AI in loan underwriting: Use cases, architecture, technologies, solution and implementation
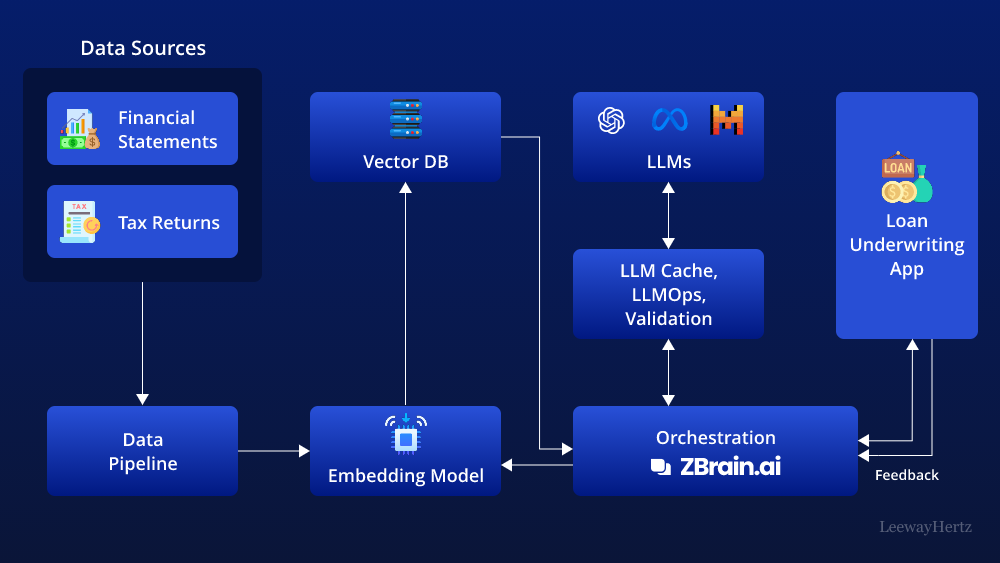
In the intricate landscape of loan underwriting, financial institutions encounter a myriad of challenges that can impede the efficiency and accuracy of the loan sanctioning process. Traditional underwriting methods often grapple with time-consuming tasks, complex data analysis, and the potential for human errors, leading to delays and increased operational costs. The need for a more streamlined and technologically advanced approach has prompted a paradigm shift in the industry, and Artificial Intelligence (AI) has emerged as a transformative force poised to redefine the loan underwriting landscape.
One of the primary hurdles faced by underwriters lies in the exhaustive process of collecting, verifying, and analyzing vast amounts of borrower data. This manual approach not only consumes considerable time but also exposes the lender to the risk of overlooking crucial details or miscalculating risk factors. Furthermore, the evolving nature of financial markets demands a nimble and adaptive underwriting process, a feat that conventional methods struggle to achieve.
Enter AI, a technological powerhouse that harnesses machine learning algorithms to process colossal datasets swiftly and accurately. The integration of AI in loan underwriting introduces automation to mundane tasks, from data collection to risk assessment, significantly reducing the time and resources expended in the process. By learning from historical data patterns and continuously adapting to evolving market dynamics, AI-driven underwriting systems enhance predictive accuracy, allowing financial institutions to make more informed and timely decisions.
This article delves into the transformative role of AI in loan underwriting, exploring how machine learning algorithms streamline data processing, fortify risk assessments, and ultimately expedite loan approval processes. By shedding light on the challenges faced by traditional underwriting methods, the article will unravel the innovative solutions AI brings to the forefront, ushering in a new era of efficiency, precision, and agility in loan sanctioning.
- What is loan underwriting?
- Challenges and limitations in traditional loan underwriting
- A brief overview of AI in loan underwriting
- How does AI in loan underwriting work?
- Use cases of AI in loan underwriting
- How does LeewayHertz’s generative AI platform optimize loan underwriting processes?
- LeewayHertz’s AI development services for loan underwriting
- The operational advantages of AI in loan underwriting
- The future of AI in loan underwriting
What is loan underwriting?
Loan underwriting is the process by which a financial institution evaluates the creditworthiness of a borrower and assesses the risk associated with lending money to them. During underwriting, the lender reviews various aspects of the borrower’s financial situation, including credit history, income, employment stability, and debt-to-income ratio. The objective is to assess the probability that the borrower can repay the loan, considering their financial profile. Lenders use underwriting to make informed decisions on whether to approve, modify, or deny a loan, as well as to establish the terms and conditions, such as interest rates and loan amounts. The underwriting process is crucial for managing risk and ensuring responsible lending practices.
Challenges and limitations in traditional loan underwriting
Conventional loan underwriting encompasses a thorough evaluation of a borrower’s creditworthiness and their capacity to repay a loan. However, there are many challenges associated with these traditional underwriting methods. Here are some of them:
- Time-consuming process: Traditional underwriting processes can be lengthy, involving significant paperwork and manual review of financial documents. This can result in delays, making it challenging for borrowers to obtain funds quickly.
- High cost: The manual nature of traditional underwriting processes contributes to high operating costs for lenders. This can be a limiting factor for both borrowers and lenders, as it may result in higher interest rates or fees for borrowers and lower profit margins for lenders.
- Limited data sources: Traditional underwriting methods often rely on a limited set of financial data, such as credit scores and income statements. This might not offer a holistic view of a borrower’s financial well-being and capacity to repay a loan, particularly for those with limited credit histories or fluctuating income.
- Subjectivity: Human judgment plays a pivotal role in traditional underwriting, which can introduce subjectivity and inconsistency in decision-making. This may lead to unfair outcomes or biased decisions.
- Risk of fraud: Manual underwriting processes may be susceptible to fraudulent activities, as it can be challenging to thoroughly verify the authenticity of all submitted documents.
- Inflexibility: Traditional underwriting methods may not adapt well to changing economic conditions or unique financial situations. This lack of flexibility can be a limitation in assessing unconventional borrowers or those facing temporary financial challenges.
- Inability to assess non-traditional income sources: Traditional underwriting methods may struggle to accurately assess income from non-traditional sources, such as freelancing, gig work, or rental income. This limitation can impact the ability of some individuals to qualify for loans.
- Limited use of technology: Many traditional underwriting processes still rely on outdated systems and manual data entry. The limited use of technology can hinder efficiency, accuracy, and the ability to incorporate advanced analytics and machine learning.
To address these challenges, the financial industry is increasingly exploring and adopting innovative technologies such as AI, machine learning, and alternative data sources to enhance the efficiency and effectiveness of loan underwriting processes. These advancements aim to streamline operations, reduce costs, and provide a more accurate assessment of a borrower’s creditworthiness.
Launch your project with LeewayHertz!
Enhance your underwriting processes with AI integration. Collaborate with LeewayHertz for custom solutions, ensuring heightened efficiency and accuracy.
A brief overview of AI in loan underwriting
Recognizing the transformative impact of artificial intelligence on the traditional lending landscape is essential in understanding AI in loan underwriting. AI is transforming the underwriting process by harnessing advanced machine learning algorithms and data analytics, facilitating more informed and efficient credit decisions. In contrast to traditional methods, AI-enabled underwriting rapidly analyzes vast datasets, incorporating alternative sources like social media activity, transaction histories, and behavioral patterns. This capability enables a more thorough and nuanced evaluation of a borrower’s creditworthiness, proving especially advantageous for individuals with limited credit histories. Furthermore, AI-driven models exhibit adaptability to changing economic conditions, enhance accuracy, and minimize human bias in decision-making. The integration of AI in loan underwriting not only streamlines processes but also promotes greater financial inclusion by providing a more holistic assessment of borrowers.
How does AI in loan underwriting work?
Integrating AI into loan underwriting utilizes various components to automate data analysis, expedite processing, extract actionable insights, and enable informed lending decisions. This surpasses conventional methods by integrating robust Large Language Models (LLMs) with the financial institution’s unique knowledge base. This approach enhances the comprehensiveness of analysis, facilitating prompt lending decisions. The architecture integrates multiple components to streamline and optimize the loan underwriting process. Here’s a detailed breakdown of how it operates:
1. Data sources: The initial step involves collecting data pertinent to the loan underwriting process from diverse sources. This data can include:
- Credit bureaus: Credit reports from major credit bureaus such as Equifax, Experian, and TransUnion to assess the borrower’s credit history, payment behavior, and overall creditworthiness.
- Financial statements: Financial statements provided by the borrower, including income statements, balance sheets, and cash flow statements, to evaluate their financial health and ability to repay the loan.
- Tax returns: Tax returns for the past few years to verify the borrower’s income and assess their tax liabilities, deductions, and financial stability.
- Employment verification: The borrower’s employment status and income by contacting their employer directly or requesting employment verification documents.
- Bank statements: Bank statements to understand the borrower’s cash flow, spending patterns, and financial habits, which can provide insights into their ability to manage debt and repay the loan.
- Asset documentation: Documentation related to the borrower’s assets, such as real estate holdings, investment accounts, and other valuable assets, to assess their overall financial position and collateral available for the loan.
- Credit references: Credit references from other lenders or creditors to gather additional information about the borrower’s creditworthiness and repayment history.
2. Data pipeline: The data gathered from the previous sources is subsequently channeled through data pipelines. These pipelines handle tasks such as data ingestion, cleaning, processing (including data transformations like filtering, masking, and aggregations), and structuring, thereby preparing it for subsequent analysis.
3. Embedding model: The processed data is segmented into chunks and fed into an embedding model. This model converts textual data into numerical representations called vectors, enabling AI models to comprehend it effectively. Well-known models for this purpose are developed by OpenAI, Google, and Cohere.
4. Vector database: The resulting vectors are stored in a vector database, facilitating streamlined querying and retrieval processes. This database efficiently manages the storage, comparison, and retrieval of potentially billions of embeddings (i.e., vectors). Prominent examples of such vector databases include Pinecone, Weaviate, and PGvector.
5. APIs and plugins: APIs and plugins such as Serp, Zapier, and Wolfram serve a critical function by linking various components together and facilitating additional functionalities, such as accessing supplementary data or executing specific tasks seamlessly.
6. Orchestration layer: The orchestrating layer plays a pivotal role in managing the workflow. An example of this layer is ZBrain, which streamlines tasks like prompt chaining, handling interactions with external APIs to determine when API calls are necessary, fetching contextual data from vector databases, and preserving memory across multiple LLM calls. This layer generates a prompt or series of prompts for submission to a language model for processing. Overall, its responsibility lies in orchestrating data flow and tasks, ensuring smooth coordination among all other components in this architecture.
7. Query execution: The data retrieval and generation process initiates when the user submits a query to the loan underwriting application. This query can be related to any aspect relevant to the borrower, encompassing factors like their financial history, creditworthiness, employment status, and loan requirements.
8. LLM processing: Upon receiving the query, the application forwards it to the orchestration layer. This layer then retrieves pertinent data from the vector database and LLM cache, sending it to the suitable LLM for processing, with the selection of the LLM dependent upon the query’s nature.
9. Output: The LLM generates an output based on the query and the data it receives. This output can take various forms relevant to loan underwriting processes, such as assessments of the borrower’s creditworthiness, identification of potential risks, generation of draft loan agreements, or summarizing the borrower’s financial profile.
10. Loan underwriting app: The verified output is then presented to the user via the loan underwriting app. This core application consolidates all data, analysis, and insights, presenting the findings in a user-friendly format and enabling decision-makers to review and act upon the information provided.
11. Feedback loop: User feedback on the LLM’s output is a crucial component of this architecture. This feedback is utilized to continuously enhance the accuracy and relevance of the LLM’s results, ensuring better performance over time.
12. Agent: AI agents play a vital role in this process by solving complex problems, interacting with the external environment, and enhancing learning through post-deployment experiences. They accomplish this by employing advanced reasoning and planning, strategically utilizing tools, and memory, recursion, and self-reflection.
13. LLM cache: To expedite the AI system’s response time, frequently accessed information is cached using tools like Redis, SQLite, or GPTCache.
14. Logging/LLMOps: During this process, LLMOps tools such as Weights & Biases, MLflow, Helicone, and Prompt Layer aid in logging actions and monitoring performance, ensuring optimal functionality of the LLMs and facilitating continuous improvement through feedback loops.
15. Validation: A validation layer is utilized to authenticate the LLM’s output, accomplished through tools such as Guardrails, Rebuff, Guidance, and LMQL, ensuring the precision and dependability of the information shared.
16. LLM APIs and hosting: LLM APIs and hosting platforms are integral for executing loan underwriting tasks and hosting the application. Depending on project requirements, developers can choose from LLM APIs like OpenAI and Anthropic or explore open-source models. Similarly, they have various hosting platform options, including cloud providers such as AWS, GCP, Azure, and Coreweave, or opinionated clouds like Databricks, Mosaic, and Anyscale. The selection of LLM APIs and cloud hosting depends on the unique needs and preferences of the project.
This flow offers a comprehensive insight into how AI streamlines loan underwriting, utilizing diverse data sources and technological tools to produce precise and actionable insights. Ultimately, by automating various loan underwriting tasks, AI enhances efficiency, mitigates risks and improves the overall quality of lending decisions.
Use cases of AI in loan underwriting
AI plays a significant role in transforming various industries, and loan underwriting is no exception. Here are some key use cases of AI in loan underwriting:
Creditworthiness assessment
In the dynamic landscape of commercial lending, the traditional reliance on robust credit scores is evolving with the adoption of automated credit decisioning systems, exemplifying a key use case for AI in loan underwriting. Contemporary lenders are departing from conventional practices, implementing alternative scoring models powered by AI to evaluate customers’ creditworthiness seamlessly. This transformative approach involves efficiently assessing credit scores against predefined standards, utilizing customer bureau data and AI-based credit scoring to ascertain eligibility. Eligible customers are presented with personalized credit offers, and upon acceptance, the streamlined process continues through automated KYC and digital client onboarding. This integration of AI not only expedites the credit assessment but also ensures rapid loan disbursal, addressing the growing demand for a faster and more responsive lending ecosystem. This use case stands as a testament to ongoing innovation and investment in commercial lending, aligning with the contemporary expectation for a swift and efficient credit assessment and disbursal procedure precisely when customers need it.
Elevating customer experience
In banking and credit lending, the integration of AI goes beyond traditional roles, transforming institutions into financial allies for their account holders. Beyond offering standard financial products, such as loans and instruments, banks are leveraging AI to craft a more enriching customer experience. AI’s role begins with the creation of detailed and data-rich customer profiles derived from the meticulous analysis of transaction line items on current accounts or card statements.
Beyond the typical B2B engagement, banks step into the realm of personalized financial companionship. This evolution allows them to provide tailored advice on savings, significant purchases, financial literacy guidance, and pivotal decision-making support. By harnessing AI, banks not only enhance their traditional offerings but also add substantial value to customers’ lives. Digital lending platforms leverage data to extend beyond financial transactions, enabling banks to notify specific customers about exclusive deals and offers tied to their credit reputation. This proactive approach not only cultivates a more experiential banking environment but also streamlines loan operations, forging a seamless and personalized journey for customers.
Streamlined loan processing
The integration of AI in loan underwriting is redefining commercial lending, offering a seamless and digitally-driven experience akin to personal loan applications. While certain regulatory constraints still impact the complete digitization of lending, such as mortgage loans and payments, there is a growing push to automate at least 95% of manual underwriting decisions across various banking domains.
In the domain of SME lending, both traditional banks and fintechs are prioritizing digitization, albeit with caution, in the corporate lending sphere. Rather than a complete overhaul of customer experiences, banks are incrementally refining standard processes, exemplified by the digitization of credit proposal papers and the automation of annual reviews to enhance efficiency.
Strategic initiatives are also underway, focusing on aiding corporate transaction approvers by automating low-risk credit renewals. This ensures that valuable manual resources can be directed toward intricate and high-credit-risk deals. AI’s role in automating data aggregation equips relationship managers with instant access to comprehensive data and risk-evaluation scores, incorporating financial and industry performance, market and sentiment analysis, important news, and external factors. This not only streamlines operations but also empowers businesses to navigate lending decisions with precision and agility, marking a significant leap forward in the landscape of commercial loan underwriting.
Data remediation in loan applications
Addressing data quality, specifically the accuracy, completeness, and reliability of personal, financial, and credit-related information in loan applications, is a critical focus area for AI technologies within the domain of loan underwriting. Recognizing this, banks and lending institutions have instituted comprehensive programs that prioritize data measurement, quality analysis, and the remediation of detected issues. However, traditional remediation processes often prove time-consuming, creating substantial backlogs in operations and requiring significant human resources for data scrubbing.
The infusion of AI intervention and technological integration redefines this landscape, introducing more efficient processes for prioritizing and remediating issues on a broader scale. Dedicated funds are allocated for the implementation of AI-integrated programs and the automation of remediation processes. Validated machine learning models are applied to scrutinize inputs, facilitating the development of analytics-driven models that ensure faster and more accurate decisions in the data remediation process. This strategic integration of AI not only expedites data remediation efforts but also enhances precision, offering a transformative approach to handling data quality in the context of loan applications.
Fortifying loan underwriting against fraud
The surge in cybercrimes amid the digital evolution necessitates robust fraud monitoring in loan underwriting, and AI emerges as a pivotal ally in this realm. In the face of identity thefts, phishing scams, ransomware attacks, and data theft becoming prevalent, the integration of AI and blockchain technology stands out as a game-changer.
AI, coupled with blockchain-powered smart contracts, reshapes identity verification for loan borrowers, ensuring both speed and security. These smart contracts monitor and regulate loans throughout their lifecycle, mitigating the risks of defaults, delayed reporting, and unreported loans. AI-driven solutions offer a shared control view of historical records, authenticate financial information and data, and provide shared access and consensus, minimizing the scope for fraudulent activities. This not only ensures efficient governance but also enhances transparency in the underwriting process. As banks and companies embrace an “AI-first” approach, the foundational importance of a sustainable and sturdy technological backbone cannot be overstated. In the quest for rapid technological advancements, the risk of poor security and fraud looms large. Therefore, rigorous security testing becomes imperative, safeguarding against potential threats and vulnerabilities before innovative AI solutions are deployed for the greater good.
Data extraction through OCR in loan underwriting
Optical Character Recognition (OCR) emerges as a specialized solution, decoding textual information within images and facilitating a transformative approach to data extraction. OCR seamlessly converts text-containing images into computer-readable characters, shaping the future course of action for financial institutions. Banks and commercial lending firms leverage OCR to extract crucial data from physical documents such as cheques, encompassing account information, handwritten amounts, and signatures for authorization. Loan applications laden with diverse documents and information witness a streamlined data extraction process powered by AI technologies. Deep learning enhances this capability, capturing additional data points through OCR to yield more comprehensive outcomes. This not only expedites data recording and maintenance but also contributes to faster decision-making in the loan underwriting process, underscoring the efficiency gains achievable through the integration of OCR technology.
Loan underwriting through behavioral analytics
Advanced behavioral analytics stands as a transformative tool, reshaping how banks and fintech firms process transactions and gain insights from primary operating accounts. Operational instruments have been developed to meticulously classify transactions into detailed revenue and expense items, while advanced behavioral analytics takes this a step further. By harnessing the power of behavioral analytics, banks and institutes can generate simplified financial statements, evaluate affordability ratios, and conduct real-time analyses of customer and supplier concentrations. The wealth of transactional data not only provides updated insights but also serves as a foundation for substantially richer and more pragmatic data solutions, laying the groundwork for iterative data improvement models. A standardized behavioral analytics model becomes the catalyst for innovation in customer experiences and the enhancement of the lending process, exemplifying how AI-driven insights elevate the efficiency and precision of loan underwriting practices.
By leveraging AI in loan underwriting, financial institutions can enhance efficiency, reduce risks, and make more informed lending decisions, ultimately benefiting both lenders and borrowers.
How does LeewayHertz’s generative AI platform optimize loan underwriting processes?
LeewayHertz’s generative AI platform, ZBrain, plays a transformative role in empowering financial institutions by optimizing loan underwriting processes and facilitating swift, efficient loan approvals. As a comprehensive, enterprise-ready platform, ZBrain empowers businesses to design and implement applications tailored to their specific operational requirements. The platform uses clients’ data, whether in the form of text, images, or documents, to train advanced LLMs like GPT-4, Vicuna, Llama 2, or GPT-NeoX for developing contextually aware applications capable of performing diverse tasks.
Enterprises encounter a multifaceted challenge in loan underwriting, grappling with issues spanning data accuracy, regulatory compliance, risk assessment, fraud prevention, subjective decision-making, meticulous documentation management, and adapting to dynamic economic conditions. ZBrain effectively addresses these challenges through its distinctive feature called “Flow,” which provides an intuitive interface that allows users to create intricate business logic for their apps without the need for coding. Flow’s easy-to-use drag-and-drop interface enables the seamless integration of large language models, prompt templates, and other genAI models into your app’s logic for its easy conceptualization, creation, or modification.
To comprehensively understand how ZBrain Flow works, explore this resource that outlines a range of industry-specific Flow processes. This compilation highlights ZBrain’s adaptability and resilience, showcasing how the platform effectively meets the diverse needs of various industries, ensuring enterprises stay ahead in today’s rapidly evolving business landscape.
ZBrain applications facilitate organizations in elevating their loan underwriting workflows, resulting in heightened efficiency, accelerated decision-making, enhanced accuracy in risk assessment, robust fraud detection, cost reduction, consistent and fair application of underwriting criteria, improved customer experiences, and streamlined compliance management, all while upholding stringent data privacy standards.
LeewayHertz’s AI development services for loan underwriting
At LeewayHertz, we build AI solutions tailored to meet the specific needs of loan underwriting processes. Our strategic AI/ML consulting empowers financial institutions to leverage AI for improved decision-making, enhanced risk assessment, and optimized loan processing.
Our expertise in developing Proof of Concepts (PoCs) and Minimum Viable Products (MVPs) allows us to demonstrate the practical application of AI in the loan underwriting process. This allows institutions to test and refine AI-driven solutions for tasks like document verification, risk scoring, and automated decisioning, ensuring they are effective and meet the specific needs of their lending operations.
Our work in generative AI also transforms routine tasks like data analysis and document verification, automating these processes to free up underwriters for more strategic roles.
By fine-tuning large language models to the nuances of financial terminology and loan evaluation criteria, LeewayHertz enhances the accuracy and relevance of AI-driven underwriting processes.
Additionally, we ensure these AI systems integrate seamlessly with existing technological infrastructures, enhancing operational efficiency and decision-making in loan underwriting.
Our AI solutions development expertise
AI solutions development for loan underwriting typically involves creating systems that enhance decision-making, automate routine tasks, and personalize loan evaluations. These solutions integrate key components such as data aggregation technologies, which compile and analyze financial information from diverse sources. This comprehensive data foundation supports predictive analytics capabilities, enabling the accurate assessment of borrower risk profiles.
Additionally, machine learning algorithms are employed to evaluate loan applications based on various criteria, ensuring that each application is assessed accurately and efficiently. These solutions often cover areas like credit scoring, risk assessment, regulatory compliance, and loan portfolio management.
Overall, AI solutions in loan underwriting aim to optimize financial outcomes, improve efficiency, and elevate the client experience.
AI agent/copilot development for loan underwriting
LeewayHertz builds custom AI agents and copilots that enhance various loan underwriting operations, enabling companies to save time and resources while facilitating faster decision-making. Here is how they help:
Credit scoring and risk assessment:
- Automating the analysis of credit reports and financial statements to assess borrower risk.
- Utilizing predictive analytics to evaluate the likelihood of loan default.
- Enhancing the accuracy of credit scoring models by incorporating diverse data sources and advanced algorithms.
Application processing:
- Automating data entry and verification tasks to speed up the loan application process.
- Validating and cross-referencing application data against external databases to ensure accuracy.
- Streamlining the documentation process by automatically generating and categorizing required documents.
Fraud detection:
- Monitoring loan applications for predefined patterns or anomalies that indicate potential fraud.
- Flagging suspicious activities based on advanced machine learning models.
- Automating the review of flagged applications to ensure thorough and timely investigation.
Regulatory compliance:
- Automating compliance checks to ensure adherence to regulatory requirements.
- Monitoring loan portfolios for compliance with internal policies and external regulations.
- Generating compliance reports and documentation to facilitate regulatory audits.
Customer engagement:
- Offering 24/7 virtual assistance to handle customer inquiries and support requests.
- Automating routine communication tasks such as loan status updates and follow-ups.
- Analyzing customer interactions to provide personalized responses and recommendations.
Portfolio management:
- Analyzing loan portfolio performance to identify trends and potential risks.
- Recommending portfolio adjustments based on real-time data and predictive analytics.
- Automating portfolio rebalancing actions to maintain optimal risk levels.
Marketing and customer segmentation:
- Generating personalized marketing communications based on borrower data and preferences.
- Assisting with content creation for websites, social media, and marketing campaigns.
- Segmenting borrowers based on predefined criteria to target specific customer groups effectively.
Process automation:
- Automating repetitive tasks such as data entry, report generation, and document management.
- Streamlining the loan approval process by automating decision-making workflows.
- Enhancing operational efficiency by reducing manual intervention and errors.
AI agents/copilots not only increase the efficiency of operational processes but also significantly enhance the quality of customer service and strategic decision-making. By integrating these advanced AI solutions into their existing infrastructure, financial institutions can achieve a significant competitive advantage, navigating the complex financial landscape with innovative, efficient, and reliable AI-driven tools and strategies.
Launch your project with LeewayHertz!
Enhance your underwriting processes with AI integration. Collaborate with LeewayHertz for custom solutions, ensuring heightened efficiency and accuracy.
The operational advantages of AI in loan underwriting
The integration of AI in loan underwriting processes brings forth a multitude of benefits, redefining the efficiency, accuracy, and overall effectiveness of the lending industry:
Increased efficiency and speed: AI introduces automation to time-consuming underwriting tasks, significantly expediting the decision-making process. Real-time data analysis capabilities ensure prompt responses to borrower applications, contributing to a more agile and efficient lending workflow.
Improved accuracy and risk assessment: AI-powered models leverage extensive datasets to discern patterns and assess risk factors, resulting in heightened accuracy in predicting creditworthiness. This enhanced precision enables financial institutions to make well-informed lending decisions, minimizing the likelihood of defaults.
Personalized decision-making: The customization capabilities of AI in underwriting empower lenders to tailor decisions based on individual borrower profiles. This personalization extends to interest rates and loan terms, optimizing the lending experience for a diverse clientele.
Cost reduction: Through the automation of manual tasks, AI significantly reduces operational costs associated with underwriting processes. This efficiency in resource utilization leads to a more cost-effective and sustainable lending operation.
Adaptability to dynamic conditions: AI’s real-time adaptability to changing economic conditions ensures that underwriting models remain responsive to evolving market trends. The continuous learning mechanisms embedded in AI contribute to a dynamic and agile approach in the face of economic fluctuations.
Enhanced fraud detection: AI employs sophisticated algorithms to identify anomalies and detect potential fraud within loan applications. This heightened capability in fraud prevention contributes to a more secure lending environment, protecting both financial institutions and borrowers.
Streamlined document processing: Leveraging Natural Language Processing (NLP) and Computer Vision, AI streamlines the analysis of unstructured data and documents in loan applications. This not only accelerates document processing but also enhances accuracy, reducing reliance on manual efforts.
Increased objectivity and consistency: AI’s rule-based operation reduces the impact of human bias, fostering greater objectivity in lending decisions. Consistent application of predefined algorithms ensures fairness throughout the decision-making process, promoting a more impartial approach.
Scalability: AI-driven systems demonstrate scalability by efficiently managing large volumes of data and applications. This scalability is particularly valuable for financial institutions dealing with expanding loan portfolios, ensuring operational efficiency as the business grows.
Data-driven insights: AI’s data analytics capabilities generate actionable insights, empowering lenders with valuable information for strategic decision-making. This data-driven intelligence aids financial institutions in identifying trends, refining underwriting strategies, and staying ahead in a competitive market.
Enhanced customer experience: Faster processing times and personalized offerings contribute to an improved customer experience in the lending process. Transparent and efficient underwriting instills trust among borrowers, fostering positive relationships between financial institutions and their clientele.
Regulatory compliance: AI systems can be designed to ensure compliance with regulatory standards, reducing the risk of errors. Automated processes enhance precision in adhering to legal requirements, mitigating regulatory challenges faced by financial institutions.
The incorporation of AI into loan underwriting not only addresses the challenges of traditional methods but also introduces a range of benefits that collectively enhance the effectiveness and customer satisfaction within the lending industry.
Launch your project with LeewayHertz!
Enhance your underwriting processes with AI integration. Collaborate with LeewayHertz for custom solutions, ensuring heightened efficiency and accuracy.
The future of AI in loan underwriting
The future of AI in loan underwriting holds exciting prospects as technology continues to evolve and financial institutions embrace innovative solutions. Here are key aspects shaping the future landscape:
Expanded use of alternative data: Financial institutions will increasingly leverage alternative data sources beyond traditional credit history. This may include social media activity, online behavior, and other non-traditional indicators, providing a more comprehensive view of an applicant’s financial behavior.
Continuous regulatory adaptation: Regulatory frameworks will adapt to address the distinct challenges introduced by AI in loan underwriting. Governments and regulatory bodies will collaborate to establish guidelines that strike a balance between harnessing the benefits of AI and safeguarding consumer rights, privacy, and equitable lending practices.
Increased focus on education and awareness: With the increasing reliance on AI, there will be a concerted effort to educate stakeholders, including borrowers, about how AI is used in loan underwriting. Transparent communication and awareness programs will be crucial for building trust in AI-driven financial processes.
Innovations in explainable AI: The development of more sophisticated explainability techniques will address the challenge of understanding complex AI models. This will be crucial in gaining trust from both regulatory bodies and the general public.
Cross-industry collaboration: Financial institutions, technology companies, and regulatory bodies will collaborate to share best practices and address common challenges associated with AI in loan underwriting. This collaborative approach will foster a more robust and ethical implementation of AI technologies.
The future of AI loan underwriting will be characterized by continued innovation, increased transparency, and a careful balance between automation and human expertise. As technology advances and regulatory frameworks adjust, AI will become a pivotal force in shaping a lending ecosystem that is more efficient, personalized, and responsible.
Endnote
The integration of AI in loan underwriting marks a paradigm shift in the financial sector, offering a myriad of benefits. AI’s data analysis capabilities allow for a more comprehensive and nuanced evaluation of borrowers, enabling lenders to make informed decisions with increased speed and accuracy. The automation of routine tasks expedites the underwriting process, reducing manual efforts and allowing financial institutions to allocate resources more efficiently. Moreover, AI-driven algorithms can assess risk factors in real-time, adapting to dynamic economic conditions and enhancing the precision of risk assessments. This not only streamlines the lending process but also contributes to more personalized and fair outcomes. The predictive power of AI models aids in identifying patterns and trends, optimizing decision-making and ultimately improving the overall efficiency of loan underwriting. While challenges exist, the transformative impact of AI in this domain underscores its potential to redefine and elevate the lending landscape, fostering innovation, inclusivity, and a more responsive financial ecosystem.
Elevate your underwriting capabilities by integrating AI. Partner with LeewayHertz for custom solutions that will redefine your loan underwriting processes, driving efficiency, precision, and a competitive edge for your business.
Start a conversation by filling the form
All information will be kept confidential.