Generative AI in manufacturing: Use cases, architecture, benefits and development
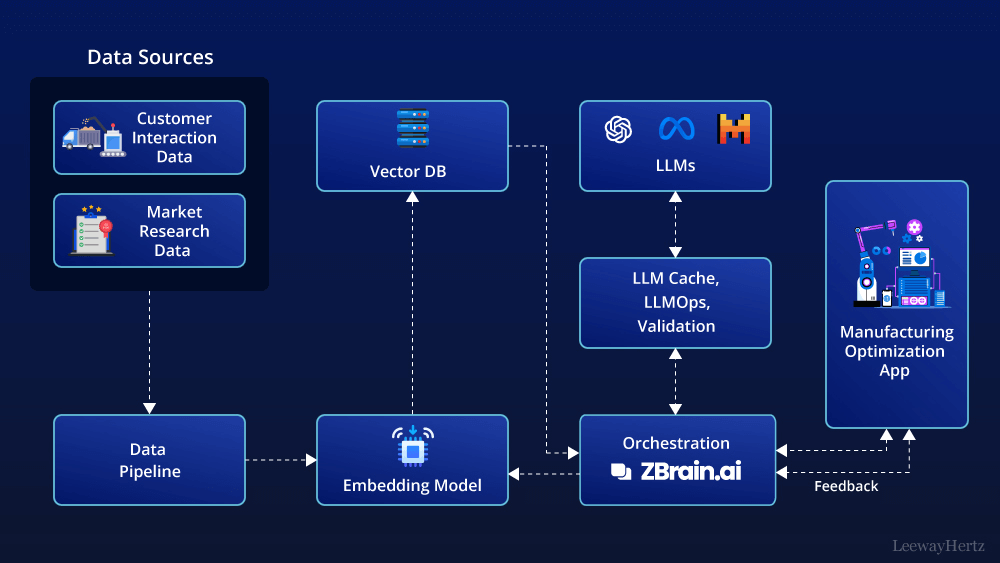
In the rapidly evolving landscape of technology, Artificial Intelligence (AI) has emerged as a driving force behind substantial transformations across diverse sectors. Among these, the manufacturing industry stands out as a prominent beneficiary, capitalizing on the advancements and potential of AI to enhance its processes and unlock new opportunities. Among the various types of AI, generative AI, known for its content creation and enhancement capabilities, is playing a significant and distinct role in shaping the advancement of manufacturing practices. Harnessing vast and diverse data streams – from real-time sensor readings to manual reports – generative AI has emerged as a critical enabler of data-driven decision-making.
As a solution to legacy systems and established management processes that dominate traditional manufacturing, generative AI interprets complex data to refine operational strategies on both the factory floor and the organizational level. Thus, generative AI equips manufacturers with the means to address data quality challenges and realize AI’s full potential in their operations.
However, the pathway to AI integration is not always smooth, especially in industries where effective communication and emotional understanding are paramount. Machines’ inherent shortcomings in interpreting language and emotional cues may hinder AI’s comprehensive adoption in these sectors.
This article aims to guide you through the intriguing field of generative AI in manufacturing. We will explore how it operates, its benefits, and its transformative role in manufacturing.
- What is generative AI?
- How does generative AI for manufacturing work?
- Generative AI use cases in manufacturing
- Data-driven research and development
- Turning raw data into valuable products
- Product design and development
- Enterprise knowledge management
- Production and operations
- Machine automation and optimization
- Quality control
- AI-driven patent management optimization
- Supplier evaluation
- Production planning and scheduling
- Customer acquisition and relationship management
- Customer interactions and support solutions
- Predictive maintenance powered by behavioral analysis
- Automatic product defect detection
- Supply chain and logistics
- Financial management
- Environmental and sustainability practices
- Health and safety compliance
- Corporate governance and internal controls
- How LeewayHertz’s generative AI platform streamlines manufacturing workflows?
- LeewayHertz’s AI development services for manufacturing
- How to implement generative AI in manufacturing workflow?
- Technologies used in generative AI in manufacturing
- Benefits of generative AI in manufacturing
What is generative AI?
As an innovative subset of artificial intelligence, generative AI stands at the forefront of digital transformation with its ability to create unique content across a multitude of formats like text, images, audio, and video. Generative AI platforms such as ChatGPT, DALL-E, and Stable Diffusion, all powered by advanced machine learning models, drive benefits across diverse sectors and functional areas, enhancing the efficiency of marketing initiatives, customer service, and sales strategies.
Generative AI hinges on the capabilities of foundational AI models. These robust, versatile models can undertake various tasks such as summarization, question- answering, and classification, among others. What sets these models apart is their ability to adapt to specific applications with very little training data.
Generative AI functions based on the principles of machine learning. The AI model is trained on a dataset that contains content created by humans. It analyzes this dataset to identify underlying patterns and structures. Once trained, the model can generate new content by leveraging the knowledge it gained from the patterns in the training dataset.
The conventional method for training a generative AI model is through supervised learning. Here, the model is supplied with a dataset of human-generated content and associated labels. It learns to generate content that parallels the provided human-generated content and carries the same labels.
A distinctive advantage of generative AI is its democratizing influence on AI development. Traditionally, the creation and deployment of AI solutions were a preserve of data scientists. However, the advent of generative AI has disrupted this exclusivity, enabling the wider community to develop AI solutions catering to businesses and other organizations.
With its inherent ability to accelerate efficiency in knowledge-based work, generative AI offers an opportunity to economize on time and resources, allowing professionals to dedicate their focus to strategic initiatives.
Generative AI is distinctive from traditional AI, particularly because it emphasizes user-specific content creation. This shift in approach underscores generative AI’s transformative potential in the broader technological ecosystem.
How does generative AI for manufacturing work?
Generative AI transforms manufacturing operations by using advanced analytics, machine learning models, and real-time monitoring to optimize production lines, enhance product quality, and reduce operational costs. GenAI technologies help with predictive maintenance, quality control, and supply chain optimization by utilizing advanced large language models (LLMs) and connecting them with the manufacturing firm’s comprehensive data sources.
Let us explore how generative AI streamlines manufacturing processes through this structured architecture of a GenAI-powered manufacturing system. This system integrates multiple components to enhance manufacturing operations and functions as follows:
Data sources: Effective manufacturing optimization relies on comprehensive and diverse data inputs, including:
- Machine telematics: Real-time data from machinery sensors that monitor operational conditions, performance metrics, and potential faults.
- Quality control data: Information from inspection systems and quality checks to identify defects and meet product standards.
- Supply chain information: Data on inventory levels, supplier delivery times, and logistics to optimize supply chain operations.
- Production schedules: Detailed production plans and historical performance data to enhance forecasting accuracy and resource allocation.
- Environmental data: Information on factory environmental conditions, such as temperature and humidity.
- Employee input and feedback: Data from operators and floor managers regarding the production process, machine operations, and potential areas for improvement.
Data pipelines: The data from these diverse sources are routed through sophisticated data pipelines that handle their ingestion, cleaning, and structuring, preparing the data for detailed analysis.
Embedding model: The processed data is segmented into chunks and fed into an embedding model. This model converts textual data into numerical representations called vectors, enabling AI models to comprehend it effectively. Popular models from providers like OpenAI, Google, and Cohere are utilized for this purpose.
Vector database: The generated vectors are stored in a vector database such as Pinecone, Weaviate, or PGvector, enabling efficient and rapid querying. Vector database efficiently manages the storage, comparison, and retrieval of potentially billions of embeddings (i.e., vectors).
APIs and plugins: APIs and plugins like Serp, Zapier, and Wolfram play a key role by connecting different components and enabling additional functionalities, such as accessing extra data, connecting with external tools or platforms or performing specific tasks with ease.
Orchestration layer: The orchestrating layer is critical in managing the workflow. ZBrain is an example of this layer that simplifies prompt chaining, manages interactions with external APIs by determining when API calls are required, retrieves contextual data from vector databases, and maintains memory across multiple LLM calls. Ultimately, this layer generates a prompt or series of prompts that are submitted to a language model for processing. The role of this layer is to orchestrate the flow of data and tasks, ensuring seamless coordination across all components of the manufacturing architecture.
Query execution: The data retrieval and generation process begin when the user submits a query to the manufacturing optimization app. These queries relate to machine efficiency, production quality, or inventory levels.
LLM processing: Once received, the app transmits the query to the orchestration layer. This layer retrieves relevant data from the vector database and LLM cache and sends it to the appropriate LLM for processing. The choice of LLM depends on the nature of the query.
Output: The LLM generates an output based on the query and the data it receives. This output can take various forms, such as predictive maintenance schedules, quality control adjustments, and optimized production strategies.
Manufacturing optimization app: This specialized app delivers AI-generated insights in a simple format, enabling manufacturing executives to make informed decisions quickly and manage their operations more effectively.
Feedback loop: User feedback on the LLM’s output is another important aspect of this architecture. The system incorporates user feedback to continuously improve the accuracy and relevance of the LLM’s outputs.
Agent: AI agents step into this process to address complex problems, interact with the external environment, and enhance learning through post-deployment experiences. They achieve this by employing advanced reasoning/planning, strategic tool utilization, and leveraging memory, recursion, and self-reflection.
LLM cache: To accelerate the AI system’s response time, frequently accessed information is cached using tools like Redis, SQLite, or GPTCache.
Logging/LLMOps: Throughout this process, LLM operations (LLMOps) tools like Weights & Biases, MLflow, Helicone and Prompt Layer help log actions and monitor performance. This ensures the LLMs operate at peak efficiency and evolve consistently through ongoing feedback mechanisms.
Validation: A validation layer is employed to validate the LLM’s output. This is achieved through tools like Guardrails, Guidance, Rebuff, and LMQL to ensure the accuracy and reliability of the information provided.
LLM APIs and hosting: LLM APIs and hosting platforms are essential for executing manufacturing tasks and hosting the application. Depending on the requirements, developers can select from LLM APIs offered by firms like OpenAI and Anthropic or opt for open-source models. Similarly, they can choose hosting platforms from cloud providers like AWS, GCP, Azure, and Coreweave or opt for opinionated clouds like Databricks, Mosaic, and Anyscale. The choice of LLM APIs and cloud hosting platforms depends on the project’s needs.
This detailed flow provides a comprehensive view of how generative AI enhances manufacturing by leveraging sophisticated data sources and technological tools. By automating key manufacturing tasks, AI boosts operational efficiency and supports a deeper analysis of production processes, ultimately leading to smarter, faster, and more reliable manufacturing outcomes.
Optimize Your Operations With AI Agents
Optimize your workflows with ZBrain AI agents that automate tasks and empower smarter, data-driven decisions.
Generative AI use cases in manufacturing
The rapid progression of artificial intelligence is impacting a range of industries. Here are a few generative AI use cases in manufacturing:
Data-driven research and development
Generative AI has emerged as a powerful tool for facilitating data-driven research and development (R&D) in the manufacturing industry. It provides innovative methods for understanding customer needs, analyzing product usage, and spotting emerging market trends.
The process begins with robust data collection from a variety of sources, such as customer feedback, product usage data, market research data, and industry trends. This data could be sourced from product sensors, customer surveys, social media, sales data, and other relevant channels. Once gathered, the data undergoes preprocessing to eliminate noise and extraneous information. Key patterns and characteristics are then extracted from this data, forming features that might encompass customer preferences, product performance parameters, and market demand patterns.
Generative AI models then execute descriptive and diagnostic analytics on the feature set. Descriptive analytics offer a summarized view of the dataset’s primary characteristics, providing an understanding of past events. In contrast, diagnostic analytics delve deeper into the data to decipher why those events occurred.
A significant capability of generative AI lies in its ability to detect bottlenecks in the manufacturing process. Various methods, including discrete, purely data-driven, and real-time detection, are utilized for this purpose. Manufacturers can optimize operations and increase productivity by identifying and addressing these bottlenecks.
Subsequently, generative AI models are trained on preprocessed data to predict customer needs, product usage patterns, and future market trends. These predictions can guide the development of new products or improvements to existing ones, enabling manufacturers to keep pace with market trends and meet customer needs more effectively.
An essential aspect of generative AI models is their capacity for continuous learning and improvement. As new data is constantly incorporated, the models adapt to changing customer needs and market trends, ensuring that the insights and predictions remain accurate and relevant.
The integration of generative AI into R&D processes equips manufacturers to make more informed decisions, develop superior products, and stay competitive. With its capacity to analyze extensive data and generate actionable insights, generative AI significantly enhances the efficiency and effectiveness of R&D in the manufacturing industry.
Turning raw data into valuable products
Generative AI is enhancing manufacturing by optimizing processes and informing product design through data analysis. This is accomplished through a synergistic combination of data analysis, predictive modeling, and continuous learning.
The process commences with collecting raw data from various sources, such as sensor data from machinery, customer feedback, sales data, and market trends. This raw data undergoes a thorough cleaning and preprocessing stage, which includes noise or outlier removal, data normalization, and managing any missing values, resulting in a clean, structured dataset ready for analysis.
Subsequently, feature extraction identifies significant patterns or characteristics in the data, serving as inputs for the Generative AI model. These patterns, often referred to as features, are essential in making accurate predictions or generating valuable insights.
With features in place, a generative AI model is trained on this data. The model discerns patterns and relationships within the data, which equips it to generate new content or make predictions based on these learned patterns.
A key benefit of generative AI lies in its ability to generate new services using the derived data insights. For instance, AI can leverage analyzed customer usage data and feedback to create personalized customer experiences, recommend products, or automate customer service.
When it comes to production control, generative AI can significantly optimize manufacturing processes by analyzing operational data. This optimization could extend to improving resource allocation, scheduling, and inventory management in a continuous flow manufacturing process, custom manufacturing, or a fixed manufacturing environment.
Generative AI models are designed to learn and adapt continually. As more data is accumulated, the models refine their predictions and generate increasingly accurate and valuable outputs.
Generative AI, by transforming raw data into valuable products and services, offers the manufacturing industry a potent tool that can enrich customer service, optimize production control, elevate quality control, and contribute significantly to the overall efficiency and productivity of the manufacturing process.
Product design and development
Generative AI is transforming product design and development in manufacturing. The process begins with engineers inputting specific goals for a product, including performance requirements, cost constraints, and other limitations. Using these parameters, the AI generates numerous design options, learning and improving with each iteration. The AI generates numerous design options using these parameters while learning and improving with each iteration. The benefits of this technology are significant: it dramatically speeds up the design process, produces more creative and efficient designs, and often results in better-performing products that consume less resources and are structurally stronger. Additionally, the reduced design time and decreased material waste lead to substantial cost savings. By leveraging generative AI, companies can create superior products more quickly and efficiently, giving them a competitive advantage in their respective industries. This technology not only enhances current design practices but also opens up new frontiers for product innovation and manufacturing efficiency.
Enterprise knowledge management
The manufacturing sector’s expansion often results from mergers and acquisitions, leading to a siloed infrastructure of systems and processes. This can make locating the appropriate and relevant information a considerable challenge for many employees, leading to considerable time wastage and reduced productivity. Implementing an enterprise knowledge bot powered by a sophisticated language model like ChatGPT can be a game-changer in addressing this issue.
Such a bot can drastically reduce employees’ time seeking information, resulting in a notable boost in productivity. Generative AI applications like ChatGPT excel in understanding and generating human-like text, enabling them to sift through vast amounts of data and extract precise and relevant information based on user queries. They can be trained on a wide range of data, from product manuals to internal process guidelines, which makes them a one-stop source for enterprise-wide information.
Additionally, these AI models can adapt and learn from new information, ensuring that the knowledge they provide remains up-to-date and relevant and helping to maintain a continual learning process within the enterprise.
The functionality of such an AI bot can also be extended to discern business rules from legacy applications, even in cases where documentation may be lacking. This capacity to extract and interpret knowledge from diverse sources can help organizations effectively manage and leverage their accumulated knowledge, leading to improved decision-making processes, streamlined operations, and enhanced productivity while fostering an environment of continuous learning and improvement.
Production and operations
Generative AI plays a crucial role in enhancing production and operations in manufacturing. By leveraging advanced algorithms and real-time data analysis, generative AI assists in managing day-to-day operations, ensuring a seamless and efficient production workflow. Predictive analytics identifies patterns and potential bottlenecks, allowing for proactive problem-solving and minimizing disruptions. Generative AI optimizes production efficiency by continuously analyzing data to fine-tune processes, improve resource allocation, and enhance overall output. It enables manufacturers to dynamically adjust to changing demands and market conditions, maximizing capacity utilization. Additionally, by providing actionable insights and recommendations, generative AI empowers decision-makers to make informed choices, leading to sustained improvements in manufacturing performance and competitiveness.
Machine automation and optimization
Generative AI is transforming manufacturing automation and optimization through data-driven insights and predictive capabilities. The process begins with extensive data collection from sensors and IoT devices on machinery, providing crucial information about various aspects of manufacturing operations.
A key application is in calculating and optimizing Overall Equipment Effectiveness (OEE), a critical measure of manufacturing efficiency. Generative AI models analyze real-time data to compute OEE metrics – availability, performance, and quality – and detect anomalies that might affect productivity.
Beyond OEE calculation, these AI models offer several benefits:
- Predictive maintenance: By analyzing equipment data, AI can predict potential failures, allowing for proactive maintenance and minimizing downtime.
- Process optimization: AI identifies inefficiencies in production processes, pinpoints bottlenecks, and suggests improvements to enhance overall productivity.
- Real-time monitoring: Continuous analysis of operational data allows for immediate detection of issues and rapid response to maintain optimal performance.
- Adaptive learning: The AI system continuously learns from new data, improving its predictions and recommendations over time.
By leveraging generative AI in this way, manufacturers can significantly improve operational efficiency, reduce costs, and maintain higher quality standards in their production processes.
Quality control
Generative AI is pivotal in maintaining product quality and overseeing production within the manufacturing industry. Traditional methods of identifying internal equipment malfunctions often prove challenging, even for experts, given the complexity of diagnosing issues solely based on functional observation. However, integrating AI and Machine Learning (ML) technologies presents a game-changing solution.
Generative AI systems can swiftly pinpoint even the most minute equipment defects. By leveraging these systems, the detection of potential issues becomes proactive rather than reactive, significantly reducing downtime and cost related to sudden equipment failure.
The sensors attached to crucial machinery gather data throughout the manufacturing process. Advanced algorithms then analyze this data, identifying any aberrations and patterns indicative of impending issues. By doing so, generative AI allows for real-time intervention, proactively averting problems and ensuring a smooth and efficient production flow, offering significant operational benefits for the manufacturing industry.
Generative AI systems detect potential errors and alert stakeholders in real time, mitigating large-scale production issues before they escalate. These AI-powered systems actively self-monitor and instantly report production defects.
AI-driven patent management optimization
In the dynamic manufacturing sector, protecting Intellectual Property (IP) is vital, but conventional patent management faces obstacles such as intricate portfolios, time-intensive analysis, legal complexities, and hurdles in strategic decision-making. Generative AI addresses these challenges by automating analysis, simplifying legal navigation, and fortifying intellectual property protection. This innovation ensures manufacturing firms can efficiently navigate evolving laws, overcome complexities, and bolster their IP portfolios. Generative AI in patent management provides a strategic edge, enabling companies to stay ahead in the competitive landscape while ensuring robust protection of their innovations.
View: Patent Portfolio Analysis Flow
Supplier evaluation
In the realm of manufacturing, making informed decisions about suppliers is critical for business success. The process, however, is often hindered by the complexity of evaluating numerous factors and analyzing vast amounts of data, leading to a time-consuming ordeal. Generative AI streamlines supplier evaluation and selection by leveraging advanced algorithms and data analytics. It simplifies the decision-making process, enabling manufacturers to swiftly and accurately assess potential suppliers. This saves valuable time and ensures that businesses make strategic choices, fostering efficiency and resilience in the supply chain.
View: Supplier Evaluation Flow
Production planning and scheduling
In manufacturing, challenges like inaccurate forecasting, suboptimal resource allocation, and inefficient inventory management can result in downtime, missed deadlines, and high carrying costs. Traditional production planning methods often fall short of meeting dynamic market demands. Generative AI brings agility and accuracy, optimizing resource allocation and inventory management. The result is increased efficiency and precision, mitigating downtime risks and reducing costs. Generative AI empowers manufacturing businesses to align seamlessly with market dynamics, ensuring timely deliveries and improved overall operational outcomes.
View: Capacity Evaluation Flow
Customer acquisition and relationship management
Generative AI significantly enhances customer acquisition and relationship management by crafting innovative marketing strategies. By analyzing vast datasets, AI algorithms identify patterns in consumer behavior, allowing businesses to target and attract new clients with personalized and highly effective campaigns. In the realm of customer relationship management (CRM), generative AI systems play a pivotal role by implementing sophisticated CRM tools. These tools facilitate efficient communication through personalized messaging, tailored recommendations, and automated interactions, thereby strengthening the bond between businesses and their customers. AI-driven CRM systems not only streamline processes but also contribute to a deeper understanding of individual customer preferences, enabling businesses to deliver more personalized and engaging services.
Customer interactions and support solutions
Generative AI is transforming customer interactions and support in the manufacturing sector by providing intelligent, scalable, and personalized solutions. This technology offers several key benefits:
- 24/7 virtual representation: AI systems can address inquiries and provide product information around the clock, improving client experiences without human intervention.
- Resource optimization: By handling routine queries, AI frees up human agents for more complex tasks, enhancing overall operational efficiency.
- Data-driven insights: AI rapidly analyzes vast amounts of customer data, including usage patterns, feedback, and preferences, to customize offerings and preemptively address potential issues.
- Continuous learning: The system improves with each interaction, leading to increasingly personalized and efficient customer service.
- Scalability: AI can handle surges in inquiries during product launches or peak periods without additional staffing.
By implementing generative AI in customer support, manufacturers can significantly improve service quality, operational efficiency, and customer satisfaction while effectively managing resources and scaling operations.
Predictive maintenance powered by behavioral analysis
Generative AI significantly enhances predictive maintenance in the manufacturing industry by applying advanced behavioral analysis. This process begins with extensive data collection through numerous sensors installed on the machinery. These sensors continuously monitor operational parameters such as temperature, vibration, pressure, and acoustics, providing a wealth of data about the machine’s operation and condition. This raw data then undergoes a feature extraction process, where vital patterns and characteristics that offer meaningful insights into the machine’s operation and health are identified. A generative model, such as a Generative Adversarial Network (GAN) or a Variational Autoencoder (VAE), is then trained on these features. The training allows the model to understand the normal operational behavior of the equipment, setting a benchmark for detecting anomalies.
Once trained, the model continuously scrutinizes real-time operational data and conducts a behavioral analysis, contrasting the real-time data with the learned ‘normal’ behavior. Any significant deviation indicates potential equipment malfunction, highlighting the need for maintenance.
The ability to detect possible malfunctions before they result in equipment failure enables the system to schedule predictive maintenance. Rather than adhering to a standard maintenance schedule, this approach schedules maintenance based on predicted equipment failures, leading to improved operational efficiency and optimized use of maintenance resources.
Moreover, as the system accumulates data over time, the generative model constantly learns and refines its predictions. This ongoing learning enhances the accuracy of predictive maintenance capabilities.
Through its behavioral analysis and continual learning, generative AI presents an innovative and efficient approach to predictive maintenance in manufacturing. By enabling the fine-tuning of production plans and the customization of component-wise maintenance schedules, it not only enhances productivity but also increases process transparency and product availability by significantly transforming the overall efficiency and effectiveness of the manufacturing process.
Automatic product defect detection
Automatic product defect detection, a critical application of generative AI in the manufacturing sector, provides an efficient, data-driven approach to maintaining high-quality standards and reducing manual inspection costs.
This process commences with extensive data collection using various sensors and vision systems that capture high-resolution images or videos of the products at different stages of the manufacturing process. These devices can collect comprehensive data on numerous product characteristics such as size, color, shape, and texture. The collected data is then subjected to preprocessing to eliminate noise and irrelevant information. Subsequently, a process of feature extraction identifies and extracts key characteristics or patterns from the data that can offer meaningful insights about the product’s quality.
View: Defect Analysis Flow
Once these relevant features are identified, a generative AI model, such as a Generative Adversarial Network (GAN) or a Variational Autoencoder (VAE), is trained which enables the model to recognize the ‘normal’ state of the product, providing a benchmark for detecting deviations.
The trained model then continuously monitors real-time production data, with any significant deviation from the ‘normal’ data signifying a potential defect.
In certain cases, the generative AI model can be trained to perform tasks such as Object Character Recognition (OCR) and detect object orientation. This ability aids in detecting defects in products that carry specific labels, markings, or have a required orientation.
The model can also detect deformities by learning what constitutes a ‘deformed’ product from the training data. When a defect is detected, the system immediately sends an alert, often with diagnostic information pinpointing the defect’s nature and location, enabling maintenance teams to address and resolve the issue quickly.
Over time, the AI system learns and enhances its predictive accuracy and reliability, continually refining its understanding of ‘normal’ and ‘defective’ product behavior. This continuous learning process makes generative AI a powerful tool for maintaining high-quality standards in manufacturing, thereby significantly enhancing the efficiency and effectiveness of quality control.
Supply chain and logistics
AI significantly enhances supply chain operations in manufacturing by optimizing distribution and logistics processes. Machine learning algorithms analyze historical data to forecast demand accurately, enabling manufacturers to coordinate distribution channels effectively. AI-driven systems continuously assess real-time variables, such as traffic patterns, to optimize logistics for cost-effective and timely deliveries. Through predictive analytics, AI aids in inventory management by anticipating fluctuations in demand, minimizing excess stock, and preventing shortages. This proactive approach not only streamlines the entire supply chain but also ensures efficient utilization of resources. By automating routine decision-making and offering data-driven insights, AI transforms manufacturing supply chains, fostering increased efficiency, reduced costs, and improved overall performance.
View: Inventory Optimization Flow
Financial management
AI transforms financial management in the manufacturing sector by seamlessly integrating advanced systems for budgeting, accounting, and financial reporting. AI streamlines financial processes specific to the manufacturing landscape through automation, ensuring precision in cost tracking and financial documentation. Leveraging predictive analytics, AI facilitates efficient resource allocation, allowing manufacturers to deploy funds for initiatives that drive innovation and growth strategically. Machine learning algorithms analyze historical financial data, enabling proactive decision-making for optimal financial performance. This integration not only enhances efficiency and compliance but also empowers financial teams in manufacturing to strategically allocate resources, fostering a more agile and resilient financial ecosystem within the manufacturing industry.
Environmental and sustainability practices
AI plays a crucial role in advancing environmental and sustainability practices in manufacturing. By employing machine learning algorithms, AI optimizes manufacturing processes, identifying opportunities to minimize resource consumption and reduce waste. AI-driven predictive analytics enable manufacturers to forecast environmental impacts and proactively implement measures to mitigate them. Additionally, AI facilitates real-time energy usage and emissions monitoring, ensuring compliance with environmental regulations. Through data analysis, AI enables manufacturers to make informed decisions on sustainable materials sourcing, further reducing the ecological footprint. Smart sensors and AI-powered systems enhance efficiency in resource utilization, contributing to a more sustainable and eco-friendly manufacturing environment. AI is a powerful ally in aligning manufacturing practices with environmental goals, promoting a greener and more responsible approach to production.
Health and safety compliance
AI significantly aids health and safety compliance by automating and enhancing various aspects of workplace protocols. AI monitors and enforces health and safety measures in real-time, ensuring adherence to protocols such as social distancing and proper Personal Protective Equipment (PPE) usage. Machine learning algorithms analyze historical safety data to identify patterns and potential risks, enabling proactive measures to prevent accidents and injuries. AI-driven predictive analytics assess the effectiveness of existing safety protocols, providing insights for continuous improvement. Moreover, AI facilitates monitoring compliance with occupational safety regulations, helping organizations maintain a secure working environment and avoid regulatory penalties. The integration of AI in health and safety compliance enhances workplace safety and enables data-driven decision-making to enhance safety measures continuously.
Corporate governance and internal controls
AI is crucial in bolstering corporate governance and internal controls within organizations. By leveraging advanced analytics and machine learning algorithms, AI contributes to effective decision-making structures, enhancing transparency and accountability. It enables real-time monitoring of vast datasets to detect anomalies, supporting internal controls for ethical conduct and risk management. AI-driven systems can automatically identify and flag potential fraud or non-compliance, fortifying the protection of company assets. Moreover, AI facilitates the creation of adaptive governance frameworks that can evolve based on emerging risks and changing business landscapes. This technology ensures a proactive approach to compliance, minimizing the likelihood of regulatory breaches.
Optimize Your Operations With AI Agents
Optimize your workflows with ZBrain AI agents that automate tasks and empower smarter, data-driven decisions.
How does LeewayHertz’s generative AI platform streamline manufacturing workflows?
LeewayHertz’s generative AI platform, ZBrain, is a powerful tool for manufacturing businesses aiming to streamline operations, enhance customer interactions, and drive growth. With its ability to create highly customized LLM-based applications trained on client’s proprietary data, ZBrain presents a unique opportunity to enhance your manufacturing workflows by boosting customer service and operational efficiency. ZBrain harnesses the full potential of clients’ business data across various formats, such as texts, images, and documents and uses popular large language models like GPT-4, Vicuna, Llama 2, and GPT-NeoX. This AI platform is designed to enhance decision-making, offer deeper insights, and boost productivity, all with a steadfast commitment to data privacy.
In the manufacturing sector, challenges like inconsistent quality control, inefficient defect analysis, and lengthy decision-making processes are common. ZBrain effectively addresses these issues with its specialized flows, which enable effortless, code-free business logic creation with its intuitive interface. It easily integrates various large language models, prompt templates, and media models, using drag-and-drop tools to construct and modify sophisticated, intelligent applications.
By leveraging AI-driven automation and advanced data analysis, these flows transform complex data into actionable insights, ensuring higher efficiency, reduced error rates, and better overall product quality, thereby providing comprehensive solutions to some of the most pressing problems in the manufacturing industry. Here are a few of them –
AI-driven supply chain optimization
ZBrain addresses complex supply chain optimization challenges for manufacturing businesses. The LLM-based apps created using ZBrain enhance supply chain visibility, predict demands, and streamline inventory management. This results in significant benefits for businesses including reduced operational costs, minimized waste, and improved delivery times. You can check out this flow to understand how ZBrain helps achieve a more responsive and efficient supply chain that swiftly adapts to market changes and customer needs.
AI-driven quality control and defect analysis
ZBrain transforms quality control and defect analysis for manufacturing enterprises. ZBrain’s LLM apps streamline the identification of product defects, enhancing accuracy and consistency in quality checks. The result is a dramatic reduction in the time and effort required for manual inspections, leading to improved product quality and operational efficiency. To understand how ZBrain brings about more precise, reliable, and cost-effective manufacturing processes, and helps businesses uphold high-quality standards, you can explore this quality control flow.
AI-driven production planning and optimization
ZBrain has also been used to streamline production planning and optimization for manufacturing firms. ZBrain’s LLM-powered apps boost forecasting accuracy, optimize resource allocation, and enhance production scheduling. The outcomes include heightened efficiency, reduced waste, and improved resource use. To see how this implementation has provided manufacturers with greater operational agility, quicker response to market demands, and increased productivity, advancing production management strategies, you can explore the detailed flow process here.
AI-driven automated supplier evaluation
ZBrain’s capabilities have been leveraged to transform supplier evaluation in manufacturing. LLM-powered apps developed using ZBrain automate supplier performance and compliance assessment, enhancing supply chain efficiency. The result is faster, more accurate evaluations, reduced risk of disruptions, and improved decision-making. Consequently, manufacturers enjoy a more dependable supplier network, leading to smoother production and increased business efficiency. For an in-depth understanding of how ZBrain refines supplier evaluation, you can view the detailed flow here.
LeewayHertz’s AI development services for manufacturing
At LeewayHertz, we craft tailored AI solutions that cater to the unique requirements of manufacturing firms. We provide strategic AI/ML consulting that enables manufacturers to harness AI for enhanced decision-making, improved operational efficiency, and optimized production strategies.
Our expertise in developing Proof of Concepts (PoCs) and Minimum Viable Products (MVPs) allows firms to preview the potential impacts of AI tools in real manufacturing scenarios, ensuring that the solutions are both effective and tailored to the industry’s specific needs.
Our work in generative AI also transforms routine tasks like report generation and data management, automating these processes to free up engineers and managers for more strategic roles.
By fine-tuning large language models to the nuances of manufacturing terminology and processes, LeewayHertz enhances the accuracy and relevance of AI-driven communications and analyses.
Additionally, we ensure these AI systems integrate seamlessly with existing technological infrastructures, enhancing operational efficiency and decision-making in manufacturing firms.
Our AI solutions development expertise
Generative AI solutions development for manufacturing typically involves creating systems that enhance decision-making, automate routine tasks, and optimize production processes. These solutions integrate key components such as data aggregation technologies, which compile and analyze operational information from diverse sources. This comprehensive data foundation supports predictive analytics capabilities, allowing for the forecasting of production trends and potential issues, thus informing strategic decisions.
Additionally, machine learning algorithms are employed to tailor manufacturing processes to specific production requirements, ensuring that each step is optimized for efficiency and quality. These solutions often cover areas like production planning, quality control, supply chain management, and equipment maintenance.
Overall, generative AI solutions in manufacturing aim to enhance operational efficiency, improve product quality, and elevate the overall production process.
AI agent/copilot development for manufacturing
LeewayHertz builds custom AI agents and copilots that enhance various manufacturing operations, enabling companies to save time and resources while facilitating faster decision-making. Here is how they help:
Production optimization:
- Analyze manufacturing data to optimize production schedules and generate detailed performance reports.
- Use real-time and historical data to predict and mitigate production bottlenecks.
- Identify and analyze production trends, helping to forecast demand and adjust manufacturing processes accordingly.
Equipment maintenance:
- Analyze equipment data to predict when maintenance is required, preventing downtime.
- Automatically schedule maintenance activities based on equipment performance data.
- Continuously monitor machinery for signs of wear and tear, ensuring optimal performance and longevity.
Quality control:
- Use computer vision and machine learning to conduct automated quality inspections, ensuring product quality.
- Identify defects in real-time during production, allowing for immediate corrective actions.
- Analyze quality control data to identify patterns and root causes of defects, facilitating process improvements.
Supply chain management:
- Analyze inventory levels and predict future requirements, ensuring efficient stock management.
- Monitor and evaluate supplier performance to ensure timely and quality deliveries.
- Use predictive analytics to forecast demand and adjust supply chain operations accordingly.
Process automation:
- Automate routine tasks such as data entry, report generation, and process monitoring.
- Ensure data accuracy through automated validation and verification processes.
- Streamline manufacturing workflows, reducing manual intervention and increasing efficiency.
Production planning:
- Gather and analyze data from diverse sources, providing a comprehensive view of the production landscape.
- Develop customized production plans based on specific manufacturing goals and constraints.
- Provide real-time insights into production conditions and performance, supporting timely and informed decision-making.
Resource allocation and optimization:
- Recommend resource allocation strategies based on predefined models or rules.
- Identify imbalances in production loads and suggest adjustments to optimize resource utilization.
- Monitor and optimize energy consumption across the production process, reducing costs and environmental impact.
Anomaly detection:
- Continuously monitor production processes for anomalies, ensuring smooth operations.
- Identify and flag unusual activities or patterns that could indicate potential issues or inefficiencies.
- Provide proactive solutions to potential problems, minimizing disruptions.
Marketing and content generation:
- Create personalized marketing communications or educational materials based on manufacturing data.
- Support content creation for websites, social media, and marketing campaigns, tailored to the manufacturing sector.
- Analyze customer data to segment markets and identify potential sales opportunities.
AI agents and copilots not only increase the efficiency of manufacturing processes but also significantly enhance the quality of production and strategic decision-making. By integrating these advanced AI solutions into their existing infrastructure, manufacturing firms can achieve a significant competitive advantage, navigating the complex production landscape with innovative, efficient, and reliable AI-driven tools and strategies.
Explore ZBrain's manufacturing use cases
ZBrain finds widespread applicability in manufacturing, performing diverse critical functions. The following highlights key use cases of this GenAI platform within the manufacturing industry.
How to implement generative AI in manufacturing workflow?
Implementing generative AI in manufacturing involves several key steps, from initial consulting to ongoing development and optimization. Here is a comprehensive guide covering all aspects:
Needs assessment and consulting:
- Define objectives: Clearly outline the goals and challenges you aim to address with AI in manufacturing.
- Industry expertise: Engage with AI consultants with expertise in both AI technologies and the manufacturing domain.
Data strategy and integration:
Data collection: Identify relevant data sources, including sensors, IoT devices, and existing systems.
Data quality: Ensure data is accurate, complete, and representative of manufacturing processes.
Data cleaning: Remove inconsistencies and outliers in the data.
Integration: Develop a strategy to integrate AI into existing manufacturing data workflows.
Technology selection and model development:
- Choose AI technologies: Select appropriate technologies, such as machine learning, computer vision, or natural language processing, based on specific manufacturing needs.
- Training data: Prepare and use historical data to train machine learning models.
- Algorithm selection: Choose algorithms suited for the identified manufacturing tasks.
- Development: Implement and fine-tune AI models for optimal performance.
Integration with manufacturing systems:
- APIs and interfaces: Develop interfaces for seamless communication between AI systems and manufacturing processes.
- Real-time integration: Ensure AI models can operate in real-time environments for immediate decision-making.
Security, compliance, and human-machine interaction:
- Data security: Implement measures to safeguard sensitive manufacturing data.
- Regulatory compliance: Ensure compliance with industry regulations related to data and AI.
- User interfaces: Design intuitive interfaces for monitoring and control that facilitate collaboration between AI systems and human operators.
- Training programs: Provide training for personnel to effectively interact with AI technologies.
Monitoring, optimization, and feedback:
- Gradual implementation: Scale AI implementations incrementally across different stages of the manufacturing process.
- Continuous improvement: Regularly optimize AI models based on performance feedback and evolving manufacturing requirements.
- Real-time monitoring: Implement systems for real-time monitoring of AI applications.
- Stakeholder feedback: Gather feedback from end-users, stakeholders, and employees.
- Iterative improvements: Use feedback to iterate and enhance AI implementations continuously.
LeewayHertz, the leading provider of AI consulting and development services, navigates the entire generative AI development lifecycle with precision. We, at LeewayHertz, commence the process by assessing project requirements, ensuring a deep understanding of stakeholder expectations. Our strategic approach encompasses model selection, training methodologies, and resource allocation, followed by meticulous design, development, rigorous testing, and continuous optimization. The final stages involve seamless integration into the client’s ecosystem, backed by ongoing support to align with evolving needs. Our specialized team excels in guiding you through strategic AI implementation, addressing unique challenges, and building tailored solutions that elevate operational efficiency.
Technologies used in generative AI in manufacturing
Generative AI design transforms manufacturing by amalgamating a wide range of technologies, such as 3D printing, Computer-Aided Design (CAD) software, and various machine learning algorithms. These tools synergistically enable the creation of groundbreaking designs and the optimization of manufacturing processes, leading to superior production outcomes. By harnessing a blend of technologies to accelerate design processes, generative AI drives innovation, and deliver efficient and effective production outcomes in manufacturing. Let’s delve into some of the key technologies and methodologies that power generative AI design in manufacturing:
- 3D printing: 3D printing, a manufacturing technique that constructs products layer by layer, plays an indispensable role in rapidly generating prototypes and producing final products in generative design. Its fast-paced nature enables quick iterations and design refinements based on feedback, simplifying production while upholding quality.
- Computer-aided design: CAD software allows designers to craft 3D models of parts and products, facilitating the development of virtual prototypes and simulations for assessing a product’s robustness, stability, and longevity. CAD software, equipped with AI optimization tools, reduces product development iterations and costs, thus boosting efficiency.
- Machine learning: Machine learning algorithms can dissect diverse data types, from product specifications to customer feedback, to create product models that outperform traditional designs or lower costs. Machine learning also automates the design process, allowing designers to refine their ideas and create superior products more efficiently and swiftly.
- Topology optimization: This technique optimizes the structure of a design to meet specific performance requirements. By progressively removing material from the design while maintaining its structural integrity, engineers can develop lighter, more efficient designs that deliver performance targets while minimizing weight.
- Finite Element Analysis (FEA): FEA allows engineers to simulate a design’s performance under varying stress, heat, and vibration conditions. These simulations enable engineers to optimize designs to meet specific operational conditions, ensuring robustness and reliability.
- Natural Language Processing: Text-based generative design technologies transform written descriptions into design options, making the design process faster and more personalized. By understanding the text and using algorithms to propose design ideas, this technology accelerates the design process and results in products that align closely with user needs and preferences.
- Reinforcement learning: Reinforcement Learning trains an AI agent to modify a design by rewarding it for achieving objectives like weight minimization or strength maximization. The AI agent learns via trial and error, exploring various design options and receiving feedback based on their alignment with the desired objectives. This technique combines AI with engineering principles to augment the design process and deliver superior outcomes.
Benefits of generative AI in manufacturing
Cybersecurity
Generative AI’s role in cybersecurity is monumental, particularly in manufacturing. These AI-powered systems provide constant vigilance, spotting potential security threats in real time, including unusual network activities or suspicious behaviors. The heart of these systems lies in complex machine learning algorithms that learn from historical data, including information about past attacks.
These algorithms detect specific patterns and activities related to cyber threats, harnessing this knowledge to forecast and efficiently adapt to new and constantly changing threats. This capability allows manufacturing companies to respond to cyber threats reactively and proactively act to prevent cyberattacks before they inflict substantial damage.
Additionally, these AI-infused systems continually learn and improve their defense strategies by studying each new encounter with cyber threats or security breaches. This continuous learning makes them more robust, forming an ever-advancing frontline defense. This aids manufacturers in operating with greater confidence and security amid the ever-changing cyber threat landscape.
AI-driven inspection and quality assurance
Generative AI, a subfield of AI, plays a vital role in enhancing manufacturing processes and boosting worker safety. Using high-resolution cameras and AI-driven algorithms, systems enabled by generative AI can detect flaws and inconsistencies that might escape human scrutiny. This ability for prompt detection enables instant corrections, reducing waste and recall incidences.
In addition, generative AI extends its application beyond mere defect identification. It constantly monitors the working environment, rapidly recognizing potential risks such as gas leaks, and promptly sends alerts to workers to undertake necessary preventive actions. This forward-thinking safety intervention assists in avoiding accidents and fosters a safer workplace.
The utilities of generative AI are not limited to safety measures but have a profound effect on productivity and efficiency as well. Quality control automation reduces the need for human involvement, freeing workers to concentrate on more intricate tasks, thereby enhancing productivity. Additionally, the early detection of defects ensures manufacturers can uphold high-quality control standards and diminishes the likelihood of inferior products reaching the market.
AI-enabled purchasing price variance
Generative AI, integrated into procurement systems, can drastically transform the landscape of manufacturing operations. It offers vital features like the estimation of raw material costs and aids in the selection of suitable vendors. These systems seamlessly bring together procurement data from an array of sources, augmenting the visibility and management of the supply chain.
The capability of generative AI to analyze multifaceted data enables manufacturers to pinpoint the best suppliers based on specific criteria, such as price, quality, and delivery timelines. This streamlines procurement processes and gives manufacturers the data-driven insights needed to make informed decisions.
These advanced generative AI systems enhance operational efficiency and enable effective supplier management by optimizing procurement operations. Furthermore, as generative AI learns and refines its analysis over time, it becomes an increasingly valuable tool for predicting market trends and making future procurement strategies more resilient.
In essence, the integration of generative AI into procurement operations equips manufacturers with the ability to refine their sourcing strategies, driving operational excellence and ultimately leading to a more efficient, productive, and adaptive manufacturing process.
Adaptability and continuous enhancement
In the manufacturing industry, generative AI models exhibit a profound capability to learn from their environment and progressively improve their performance over time. This ensures consistently producing high-quality results and effective solutions to evolving challenges. As product demands shift in response to market trends and consumer needs, these AI models can be swiftly retrained to adapt and fine-tune their outputs, enhancing the overall production process and maximizing the return on investment.
The key strength of generative AI in manufacturing lies in its remarkable adaptability and continuous learning ability. This makes them incredibly effective and valuable tools for various manufacturing applications. By learning from their past performance, they can self-improve, providing consistent enhancements and the flexibility to address changing production needs.
In the long run, generative AI’s self-learning and adaptive capabilities not only optimize current manufacturing processes but also pave the way for future success in the industry. They stand ready to adjust to evolving market dynamics, continuously delivering value across diverse manufacturing scenarios. Thus, the integration of generative AI in manufacturing elevates immediate productivity and fortifies the industry’s resilience to future market changes.
Cost reduction
Generative AI plays a pivotal role in manufacturing by significantly curbing overhead expenses, particularly those associated with unexpected downtime. Such unexpected pauses in production can prove costly for manufacturers, leading to considerable financial setbacks.
Manufacturers can deploy predictive maintenance measures that effectively prevent unplanned downtime by incorporating generative AI into their operational strategies. Predictive maintenance leverages advanced AI algorithms to predict potential machinery malfunctions before they occur, enabling proactive maintenance and thereby averting sudden interruptions in production.
This strategic approach helps manufacturers circumvent costly disruptions and optimizes productivity by ensuring seamless operations. Moreover, it aids in minimizing financial losses that could be incurred from unexpected equipment failures.
Integrating generative AI in manufacturing operations is a powerful tool for cost reduction. It proactively addresses potential issues before they escalate, reducing costs, optimizing efficiency, and significantly contributing to a more sustainable and profitable manufacturing environment.
Sustainable design solutions
Generative AI in the manufacturing industry catalyzes sustainable and eco-friendly product design. It meticulously considers various parameters such as recyclability and environmental impact while designing products. This enables manufacturers to engineer products that align with eco-friendly norms, thereby minimizing their environmental footprint.
By factoring in these considerations, manufacturers can substantially reduce their carbon emissions and play a proactive role in environmental conservation. Generative AI provides the ability to optimize product designs with an emphasis on sustainability, enabling manufacturers to align their practices with the principles of green manufacturing.
Through generative AI, manufacturers can design high-performing, cost-effective, and environmentally conscious products. This fosters a shift towards sustainable manufacturing, promoting a greener and more sustainable future.
Accelerated design cycles
Generative AI is a game-changer in the manufacturing industry by accelerating the design process. It assists designers by automating labor-intensive tasks in the design cycle, freeing up their time for more strategic work.
Rather than manually creating and evaluating prototypes, designers can delegate these tasks to the AI system. Generative AI models can generate multiple design options, run simulations, and assess each design’s viability, drastically reducing the number of iterations required to reach the desired outcome.
This efficiency enables designers to traverse the design cycle faster, arriving at the optimal solution in significantly less time. The integration of generative AI not only speeds up the design process but also enhances the quality and effectiveness of the design, leading to improved products and shorter time-to-market.
Enhanced diversity in design
Generative AI is a powerful creative tool in the manufacturing industry, enabling designers to devise various product designs endowed with unique attributes. These designs can be fine-tuned to satisfy specific criteria such as cost efficiency, enhanced performance, or durability. This opens up a wide spectrum of possibilities, allowing designers to select the most suitable solution that aligns perfectly with their unique needs and expectations.
Generative AI fosters a flexible and efficient approach to product development. It eliminates the limitations of traditional design methods by allowing designers to experiment with many design options. This ensures a comprehensive exploration of design possibilities, achieving an optimal outcome that best fits the specific requirements.
Increased performance
Generative AI plays a pivotal role in amplifying product performance in the manufacturing sector. It harnesses customer feedback data as a valuable resource for refining and optimizing product designs. This enables manufacturers to align their products more closely with customer expectations, leading to the production of superior-performance products.
Such an approach ensures the delivery of high-performing products, cultivates customer loyalty and boosts sales growth. Manufacturers can create bespoke products that resonate with specific customer needs and expectations by embedding customer preferences and insights into the design process.
Generative AI in design is a significant asset for manufacturers, enhancing customer satisfaction, fostering robust relationships, and paving the way for business growth. By tailoring products to customer needs and expectations, manufacturers can ensure they deliver high-quality, high-performance products that truly resonate with their customers, driving both customer satisfaction and business success.
Endnote
Generative AI has brought about a profound and positive change for the manufacturing sector. It elevates human capabilities by complementing human efforts with AI-driven innovation and creativity, empowering manufacturers to explore new possibilities and ideas. Additionally, it leads to a considerable improvement in operational efficiency, streamlining processes and optimizing production. The impact of generative AI extends beyond businesses into broader societal contexts. In the manufacturing industry, generative AI has the potential to bring about a significant transformation by improving design processes and enhancing overall efficiency, crucial in the fast-paced, competitive environment of Industry 4.0. Generative AI offers a promising path for the manufacturing sector to thrive in this new era of advanced technologies and digitalization.
Moreover, when integrated effectively, generative AI unlocks new growth opportunities, helping manufacturing businesses stay ahead of the curve, paving the way for a more innovative and sustainable future.
Harness the transformative power of generative AI to increase efficiency, boost innovation, and gain a competitive edge in your manufacturing operations. Get in touch with LeewayHertz AI experts today to start your AI journey!
Start a conversation by filling the form
All information will be kept confidential.
FAQs
How can generative AI be used in the field of manufacturing?
Generative AI in manufacturing can be employed for design optimization, prototyping, and even creating entirely new product concepts. By generating variations of designs based on specified parameters, it accelerates the product development process and facilitates innovation in manufacturing.
How do large language models help in design and manufacturing?
Large language models can assist in generating detailed documentation, conducting complex analyses, and facilitating communication between team members. Their natural language processing capabilities streamline tasks, enhance collaboration, and ultimately contribute to more efficient design and manufacturing processes.
How will AI change manufacturing industry?
AI is set to transform the manufacturing industry by optimizing production processes, predicting maintenance needs, and enhancing product design. Automation, enabled by AI, will lead to increased efficiency, reduced costs, and a more agile manufacturing industry capable of adapting to rapidly changing market demands.
What does AI mean for the future of manufacturing?
The future of manufacturing with AI signifies a shift towards intelligent, data-driven, and adaptive processes. AI technologies will play a pivotal role in creating smart factories, improving decision-making, and fostering innovation, ultimately leading to a more sustainable and competitive manufacturing landscape.
What are the key advantages of using generative AI in manufacturing?
The key advantages of using generative AI in manufacturing include enhanced design exploration, reduced time-to-market, improved product performance through optimization, and the ability to generate cost-effective and resource-efficient solutions.
Can generative AI be applied to specific manufacturing processes?
Yes, generative AI can be applied to specific manufacturing processes. It can be tailored to address the unique requirements and constraints of different processes, such as additive manufacturing, CNC machining, and assembly line optimization.
How can generative AI improve manufacturing processes for cost efficiency?
Generative AI can improve manufacturing processes for cost efficiency by optimizing designs, materials, and production workflows. It helps identify the most cost-effective solutions, reduces material wastage, and enhances overall operational efficiency, thereby lowering production costs.
How can LeewayHertz's generative AI development services help my manufacturing enterprise?
Here are some ways in which LeewayHertz’s generative AI development services can benefit your manufacturing enterprise:
- Customized product design: LeewayHertz’s generative AI solutions can create unique and customized product designs that meet specific requirements and constraints.
- Optimized manufacturing processes: Advanced generative AI algorithms can optimize manufacturing processes, improving efficiency, reducing costs, and minimizing resource wastage.
- Rapid prototyping: Utilize generative AI for rapid prototyping, allowing for quicker iterations in the product development cycle and accelerating time-to-market.
- Cost reduction: By using LeewayHertz’s generative AI solutions to optimize material usage, production workflows, and overall operational efficiency, you will ensure cost efficiency for your business.
- Supply chain optimization: The solutions can enhance supply chain management, forecasting, and inventory optimization for improved overall performance.
- Adaptability to specific manufacturing processes: LeewayHertz’s generative AI services may be tailored to suit specific manufacturing processes within your enterprise, providing a more targeted and effective solution.
- Data-driven decision-making: LeewayHertz develops specialized GenAI solutions for analyzing and interpreting data from various sources, enabling data-driven decision-making for better business outcomes.
Reach out to LeewayHertz directly to discuss your specific needs and how they can tailor their generative AI solutions to address the unique challenges and goals of your manufacturing enterprise.
Can LeewayHertz integrate generative AI into my enterprise's existing manufacturing workflows, and how would it benefit the processes?
Yes, LeewayHertz specializes in seamlessly integrating generative AI solutions into your existing manufacturing workflows. This integration optimizes processes, enhances product design, and fosters innovation, leading to improved efficiency and reduced time-to-market.
How does LeewayHertz ensure transparency and accountability in data processing within AI-powered manufacturing systems?
LeewayHertz prioritizes transparency and accountability in data processing. We implement audit trails and transparent data processing practices within AI-powered manufacturing systems, ensuring a clear record of data activities.
How does LeewayHertz ensure data privacy and security when implementing AI solutions in manufacturing operations?
At LeewayHertz, data privacy and security is a top priority. We implement robust encryption access controls and adhere to stringent data protection regulations to safeguard sensitive information in AI-powered manufacturing systems.
- What is generative AI?
- How does generative AI for manufacturing work?
- Generative AI use cases in manufacturing
- How LeewayHertz’s generative AI platform streamlines manufacturing workflows?
- LeewayHertz’s AI development services for manufacturing
- How to implement generative AI in manufacturing workflow?
- Technologies used in generative AI in manufacturing
- Benefits of generative AI in manufacturing
- Contact us