AI in financial modeling: Bridging the gap between data and decisions
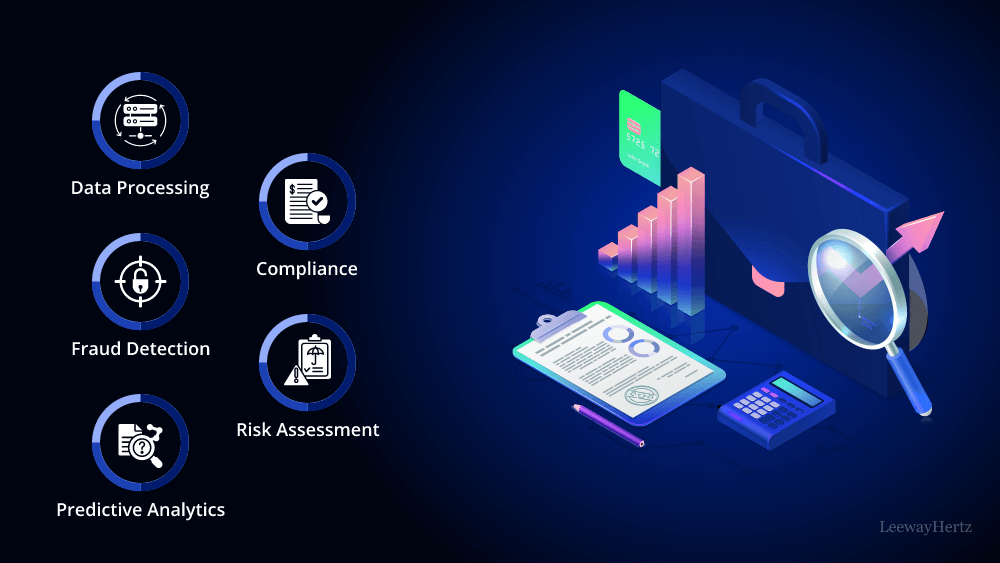
The financial industry is a high-risk industry where every decision can lead to a make-or-break moment. In such a risky sector, the value of accurate and agile financial modeling cannot be overstated. Say a global investment firm is considering a multi-million-dollar acquisition. To make an informed decision, they need to dissect mountains of financial data, assess risks, and predict future performance with utmost precision. One rash decision in such a situation can result in the business losing millions of dollars project. This is where financial modeling steps into the spotlight.
Financial modeling is the cornerstone of strategic decision-making in finance. It serves as the guiding tool for investment decisions, forecasting cash flows and uncovering opportunities in the dynamic financial business setting. However, traditional financial modeling, such as handling massive datasets and adapting to dynamic market conditions, is time-consuming and prone to human errors. AI steps in as a game-changer in such a scenario, altering how financial modeling works and making decision-making in finance even better.
This article explores the role of AI in financial modeling, its benefits in the private equity and investment sector, and more.
- What is financial modeling?
- What is a financial model used for?
- Challenges in traditional financial modeling and how AI addresses them
- Applications of AI in financial modeling
- Elements and components of a financial model
- Who builds financial models?
- How does AI for financial modeling benefit the private equity and investment sector?
What is financial modeling?
Financial modeling is the process of creating a mathematical representation or a simulation of a financial situation or a business using various financial data, variables, and assumptions. It’s a crucial financial and investment analysis tool used for decision-making, forecasting, and planning. Financial models, in short, help predict the future financial performance of a company by merging accounting, business metrics and finance. They can be applied to various scenarios, including valuing a company, projecting future financial performance, evaluating investment opportunities, and assessing risk.
Depending on the complexity of the model, its intended use, and the modeler’s preferences, many approaches and tools are frequently used to depict financial models. One of the most common tools for developing financial models is spreadsheet software like Microsoft Excel. Worksheets in Excel may contain cells that can hold values, formulae, and functions. Excel’s built-in financial modeling functionality frequently consists of linked spreadsheets that each represent a particular component of the model, such as the income statement, balance sheet, or cash flow statement.
What is a financial model used for?
Financial models are used for a wide range of purposes in the world of finance, business, and investment. Here are some applications of financial models:
- Valuation: Financial models are used to estimate the value of a company, an asset, or an investment. Common valuation techniques include discounted cash flow (DCF) analysis, comparable company analysis (CCA), and precedent transactions analysis.
- Investment analysis: Investors use financial models to assess the potential returns and risks associated with different investment opportunities, such as stocks, bonds, real estate, or startups.
- Business planning: Companies use financial models to plan for the future, set financial goals, and make informed decisions about budgets, capital allocation, and resource allocation.
- Mergers and acquisitions (M&A): Financial models play a crucial role in M&A transactions by helping buyers and sellers evaluate the financial implications of a deal, determine a fair purchase price, and assess the potential synergies.
- Project finance: Financial models are used to evaluate the feasibility and profitability of large-scale projects, such as infrastructure development, by estimating future cash flows, debt servicing, and return on investment.
- Risk management: Financial models are used to assess and manage financial risks, such as interest rate risk, currency risk, and credit risk. Monte Carlo simulations are often employed for risk analysis.
- Budgeting and forecasting: Companies use financial models to create budgets and forecasts, enabling them to plan for revenue, expenses, and cash flow in the coming months or years.
- Startup valuation: Startups and early-stage companies use financial models to attract investors, secure funding, and demonstrate their growth potential.
- Real estate analysis: Real estate professionals use financial models to evaluate the financial viability of property investments, including estimating property values, rental income, and return on investment.
- Loan and credit analysis: Financial institutions use models to assess the creditworthiness of borrowers and determine the terms of loans and credit lines.
- Portfolio management: Investment managers use financial models to construct and manage investment portfolios, optimizing asset allocation and risk-adjusted returns.
- Financial reporting: Publicly traded companies use financial models to prepare financial statements, such as income statements, balance sheets, and cash flow statements, for regulatory compliance and shareholder reporting.
- Sensitivity analysis: Financial models are used to perform sensitivity analysis, which involves testing the impact of different variables and assumptions on financial outcomes to assess risk and make informed decisions.
- Capital structuring: Financial models help in optimizing a company’s capital structure, analyzing various combinations of debt, equity, and other financing sources to achieve the most cost-effective and sustainable capital mix.
- Tax planning: Companies use financial models to analyze the tax implications of business decisions and strategies, aiding in efficient tax planning and compliance.
- Dividend policy analysis: Financial models assist in determining an appropriate dividend policy based on the company’s earnings, cash flow, and investment needs.
- Operational optimization: For operational decisions like facility expansions, product line extensions, or other strategic initiatives, financial models can simulate the financial impact of these choices.
- Strategic planning and analysis: Financial models are used for long-term strategic planning, helping companies to evaluate potential pathways for growth and expansion.
- Economic and market analysis: In broader terms, financial models are used to analyze economic trends and market conditions, aiding in macroeconomic forecasting and market analysis.
- Insurance underwriting: In the insurance industry, financial models are used for underwriting purposes, assessing the risks and potential costs associated with insurance policies.
- Energy and commodity market analysis: In sectors like energy and commodities, financial models are employed to predict market movements and pricing, crucial for trading and investment decisions.
- Supply chain management: Financial models can be used to optimize supply chain operations by analyzing costs, revenues, and logistical efficiencies.
- Pension fund management: Financial models assist in managing pension funds, ensuring sufficient funds are available to meet future liabilities.
Elevate Your Financial Models with AI Precision
Challenges in traditional financial modeling and how AI addresses them
AI can address several challenges in traditional financial modeling, offering solutions that improve accuracy, efficiency, and decision-making. Here are some key challenges and how AI can help overcome them:
Handling large and complex data sets
- Challenge: Traditional financial models struggle to process and analyze vast amounts of data quickly and accurately.
- AI solution: AI algorithms can efficiently process large and complex data sets, extracting meaningful insights from structured and unstructured data sources. Machine learning models can identify hidden patterns and relationships that may be challenging for humans to discern.
Manual data entry and error-prone processes
- Challenge: Manual data entry can introduce errors into financial models, leading to inaccurate results and decisions.
- AI solution: AI automates data gathering, cleansing, and entry, reducing the risk of errors. Natural Language Processing (NLP) can extract information from documents and reports, eliminating the need for manual data input.
Time-consuming forecasting and analysis
- Challenge: Traditional financial modeling often involves time-consuming tasks like data analysis, forecasting, and scenario testing.
- AI solution: AI accelerates these processes. Machine learning models can make rapid and accurate predictions, allowing for faster scenario analysis and decision-making.
Limited data sources and traditional metrics
- Challenge: Traditional models may rely on a limited set of financial metrics and data sources, potentially missing valuable information.
- AI solution: AI can incorporate a broader range of data sources, including non-traditional data like social media sentiment and news articles. This enriches the analysis and provides a more comprehensive view of market conditions.
Difficulty in real-time analysis
- Challenge: Traditional models often struggle to provide real-time insights, which are crucial in fast-moving financial markets.
- AI solution: AI-powered algorithms can analyze data in real-time, making it possible to respond quickly to changing market conditions, assess risks, and seize opportunities.
Risk management complexity
- Challenge: Managing financial risks effectively is a complex task due to the dynamic nature of markets.
- AI solution: AI models can identify and assess various types of financial risks, such as credit risk, market risk, and operational risk, in real-time. They provide early warnings and support proactive risk management.
Regulatory compliance and reporting
- Challenge: Complying with complex financial regulations and reporting requirements can be time-consuming and error-prone.
- AI solution: AI automates compliance tasks, ensuring accurate and timely reporting while reducing the risk of regulatory violations.
Lack of personalization
- Challenge: Traditional models may offer one-size-fits-all solutions without considering individual preferences and risk tolerance.
- AI solution: AI-driven robo-advisors provide personalized investment advice and portfolio management based on individual goals and risk profiles, enhancing the customer experience.
Difficulty in portfolio optimization
- Challenge: Constructing well-diversified portfolios manually can be challenging, leading to suboptimal asset allocation.
- AI solution: AI-powered portfolio optimization models use advanced algorithms to construct and manage portfolios that maximize risk-adjusted returns based on individual preferences.
Elevate Your Financial Models with AI Precision
Applications of AI in financial modeling
Data processing and analysis
AI plays a crucial role in enhancing data processing and analysis within financial modeling. It has the capability to rapidly process vast volumes of financial data, including historical records, market data, and news sentiment. Through machine learning algorithms, AI conducts in-depth data analysis, identifying trends, patterns, and anomalies that might not be easily discernible through traditional analysis methods. This comprehensive data analysis significantly enhances the accuracy of financial models, ultimately enabling more informed and data-driven decision-making processes.
Automation of routine tasks
One of the fundamental strengths of AI lies in its ability to automate routine and time-consuming tasks that are inherent in financial modeling. These tasks include data entry, data cleansing, and report generation, among others. By automating these repetitive processes, AI not only reduces the risk of human errors in financial models but also allows finance professionals to allocate their time and expertise to more strategic aspects of financial analysis.
Predictive analytics
AI’s predictive capabilities are a game-changer in financial modeling. Machine learning models have the capacity to make accurate predictions regarding financial market movements, stock prices, and economic indicators. This enables finance professionals to have more reliable forecasts at their disposal, which, in turn, supports better-informed investment decisions and financial planning.
Natural Language Processing (NLP)
A survey conducted by Nvidia, encompassing finance-based firms in America and Europe with over 200 respondents, highlighted that natural language processing and large language models represented the leading AI use cases, accounting for 26% of the responses. NLP is thus, undoubtedly, emerging as a driving force of innovation among finance-oriented businesses. NLP technology empowers AI to read and comprehend textual data, including financial news, reports, and earnings call transcripts. By extracting sentiment and key information from text-based sources, AI can assess market sentiment and its potential impact on investments. This ability to analyze and incorporate textual data into financial models adds valuable information for decision-makers.
Risk assessment and management
AI’s real-time risk assessment capabilities are indispensable in financial modeling. AI models can swiftly identify and assess various types of financial risks, such as credit risk, market risk, and operational risk. This real-time risk assessment enables proactive risk management and timely decision-making to mitigate potential losses.
Scenario analysis and sensitivity analysis
AI streamlines scenario and sensitivity analysis by automating the process of testing various scenarios. AI-driven models can rapidly adjust key variables in financial models to assess potential outcomes under different conditions. This automation simplifies the evaluation of the impact of changes in specific variables on financial results, allowing for a more comprehensive analysis.
Fraud detection and security
AI plays a critical role in enhancing security within financial modeling. AI-driven fraud detection systems analyze transaction data to identify suspicious patterns and potential fraudulent activities. These systems bolster security measures, safeguarding financial institutions and their clients from fraudulent activities.
Compliance and regulatory reporting
AI-driven automation streamlines compliance tasks, ensuring adherence to complex financial regulations and reporting requirements. By automating these tasks, AI reduces the risk of regulatory violations and associated penalties, thus ensuring regulatory compliance in a more efficient manner.
Personalized financial advice
AI-driven robo-advisors provide personalized investment advice and portfolio management based on individual goals and risk profiles. These solutions offer cost-effective alternatives to traditional wealth management services, making personalized financial advice more accessible to a broader range of investors.
Elements and components of a financial model
Key elements and components of a financial model may include:
- Historical data: Financial models often start with an analysis of historical financial data, such as income statements, balance sheets, and cash flow statements, to understand past performance and trends.
- Assumptions: Financial models rely on a set of assumptions about future variables and conditions. These assumptions can cover aspects like revenue growth rates, operating expenses, interest rates, inflation, and market conditions.
- Financial statements: The model typically includes projected financial statements, such as income statements, cash flow statements, and statements of changes in equity, which provide a comprehensive view of the financial performance and position of the entity being analyzed.
- Revenue projections: Financial models often project future revenues based on factors like sales growth, pricing strategies, and market demand.
- Expense projections: They also estimate future expenses, including operating costs, taxes, interest payments, and capital expenditures.
- Cash flow analysis: Financial models calculate cash flows to assess the availability of funds for various purposes, such as debt repayment, investment, and dividend distribution.
- Scenario analysis: Financial models may incorporate multiple scenarios to analyze different possible outcomes under various conditions, helping decision-makers plan for different contingencies.
- Risk assessment: Advanced financial models may include risk assessment tools like Monte Carlo simulations to quantify and manage financial risks.
- Graphs and charts: Visual aids like graphs and charts can make it easier to interpret and communicate the results of the financial model.
- Valuation metrics: Financial models often include valuation metrics like Net Present Value (NPV), Internal Rate of Return (IRR), and payback period. These metrics are crucial for assessing the profitability and feasibility of investments.
Elevate Your Financial Models with AI Precision
Who builds financial models?
Financial models are typically built by professionals with expertise in finance, accounting, and related fields. Here are some of the industry-wise roles and individuals who are involved in building financial models:
Investment banking and finance
Financial analysts: They are responsible for creating models for analysis in investment banking, corporate finance, asset management, and consulting.
Investment bankers: Investment bankers create models for valuing companies, mergers, acquisitions, and financial transactions.
Corporate finance
Corporate finance professionals: These professionals build models for budgeting, forecasting, capital allocation, and evaluating investment opportunities within companies.
Financial Planning & Analysis (FP&A) teams: They focus on financial modeling for budgeting and strategic planning.
Private equity
Private equity analysts: They develop complex models for evaluating potential investments, conducting due diligence, and monitoring portfolio companies.
Portfolio managers: Portfolio managers utilize models to optimize asset allocation, assess portfolio performance, and make investment decisions based on risk and return analysis.
Consulting
Management consultants: Build models to support strategic decision-making, including market analysis, cost assessment, and scenario modeling.
Other sectors
Financial planners: They use models to help clients plan for retirement, assess investment strategies, and achieve financial goals.
Real estate analysts: They create models to assess property feasibility, estimate valuations, and evaluate investment returns in real estate development and investment.
Risk managers: As the name suggests, risk managers employ models to assess and mitigate financial risks, such as credit, interest rate, and market risks.
Quantitative analysts (quants): They create mathematical models for trading strategies, risk management, and derivatives pricing.
Government agencies: They use financial models to analyze policy impact, project tax revenues, and plan public infrastructure projects.
Each of these professionals plays a vital role in their respective industries by constructing financial models to aid in decision-making, analysis, and planning.
How does AI for financial modeling benefit the private equity and investment sector?
Improved investment decisions
AI-powered predictive analytics can accurately estimate changes in stock prices, market activity, and economic indicators. Investors may make more intelligent and strategic decisions by using these tools, which can quickly spot trends, patterns, and untapped possibilities. Better investing decisions may result in greater returns, lower risks, and a stronger position in the market by supplementing human judgment with data-driven insights.
Efficient due diligence
One distinguishing characteristic of AI integration in the investing sector is efficiency in the procedures of due diligence. AI speeds up the assessment of possible investments by automating the collection and analysis of financial data, company performance metrics, and regulatory compliance. In addition to saving a lot of time, this automation improves accuracy by lowering the possibility of human error. Additionally, AI is able to continuously monitor news, social media, and other outside influences, offering important updates that might influence investment choices. As a result, effective due diligence enables investors to decide quickly and with the best use of available resources.
Portfolio optimization
Financial models powered by AI take into consideration a wide range of important variables, such as investor risk tolerance, particular investment goals, and market circumstances. Investors may reach a degree of asset allocation precision that was previously unachievable by utilizing the enormous computational power and data analytic capabilities of AI. As a consequence, portfolios are not only tailored to each investor’s specific preferences and aims but also perfectly calibrated to respond to shifting market dynamics. Because of the consequently increased potential for greater returns and risk avoidance, portfolio optimization using AI has become an essential tool for investors looking to optimize their financial strategy in a dynamic and cutthroat investing environment.
Behavioral analysis
AI algorithms discover novel insights from market sentiments and investor behavior by closely examining investor sentiment, keeping track of news trends, and filtering through social media data. Making intelligent investing decisions is made much easier with this data-driven understanding. Investors can proactively modify their strategy by gauging the current market mood and spotting new trends or worries. This helps them to take advantage of possibilities or avoid potential dangers in addition to facilitating prompt actions. In the end, behavioral analysis with AI gives investors a potent tool to gauge the market mood in real-time, improving their capacity to make flexible and knowledgeable investment decisions in today’s quick-paced and data-rich financial scene.
Endnote
The integration of AI into financial modeling represents a transformative leap forward in the domain of finance. AI-powered tools and techniques have redefined the boundaries of what’s possible, offering professionals in finance and investment a powerful set of capabilities for data analysis, predictive modeling, risk management, and decision-making.
AI will play a bigger part in financial modeling as it develops and adapts to the changing financial scene. The advantages of AI, such as improved data processing, regular operations being automated, and personalized insights, enable finance professionals to better manage the complexity of the current financial world.
Looking to leverage AI’s potential in financial modeling? Contact LeewyHertz’s experienced AI developers today for tailored solutions that elevate your financial strategies.
Start a conversation by filling the form
All information will be kept confidential.
Frequently Asked Questions
How does AI enhance financial modeling?
AI enhances financial modeling by processing vast amounts of data rapidly, identifying complex patterns and correlations, and generating more accurate predictions for investment opportunities, risk assessment, and portfolio management.
Can AI be used for risk management in financial modeling?
Yes, AI techniques such as machine learning and deep learning are extensively used for risk management in financial modeling. These technologies help in identifying and assessing different types of risks, including market, credit, operational, and compliance risks, thereby enabling financial institutions to mitigate potential losses and optimize their risk-adjusted returns.
How does AI-powered financial modeling impact investment decisions?
AI-powered financial modeling equips investors with advanced, data-driven insights, significantly enhancing their decision-making capabilities. By integrating AI, investors can rapidly identify lucrative opportunities, respond to market changes, optimize their asset allocations based on predictive analytics, and manage risks more precisely. This leads to more strategic, informed, and timely investment decisions, potentially increasing returns while mitigating risks.
Which types of financial models are most commonly enhanced using AI?
Some examples include credit scoring models for lending institutions, stock price prediction models used by investment firms, and fraud detection systems employed by banks and other financial organizations.
How can LeewayHertz assist with AI in financial modeling?
LeewayHertz offers expertise in developing AI-driven financial modeling solutions customized to the specific needs of financial institutions, investment firms, and fintech startups. Our team specializes in building predictive models, algorithmic trading systems, risk management tools, and automated investment platforms using advanced AI techniques.
What sets LeewayHertz apart as an AI partner for financial modeling?
LeewayHertz distinguishes itself as a leading AI consulting and development company with a proven track record of delivering innovative solutions for financial modeling. Our team of data scientists, AI engineers, and domain experts collaborates closely with clients to understand their business objectives, develop robust AI models, and deploy scalable solutions that drive tangible business outcomes.
How does LeewayHertz ensure data security and compliance in AI-driven financial modeling projects?
LeewayHertz prioritizes data security and compliance with industry regulations in all AI-driven financial modeling projects. We implement robust encryption protocols, access controls, and compliance measures to safeguard sensitive financial data and ensure regulatory compliance throughout the project lifecycle.
What is the process for initiating a financial modeling project with LeewayHertz?
To begin a financial modeling project with LeewayHertz, clients can schedule a consultation with our team to discuss their requirements, objectives, and vision for the project. Our experts will then conduct a thorough analysis of the data and develop a customized AI solution tailored to the client’s specific needs and objectives.
Can LeewayHertz provide ongoing support and maintenance for AI-powered financial modeling solutions?
To begin a financial modeling project with LeewayHertz, clients can schedule a consultation with our team to discuss their requirements, objectives, and vision for the project. Our experts will then conduct a thorough analysis of the data and develop a customized AI solution tailored to the client’s specific needs and objectives.
Insights
Generative AI for enterprises: The architecture, its implementation and implications
By understanding the architecture of generative AI, enterprises can make informed decisions about which models and techniques to use for different use cases.
From diagnosis to treatment: Exploring the applications of generative AI in healthcare
Generative AI in healthcare refers to the application of generative AI techniques and models in various aspects of the healthcare industry.
How to create a generative video model?
Generative video models are machine learning algorithms generating new video data based on patterns learned from training datasets. Explore more about it!