices across different geographies and channels to maximize overall sales and profitability.
Inventory and sales integration:
- Sync dynamic pricing models with inventory management systems to adjust prices based on stock levels and logistical considerations.
- Predict and manage the supply chain impacts by adjusting prices to smooth out demand spikes or clear excess inventory.
- Align pricing decisions with sales strategies to optimize both revenue and inventory turnover.
Customer segmentation and engagement:
- Segment customers based on buying patterns and price sensitivity, crafting targeted pricing strategies that appeal to different segments.
- Develop personalized promotions and discounts that attract and retain customers, boosting customer loyalty.
- Deliver tailored pricing to individual customers in real-time, enhancing the customer experience and increasing conversion rates.
Compliance and ethical considerations:
- Ensure that dynamic pricing strategies comply with all relevant regulations and maintain ethical standards.
- Monitor pricing practices continuously, preventing discriminatory pricing and maintaining fairness across customer groups.
- Maintain transparency with customers about how prices are determined, fostering trust and long-term relationships.
LeewayHertz’s AI agents and copilots streamline pricing processes and significantly enhance the effectiveness of pricing strategies. By integrating these advanced AI solutions into existing systems, companies can navigate the complexities of market dynamics with innovative, efficient, and responsive tools, achieving a substantial competitive edge.
Key considerations in building AI-powered dynamic pricing solutions
Building AI-powered dynamic pricing systems involves several key considerations to ensure effectiveness, fairness, and ethical use. Here are some of the essential considerations:
- Data quality and availability: High-quality data is crucial for training AI models. Ensure that you have access to relevant and reliable data, including historical pricing data, customer demographics, market trends, and competitor pricing.
- Algorithm selection: Based on the complexity of your pricing strategy and the available data, choose appropriate algorithms for dynamic pricing. Common approaches include machine learning algorithms like regression, classification, reinforcement learning, and time-series analysis.
- Ethical and legal compliance: Businesses must prioritize fairness, transparency, and alignment with ethical principles when implementing dynamic pricing strategies. Providing clear explanations of pricing algorithms and factors influencing prices fosters trust and confidence among customers. Additionally, active engagement with stakeholders, including customers, regulators, and advocacy groups, is crucial to address concerns and ensure that pricing strategies meet ethical standards and customer expectations. By prioritizing ethical considerations and customer transparency, businesses can build sustainable and socially responsible dynamic pricing systems that benefit both the organization and its customers.
- Fairness and bias mitigation: Guard against biases in the data and algorithms that could lead to unfair pricing practices. Implement fairness-aware techniques to identify and mitigate biases in pricing decisions, especially concerning sensitive attributes like race, gender, or socioeconomic status.
- Dynamic market analysis: Continuously monitor market conditions, competitor pricing strategies, and customer behavior to adjust pricing dynamically. Real-time data analysis and market segmentation can help tailor pricing strategies to different customer segments and market segments.
- Customer segmentation: Segment customers based on their preferences, purchasing behavior, and willingness to pay. Customize pricing strategies for different customer segments to maximize revenue while ensuring customer satisfaction.
- Demand forecasting: Develop accurate demand forecasting models to predict customer demand and price elasticity. Understanding how changes in price affect demand can help optimize pricing strategies to maximize revenue and profit margins.
- Optimization objectives: Define clear optimization objectives, such as maximizing revenue, profit, market share, or customer satisfaction. Customize pricing algorithms and strategies to align with your business goals and objectives.
- Experimentation and testing: Conduct A/B testing and experiments to evaluate the effectiveness of different pricing strategies. Test new pricing algorithms and strategies in controlled environments before implementing them in production to minimize risks.
- Monitoring and feedback: Implement robust monitoring systems to track the performance of dynamic pricing algorithms in real-time. Monitor key performance indicators (KPIs) such as revenue, profit, customer churn, and customer satisfaction. Collect feedback from customers and stakeholders to refine pricing strategies continuously.
- Scalability and performance: Ensure that your dynamic pricing system is scalable and can handle large volumes of data and real-time pricing decisions. Optimize algorithms and infrastructure for performance to minimize latency and ensure timely pricing updates.
- Human oversight: While AI can automate pricing decisions, human oversight is essential to review and validate pricing recommendations, especially for high-stakes pricing decisions or when ethical considerations are involved. Human experts can provide domain knowledge and intuition that AI algorithms may lack.
By considering these key factors, organizations can build AI-powered dynamic pricing systems that optimize revenue, enhance competitiveness, and deliver value to customers while adhering to ethical and legal standards.
Optimize Your Operations With AI Agents
Optimize your workflows with ZBrain AI agents that automate tasks and empower smarter, data-driven decisions.
AI-based dynamic pricing in action: Use cases across diverse industries
Dynamic pricing has widespread applications across various industries, enabling businesses to optimize revenue and stay competitive. Here are some use cases and examples of dynamic pricing implementation:
E-commerce sector: E-commerce platforms utilize dynamic pricing to adjust product prices in real-time based on factors such as demand, competitor pricing, and customer behavior. For instance, online retailers may offer personalized discounts or adjust prices during flash sales to stimulate purchases and maximize revenue.
Travel and hospitality industry: Hotels, airlines, and travel agencies leverage dynamic pricing to adjust room rates, ticket prices, and vacation packages dynamically. This allows them to capitalize on fluctuations in demand, seasonal trends, and booking patterns, optimizing revenue while ensuring maximum occupancy and resource utilization.
Retail and consumer goods: Retailers and consumer goods companies implement dynamic pricing to optimize pricing strategies across various products and categories. For example, supermarkets may adjust prices based on inventory levels, expiration dates, and promotional campaigns, while fashion retailers may use dynamic pricing to clear excess inventory or capitalize on trends.
Industrial B2B markets: Business-to-business (B2B) and industrial sectors leverage dynamic pricing to negotiate contract terms, adjust pricing based on order volumes, and manage pricing agreements with suppliers and distributors. This allows them to optimize pricing structures, improve profit margins, and maintain competitive pricing in complex supply chains.
Transportation and logistics: Transportation and logistics companies utilize dynamic pricing to optimize freight rates, shipping fees, and transportation services based on factors such as fuel costs, demand-supply dynamics, and route efficiency. Dynamic pricing enables these companies to maximize resource utilization, minimize empty miles, and improve overall operational efficiency.
Entertainment and events: Entertainment venues, concert organizers, and event management companies leverage dynamic pricing to adjust ticket prices based on factors such as seat availability, demand for specific events, and proximity to the event date. Dynamic pricing helps optimize ticket sales, maximize revenue, and manage capacity constraints for popular events and performances.
Healthcare and pharmaceuticals: Healthcare providers and pharmaceutical companies implement dynamic pricing strategies to optimize pricing for medical services, prescription drugs, and healthcare products. Dynamic pricing enables healthcare organizations to adjust prices based on factors such as patient demographics, insurance coverage, and market demand, ensuring affordability and accessibility of healthcare services while maximizing revenue.
Financial services: Financial institutions and fintech companies utilize dynamic pricing to optimize pricing for banking services, insurance products, and financial instruments. Dynamic pricing enables banks and insurance companies to tailor pricing strategies based on factors such as risk assessment, customer segmentation, and market conditions, optimizing revenue while meeting regulatory requirements and customer expectations.
In each of these sectors, dynamic pricing solutions powered by AI enable businesses to adapt to changing market conditions, optimize pricing strategies, and drive revenue growth while delivering value to customers. As dynamic pricing continues to evolve, businesses across industries will increasingly rely on AI-powered solutions to gain a competitive edge and thrive in today’s dynamic market landscape.
Future trends and innovations in building AI-powered dynamic pricing systems
As technology continues to advance rapidly, the future of AI-powered dynamic pricing systems holds several promising trends and innovations. Here are some key developments to watch out for:
- Advanced AI algorithms: Future dynamic pricing systems will leverage advanced AI algorithms, including deep learning techniques such as recurrent neural networks (RNNs) and transformers. These algorithms will capture complex patterns more accurately, improving demand forecasting and pricing optimization.
- Real-time decision-making: With the advancement of computing power and algorithm efficiency, dynamic pricing systems will become increasingly capable of making real-time pricing decisions. This will enable businesses to respond instantly to changes in market conditions, leading to more agile and competitive pricing strategies.
- Personalized pricing: Future dynamic pricing systems will focus on delivering personalized pricing strategies tailored to individual customers. By leveraging customer data and behavioral analytics, businesses can offer pricing that reflects each customer’s preferences, purchasing history, and willingness to pay.
- Ethical and fair pricing: There will be a growing emphasis on ethical and fair pricing practices in dynamic pricing systems. Businesses will need to ensure that their pricing strategies are transparent, unbiased, and equitable, addressing concerns related to price discrimination and fairness.
- Integration with emerging technologies: Dynamic pricing systems will increasingly integrate with emerging technologies such as blockchain and Augmented Reality (AR). These technologies will provide additional data sources and insights for pricing optimization, leading to more accurate and effective pricing strategies. While the integration of these technologies with dynamic pricing systems is feasible, it requires careful consideration of technical, ethical, and legal aspects. For instance, businesses must ensure that the use of AR respects customer privacy and that blockchain implementations comply with data protection regulations. Additionally, the cost and complexity of implementing these technologies should be weighed against the potential benefits of pricing optimization.
- Autonomous pricing systems: The future may see the development of autonomous pricing systems that can self-learn, self-optimize, and adapt to changing market conditions without human intervention. These systems will leverage reinforcement learning and other advanced techniques to continuously improve pricing strategies.
- Collaborative pricing ecosystems: Businesses may collaborate within pricing ecosystems to share data and insights for mutual benefit. These collaborative efforts can lead to collective intelligence and better-informed pricing decisions across industries.
- Regulatory compliance: With increasing scrutiny of AI algorithms and data privacy, future dynamic pricing systems will need to ensure compliance with regulatory frameworks such as CCPA. Businesses will need to prioritize data security, transparency, and accountability in their pricing practices.
The future of AI-powered dynamic pricing systems will be characterized by advancements in AI algorithms, real-time decision-making capabilities, personalized pricing strategies, ethical considerations, integration with emerging technologies, and the development of autonomous pricing systems. These trends promise to redefine pricing strategies and enhance competitiveness in the marketplace.
Endnote
Building an AI-powered dynamic pricing solution represents a pivotal step toward achieving greater efficiency, competitiveness, and profitability in modern business operations. By harnessing the power of AI and advanced machine learning algorithms, organizations can unlock unprecedented capabilities in pricing optimization. This article has explored the fundamental concepts of dynamic pricing, the role of AI in reshaping pricing strategies, and the key components involved in building an AI-powered dynamic pricing system.
From data collection and preprocessing to model training, evaluation, and deployment, each step in the process plays a crucial role in the success of dynamic pricing initiatives. The integration of AI enables businesses to analyze vast amounts of data, identify patterns, and make real-time pricing decisions with precision and agility. Moreover, AI-powered dynamic pricing systems offer the flexibility to adapt to changing market conditions, personalize pricing strategies, and optimize revenue opportunities.
Looking ahead, the future of AI-powered dynamic pricing solutions holds immense promise, with advancements in AI algorithms, integration with emerging technologies, and the development of autonomous pricing systems. However, organizations need to address ethical considerations, regulatory compliance, and customer trust to ensure the responsible and ethical use of AI in pricing practices. Building an AI-powered dynamic pricing solution is not merely about adopting new technology but about embracing a transformative mindset that prioritizes data-driven decision-making, innovation, and customer-centricity. By leveraging AI to optimize pricing strategies, organizations can stay ahead of the curve, drive sustainable growth, and deliver greater value to customers in an increasingly competitive marketplace.
Ready to transform your pricing strategy? Partner with LeewayHertz, experts in AI consulting and development services, to build your AI-powered dynamic pricing solution and stay ahead in today’s competitive market landscape.
Start a conversation by filling the form
All information will be kept confidential.