AI-ready data: Roadblocks, best practices, benefits, applications, tools and technologies, and future trends
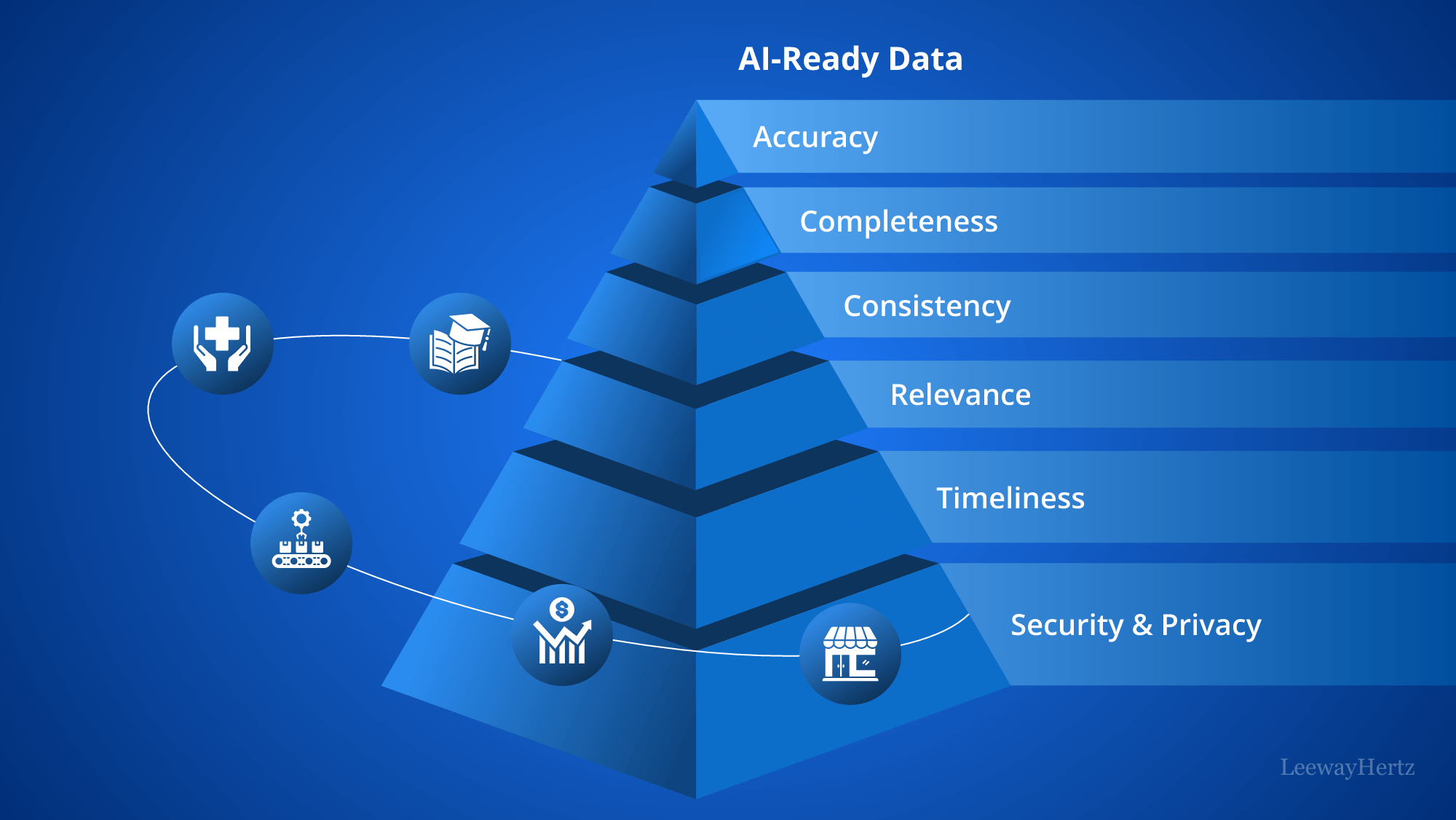
Data is the fuel for AI systems, driving their ability to deliver insights, make predictions, and transform decision-making processes. However, the effectiveness of AI solutions is heavily dependent on the quality and readiness of the data it processes. According to a recent Harvard Business Review article, a staggering 80% of AI projects fail, with poor data quality, lack of relevant data, and insufficient understanding of AI’s requirements being key contributors to this high failure rate.
The challenge of data readiness for AI is significant, as highlighted by a survey conducted by Scale AI (Hwang, 2022). This survey reveals that many participants face difficulties related to data readiness within their machine learning (ML) projects. Notably, data quality emerges as the biggest hurdle, with a survey of over 1,300 ML practitioners indicating that a third of respondents encounter issues related to data quality. Other common problems include difficulties with data collection, analysis, storage, and versioning, as detailed in the Zeitgeist: AI Readiness Report by Scale AI.
With AI technologies projected to contribute up to $15.7 trillion to the global economy by 2030, the emphasis on preparing data to meet AI requirements is more pressing than ever. Organizations must recognize that investing in AI-ready data is not just a technical necessity but a strategic imperative. The ability to harness data effectively will determine the success of AI initiatives and, by extension, the competitive edge of businesses in an increasingly data-driven world.
An effective approach to ensuring data readiness involves addressing the gaps identified in these surveys and reports. This includes developing robust data management practices, investing in data quality improvement initiatives, and adopting technologies that streamline data integration and processing. By tackling these issues proactively, organizations can enhance their data readiness, reduce the risk of AI project failures, and better leverage AI technologies to drive growth and innovation.
This article explores what constitutes AI-ready data and why it is essential for effective AI implementation. We will examine the key roadblocks organizations face in achieving data readiness and discuss the factors that make data truly AI-ready. Additionally, we will delve into best practices for data preparation, the principles guiding AI-ready data, and how to align data with specific use-case requirements. By understanding these elements, businesses can ensure their data is not only ready for AI but optimized to drive significant value.
- What is AI-ready data?
- Why is AI-ready data important?
- Key drivers of AI-ready data
- How AI-ready data differs from traditional data
- The roadblocks to AI-ready data: Overcoming obstacles to success
- Assessing data readiness for AI
- AI-ready data infrastructure and architecture: Laying the foundation for success
- The 6 principles of AI-ready data: A framework for success
- How to ensure data readiness for AI: A practical approach to data preparation
- Applications of AI-ready data across industries
- Benefits of AI-ready data
- Tools and technologies for AI-ready data
- Best practices for optimizing data readiness for AI
- How can LeewayHertz help you get AI-ready data?
- Future trends and innovations in AI-ready data
What is AI-ready data?
AI-ready data refers to data that is well-prepared and structured in a manner that maximizes its effectiveness for use in artificial intelligence applications. This concept goes beyond merely having large amounts of data; it emphasizes the quality, structure, and relevance of the data, ensuring that it can be efficiently processed and analyzed by AI algorithms to generate meaningful insights. It’s not just about having large amounts of data; it’s about having the right information in the right format and with the right context to enable AI to function effectively and deliver meaningful insights.
Key characteristics of AI-ready data include:
- High quality: AI-ready data must be accurate, reliable, and consistent. Quality data is free from errors, duplications, and inconsistencies, which can otherwise mislead AI models and degrade their performance.
- Structured format: While AI can handle unstructured data, having data in a structured format (such as tables and databases) significantly enhances processing efficiency. Structured data is organized in a predefined manner, making it easier to query and analyze.
- Comprehensive coverage: AI models require a diverse range of data to make accurate predictions and decisions. AI-ready data should cover all relevant aspects of the problem domain, capturing a broad spectrum of scenarios and variables.
- Timeliness and relevance: Data must be up-to-date and pertinent to the current context. Timely data ensures that AI models reflect recent trends and changes, while relevant data directly aligns with the objectives of the AI application.
- Data integrity and security: Ensuring that data remains intact and protected from unauthorized access is crucial. AI-ready data must be safeguarded against breaches and corruption, which could otherwise compromise the integrity of the AI models built upon it.
Why is AI-ready data important?
AI-ready data is the lifeblood of artificial intelligence. Without it, even the most sophisticated AI algorithms are powerless. Imagine trying to build a house without bricks and mortar – the result would be a flimsy, unusable structure. AI-ready data provides the foundation for building robust and reliable AI systems.
Here’s why AI-ready data is so critical:
1. Fueling accurate AI models:
AI models learn from data. The quality and relevance of the data directly impact the accuracy and effectiveness of the models. AI-ready data ensures that the models are trained on:
- Relevant information: Data that directly relates to the AI’s intended purpose. This ensures the AI learns the right patterns and avoids being misled by irrelevant information.
- Complete data: Data that covers all relevant aspects of the problem domain. This helps AI models develop a holistic understanding and avoid biases.
- Accurate and consistent data: Data that is free from errors, duplications, and inconsistencies. This is essential for building trustworthy and reliable AI systems.
2. Enabling powerful AI applications:
AI-ready data unlocks the potential of AI applications across industries. It allows us to:
- Automate tasks: High-quality data enables AI to efficiently automate routine processes, such as customer service, data entry, and inventory management. By providing accurate and consistent data, AI systems can perform these tasks with precision, freeing up human resources for more strategic activities.
- Optimize processes: AI algorithms can analyze data to identify inefficiencies and optimize workflows in areas like supply chain management, resource allocation, and marketing campaigns.
- Personalize customer experiences: AI can use data to tailor products, services, and recommendations to individual customer needs, enhancing satisfaction and loyalty.
- Drive scientific discoveries: AI can analyze large datasets in fields like healthcare, research, and development to accelerate breakthroughs in drug discovery, disease diagnosis, and scientific exploration.
3. Achieving tangible business outcomes:
AI-ready data is the key to achieving real-world results with AI. By leveraging high-quality data, businesses can:
- Increase efficiency and productivity: Automate tasks, optimize processes, and make better decisions, leading to significant improvements in performance.
- Improve customer satisfaction: Offer personalized experiences, enhance customer service, and build stronger relationships.
- Gain a competitive advantage: Develop innovative products and services, personalize customer experiences, and create new business models.
- Reduce costs and risks: Optimize operations, mitigate potential threats, and make more informed decisions.
In essence, AI-ready data is the foundation for building intelligent systems that deliver real value and drive meaningful change. It is the key to unlocking the full potential of AI and ushering in a more intelligent and efficient future.
Key drivers of AI-ready data
Understanding the key drivers behind the need for AI-ready data is essential for organizations looking to leverage AI technologies effectively. Here are the primary factors influencing the demand for data that is optimized for AI applications:
1. Vendor-provided models:
As many AI models, particularly those involving generative AI, are sourced from external vendors, it becomes crucial for enterprises to focus on optimizing their data to maximize the benefits of these pre-trained models. These models are designed to perform well with high-quality, well-structured data. Organizations must ensure their data aligns with the requirements of these models to fully leverage their capabilities and achieve the desired outcomes.
2. Data availability and quality:
High-quality data is a fundamental requirement for effective AI solutions. Organizations often overlook AI-specific data management challenges, such as data bias and quality issues. Addressing these challenges is critical for the successful implementation of AI systems. Ensuring that data is accurate, representative, and free from biases is essential for developing reliable and trustworthy AI models.
3. Disruption of traditional data management:
The rapid advancement of AI technologies is driving a shift from traditional data management practices to more dynamic and innovative approaches. Techniques such as data fabrics and augmented data management are becoming increasingly important. Innovations like knowledge graphs enhance the effectiveness of AI models by providing contextual information and improving data integration and retrieval processes.
4. Bias and hallucination mitigation:
New data management solutions are being developed to address AI-specific issues such as bias and hallucinations. Effective data management strategies play a crucial role in structuring and preparing data to mitigate these challenges. By implementing robust data governance and quality control measures, organizations can reduce the impact of biases and inaccuracies in AI models.
5. Integration of structured and unstructured data:
Generative AI technologies are breaking down the traditional boundaries between structured and unstructured data. This evolution necessitates that data management practices adapt to handle a variety of data formats and uses. Organizations need to develop strategies that can manage and integrate diverse types of data, including text, images, audio, and video, to fully capitalize on the capabilities of generative AI.
By addressing these drivers, organizations can better prepare their data for AI applications, ensuring it is of high quality, well-structured, and capable of supporting the sophisticated requirements of modern AI technologies.
How AI-ready data differs from traditional data
Traditional data often lacks the preparation necessary for optimal AI utilization. The primary differences between AI-ready data and traditional data include:
Aspect | AI-ready data | Traditional data |
---|---|---|
Preparation and cleaning | Pre-cleaned and pre-processed for immediate AI use. | Often raw and unprocessed, requiring extensive cleaning. |
Integration | Integrated from multiple sources into a unified dataset. | Often fragmented across different systems and formats. |
Structure and format | Typically structured and standardized for easy querying. | May be unstructured or inconsistently formatted. |
Metadata and annotations | Includes comprehensive metadata and contextual information. | Often lacks detailed metadata and annotations. |
Timeliness | Data is up-to-date and relevant to current contexts. | Data may be outdated or less relevant. |
Scalability | Designed to scale with growing data volumes and needs. | May struggle with scalability, especially with large datasets. |
Accessibility | Easily accessible and usable by AI tools and models. | Accessibility may be limited or require additional processing. |
Quality and integrity | High-quality data with minimal errors and inconsistencies. | Quality issues such as inaccuracies and duplications may be prevalent. |
Compliance and security | Managed with strong security measures and compliance protocols. | Security and compliance may be less robust, potentially exposing data to risks. |
Processing requirements | Optimized for efficient processing and analysis by AI models. | May require additional processing to be suitable for AI analysis. |
The roadblocks to AI-ready data: Overcoming obstacles to success
While the potential of AI-ready data is immense, achieving it is not without its challenges. Many roadblocks can hinder the journey toward a robust and reliable data ecosystem, potentially jeopardizing AI projects and their intended outcomes. This section explores some of the most common obstacles to achieving AI-ready data and provides insights into how to overcome them.
Data silos: The challenge of fragmented information
Data silos occur when different departments or systems within an organization store data independently, creating isolated pockets of information. This fragmentation hinders data accessibility, analysis, and utilization.
Impact: AI models require a holistic view of data to learn meaningful patterns and make accurate predictions. Silos limit the scope of data available for training, leading to biased models and inaccurate results.
Solution: Establish a centralized data repository, such as a data lake, to consolidate data from various sources. Implement data integration strategies to connect disparate systems and break down silos.
Data inconsistency: The pitfalls of lacking standardization
Inconsistencies in data formats, definitions, and values across different sources can create confusion and hinder AI processing.
Impact: Inconsistent data can lead to errors, biases, and unreliable AI model outputs. Imagine a model trained on data where “male” is sometimes represented as “M” and sometimes as “Male” – this could lead to inaccurate results.
Solution: Define clear data standards and formats for all data elements. Implement data quality checks and validation processes to identify and correct inconsistencies. Consider using data governance tools and frameworks to enforce consistency across the entire data ecosystem.
Data quality issues: Errors, missing values, and incompleteness
Data quality problems, such as errors, missing values, and incomplete information, can significantly impact AI model accuracy.
Impact: Inaccurate or incomplete data can lead to biased models, inaccurate predictions, and unreliable AI outputs. If a dataset lacks customer income information for a large percentage of individuals, an AI model trying to predict purchasing behavior will be severely limited.
Solution: Implement data cleaning and preprocessing techniques to address data quality issues. Utilize data imputation methods to handle missing values and leverage data enrichment techniques to fill in gaps.
Data privacy and security: Navigating ethical and legal considerations
In today’s data-driven world, privacy and security are paramount. Sensitive data must be handled with utmost care, adhering to legal regulations and ethical guidelines.
Impact: Data breaches and privacy violations can damage an organization’s reputation, erode customer trust, and incur significant legal penalties. AI projects involving sensitive data require robust security measures and strict compliance with regulations like GDPR and CCPA.
Solution: Implement robust data security protocols, including encryption, access control, and data masking. Adopt privacy-enhancing techniques like differential privacy and federated learning to protect sensitive data during AI model training.
Lack of expertise: The need for data science and engineering skills
Building and maintaining an AI-ready data ecosystem requires a team with specialized skills in data science, engineering, and AI.
Impact: Without skilled professionals, organizations may struggle to manage data effectively, implement data governance practices, and build robust AI solutions.
Solution: Invest in hiring or training data science and engineering professionals. Consider collaborating with data science consultants or partnering with organizations specializing in AI development and data management.
By actively addressing these challenges and investing in the right strategies, organizations can pave the way for a successful AI journey, where data becomes a powerful asset that fuels innovation and drives growth.
Assessing data readiness for AI
Ensuring data readiness is critical for the success of AI systems. It involves evaluating several key aspects of data to ensure it meets the requirements for effective AI performance:
- Data quality: This includes checking the completeness, accuracy, and consistency of the data. High-quality data should be comprehensive and free of errors.
- Representativeness: The data must accurately reflect real-world scenarios. This means it should capture a wide range of conditions relevant to the AI application.
- Feature relevancy and duplicity: Relevant features should be included, and any duplicate or irrelevant data should be eliminated to improve model performance.
- Class separability: For tasks involving classification, the data must enable clear differentiation between different classes.
- FAIR compliance: The data should be Findable, Accessible, Interoperable, and Reusable. This ensures that it can be effectively utilized and integrated into AI systems.
Conducting these assessments early in the machine learning (ML) pipeline is highly beneficial. Identifying data issues early allows organizations to take proactive steps to address them, improving the overall quality and robustness of AI models.
Challenges often faced during this process include handling missing or incomplete data, managing noisy or irrelevant features, and ensuring data privacy and security. Tools like data readiness reports and frameworks such as Lawrence’s band-based categorization (C, B, A) and the MIDaR scale for medical imaging data provide structured methods for evaluating and improving data readiness.
Recent research emphasizes the need for intelligent metrics and frameworks like OpenDataVal, which helps streamline data preparation by offering a unified environment for assessing data quality.
By thoroughly assessing and preparing data, organizations can enhance the effectiveness and reliability of their AI systems, leading to more accurate and trustworthy outcomes.
AI-ready data infrastructure and architecture: Laying the foundation for success
A robust and scalable data infrastructure is the cornerstone of a successful AI journey. It provides the foundation for storing, managing, processing, and analyzing vast amounts of data efficiently and effectively. This section explores key components of an AI-ready data infrastructure and architecture, highlighting how they contribute to unlocking the potential of AI.
1. Building a robust data ecosystem: Centralized vs. decentralized approaches
The first step in designing an AI-ready data infrastructure is to determine the optimal data ecosystem architecture. Two primary approaches exist:
- Centralized data ecosystem: Data is stored and managed in a single, centralized location, often in a data lake. This approach offers advantages in terms of governance, security, and data accessibility.
- Decentralized data ecosystem: Data is distributed across various systems and locations, often in a cloud-based environment. This approach provides greater flexibility and scalability but requires careful management to ensure data consistency and security.
The choice between centralized and decentralized approaches depends on factors like data volume, security requirements, and organizational structure.
2. Data lakes: A modern solution for data storage and management
Data lakes are increasingly popular for storing and managing diverse data sources in a centralized location. They offer several advantages for AI:
- Schema-on-read: Data is stored in its raw format, allowing for flexibility in how it’s accessed and analyzed later.
- Scalability and elasticity: Data lakes can easily scale to accommodate growing data volumes and evolving needs.
- Unified data repository: Data from various sources can be consolidated into a single repository, simplifying access and integration for AI applications.
3. Data pipelines: Automating data ingestion, processing, and transformation
Data pipelines automate the process of ingesting, cleaning, transforming, and preparing data for AI applications. They streamline data workflows, improve efficiency, and ensure data consistency.
- Ingestion: Data pipelines extract data from various sources, including databases, APIs, and files.
- Transformation: Data is cleaned, transformed, and enriched to meet the specific requirements of AI models.
- Loading: Processed data is loaded into a data lake or other storage systems.
4. Cloud-based solutions: Scaling up data storage and computing power
Cloud computing platforms offer scalable and cost-effective solutions for storing and processing large volumes of data. They provide flexible infrastructure and resources to meet the demanding requirements of AI workloads.
- Cloud storage services: Cloud providers like AWS, Azure, and Google Cloud offer scalable and secure storage options for large datasets.
- Cloud computing services: Cloud-based computing resources like virtual machines and serverless functions provide the necessary processing power for training and deploying AI models.
5. Choosing the right tools and technologies: A guide to data platforms
The choice of data platform and tools is crucial for building an AI-ready data infrastructure.
- Data warehousing platforms: Designed for structured data, providing advanced analytical capabilities. Examples include Google BigQuery, Azure Synapse Analytics, and Amazon Redshift.
- NoSQL databases: Suitable for unstructured and semi-structured data, offering flexibility and scalability. Examples include MongoDB, Cassandra, and Redis.
- Data pipelines and orchestration tools: Automate data ingestion, transformation, and loading. Examples include Apache Airflow, Luigi, and Prefect.
- Data governance tools: These tools help enforce data quality, security, and compliance policies. Examples include Alation, Collibra, and Dataiku.
By carefully considering these factors and selecting the appropriate tools and technologies, organizations can create a data infrastructure that supports efficient data management, processing, and utilization, unlocking the full potential of AI.
The 6 principles of AI-ready data: A framework for success
Ensuring that data is AI-ready involves adhering to key principles that enhance its quality, usability, and effectiveness for artificial intelligence applications. Here are the six essential principles of AI-ready data:
1. Accuracy: Accuracy refers to the correctness of the data. Accurate data precisely represents the real-world entities or events it is meant to capture.
Importance: For AI models to make reliable predictions and decisions, the data they are trained on must be correct. Inaccurate data can lead to erroneous outcomes and undermine the effectiveness of AI systems.
Best practices:
- Implement rigorous data validation processes.
- Regularly review and update data sources.
- Utilize automated tools to detect and correct errors.
2. Completeness: Completeness ensures that the data contains all necessary information required for comprehensive analysis and decision-making.
Importance: Incomplete data can result in gaps that affect the accuracy and reliability of AI models. Ensuring that all relevant aspects are covered is crucial for effective AI training and analysis.
Best practices:
- Identify and address missing data points.
- Integrate data from various sources to fill gaps.
- Use data imputation techniques to estimate and complete missing values.
3. Consistency: Consistency means that data is uniform and coherent across different datasets and sources, adhering to the same standards and formats.
Importance: Inconsistent data can lead to confusion and errors in AI models. Consistent data ensures that different datasets align and can be seamlessly integrated and analyzed.
Best practices:
- Establish and enforce data standards and formats.
- Regularly synchronize and reconcile data across sources.
- Use data integration tools to maintain consistency.
4. Relevance: Relevance ensures that the data is pertinent to the specific problem or application for which AI is being used.
Importance: Relevant data directly impacts the performance and accuracy of AI models. Irrelevant data can introduce noise and reduce the model’s ability to generate meaningful insights.
Best practices:
- Align data collection efforts with the goals of the AI application.
- Continuously evaluate and update data to ensure its relevance.
- Filter and select data that directly contributes to the AI model’s objectives.
5. Timeliness: Timeliness refers to current and up-to-date data reflecting the most recent and relevant information.
Importance: AI models require timely data to produce accurate and actionable insights. Outdated data can lead to decisions based on obsolete information, impacting the model’s effectiveness.
Best practices:
- Implement real-time data collection and updating mechanisms.
- Regularly review and refresh data to maintain its relevance.
- Use data streaming technologies to keep data current.
6. Security and privacy: Security and privacy ensure that data is protected from unauthorized access and misuse while also complying with data protection regulations.
Importance: Protecting data is essential for maintaining trust and compliance. AI systems must handle data securely to prevent breaches and ensure that sensitive information is safeguarded.
Best practices:
- Implement robust data encryption and access controls.
- Ensure compliance with data protection regulations such as GDPR and CCPA.
- Regularly audit data security practices and update them as needed.
In summary, adhering to these six principles—accuracy, completeness, consistency, relevance, timeliness, and security and privacy—is crucial for preparing data that is truly AI-ready. By following these principles, organizations can ensure that their data is well-suited for effective AI applications, leading to more reliable and actionable insights.
How to ensure data readiness for AI: A practical approach to data preparation
Transforming raw data into a form that is ready for AI applications involves a detailed and systematic approach. This guide outlines the essential steps to prepare data, ensuring it is usable and valuable for your AI models.
Understanding your data needs: Aligning data with use-case requirements
The first step in ensuring data readiness for AI is to align your data with the specific objectives of your AI model. This process involves understanding both the goals of your AI project and the nature of the data required to meet those goals.
Target variables: Begin by clearly defining what you aim to predict or analyze with your AI model. Target variables are the outcomes or results your model is designed to forecast or classify. Understanding these variables helps in selecting the appropriate input features that will provide relevant information for achieving your objectives.
Input features: Next, identify which data points are essential for predicting or analyzing the target variables. Input features are the individual data elements that contribute to the model’s ability to make accurate predictions. Determining which features are relevant involves understanding the data’s context and how each feature contributes to the target variables.
Data format: Specify the data format required by your AI model. Different models may require data in various formats, such as structured data (e.g., tables and spreadsheets), unstructured data (e.g., text documents and images), or time series data (e.g., sensor readings over time). Ensuring that your data aligns with the required format is crucial for the model’s performance and compatibility.
Data quality expectations: Establish the standards for data quality, including accuracy, completeness, and consistency. High-quality data is essential for reliable AI outcomes, as poor-quality data can lead to inaccurate or misleading results. Setting clear expectations for data quality helps in evaluating and maintaining the integrity of the data used for training and testing AI models.
Data cleaning and preprocessing: Addressing inaccuracies, inconsistencies, and missing values
Raw data often contains errors, inconsistencies, and gaps that need to be addressed to ensure its integrity and usability for AI models. The process of data cleaning and preprocessing involves several key tasks:
Missing value imputation: This step involves filling in gaps where data is missing. Techniques such as mean, median, or more advanced methods are used to estimate and replace missing values based on the context and distribution of the data. This process helps prevent gaps from affecting the analysis and model performance.
Outlier detection and removal: Outliers are extreme values that can distort the results of data analysis. Identifying and managing these outliers is crucial to ensure that they do not skew the model’s predictions. This can involve removing outliers or adjusting them to align more closely with the overall data distribution.
Data transformation: Data transformation involves converting raw data into formats suitable for analysis. This includes scaling and normalization to bring different features to a common scale or applying log transformations to manage skewed data. Transforming data helps improve the performance and accuracy of AI models.
Data standardization: Ensuring consistent data formats and units across different sources is essential for integration. Data standardization involves aligning formats and measurement units to facilitate smooth and accurate data processing. This ensures that data from various sources can be combined and analyzed cohesively.
Data deduplication: Removing duplicate records is important for maintaining data accuracy and avoiding bias. Data deduplication helps in ensuring that each record is unique and that repeated entries do not distort the results of the analysis or model predictions.
Data enrichment: Augmenting data with relevant context and features
Enriching data involves enhancing its value by adding additional context and features, which improves the AI model’s ability to analyze and interpret the information effectively. This process helps in making the data more comprehensive and relevant for model training and prediction.
Adding demographic information: Incorporating demographic details such as age, gender, location, and income into your data provides valuable context that can improve the model’s understanding of customer behavior and preferences. This additional information helps in creating more accurate and targeted insights, making the model’s predictions more relevant and actionable.
Adding external data sources: Combining your internal data with external data sources, such as weather data, market trends, or social media sentiment, provides a broader context and a more comprehensive view. This enriched dataset allows the AI model to capture additional factors that may influence outcomes, leading to more informed and precise predictions.
Creating derived features: Generating new features from existing data, such as calculating ratios, creating interaction terms, or deriving time-based features, enhances the model’s performance. Derived features can uncover hidden patterns and relationships within the data, improving the model’s ability to make more nuanced and accurate predictions.
Data transformation: Converting raw data into AI-friendly formats
AI models often require data to be in specific formats for effective analysis and prediction. Data transformation involves adapting raw data to meet these requirements, ensuring compatibility with the chosen AI algorithms.
Converting categorical variables: This step involves transforming text-based categories into numerical formats. Techniques such as one-hot encoding or label encoding are used to represent categorical data in a way that AI models can process. By converting categories into numerical values, the data becomes suitable for algorithmic analysis, allowing the model to understand and utilize these variables effectively.
Structuring unstructured data: Unstructured data, such as text, images, or audio, needs to be converted into structured formats to be useful for AI analysis. This involves applying methods like Natural Language Processing (NLP) for text or image recognition algorithms for visuals. Structuring unstructured data helps in extracting meaningful information and converting it into a format that can be readily analyzed by AI models.
Data aggregation: Data aggregation involves summarizing and grouping data into meaningful categories or aggregates. This process consolidates detailed data into higher-level summaries, making it easier to analyze trends and patterns. Aggregated data helps in simplifying complex datasets and provides a clearer view of the information, which is essential for generating insights and supporting decision-making.
Data validation: Ensuring accuracy, completeness, and consistency
After the processes of data cleaning, transformation, and enrichment, validating the quality of your data is essential to ensure it is suitable for use with AI models. This involves several key steps:
Data profiling: This step involves analyzing the characteristics of your data to uncover potential issues or biases. Data profiling helps in understanding the data’s structure, distribution, and quality, which can reveal inconsistencies, anomalies, or patterns that may affect the model’s performance.
Data consistency checks: Verifying that data adheres to expected patterns and relationships ensures that it is consistent across different sources and datasets. Consistency checks help in identifying discrepancies or errors that could lead to inaccurate analysis or predictions.
Data completeness checks: Assessing the completeness of data involves identifying and addressing any missing values or gaps. Ensuring that the dataset is complete is crucial for accurate analysis, as missing data can lead to skewed results or unreliable model performance.
Data integrity checks: Ensuring the accuracy and reliability of data involves verifying that the data has not been corrupted or altered. Data integrity checks help in maintaining the trustworthiness of the dataset, ensuring that the information used by the AI model is accurate and dependable.
By following these steps, you can transform raw data into a valuable resource that fuels your AI models, ensuring that your AI projects are built on a strong foundation of high-quality data.
Applications of AI-ready data across industries
AI-ready data is not just a technical concept; it’s a catalyst for real-world transformation across numerous industries. By harnessing the power of AI-ready data, organizations are unlocking innovative solutions, enhancing efficiency, and driving growth in a wide range of applications. Here are some key areas where AI-ready data is making a significant impact:
- Healthcare:
- Personalized medicine: AI-ready data from patient records, genetic sequencing, medical imaging, and wearable devices fuels personalized treatment plans, tailored drug therapies, and early disease detection. For example, AI models trained on large-scale datasets of patient data and genomics can predict individual responses to treatments, leading to more effective personalized care.
- Precision diagnostics: AI models trained on AI-ready data are transforming medical diagnostics. AI-powered image analysis can identify subtle patterns in medical scans, enabling faster and more accurate diagnoses of conditions like cancer and heart disease.
- Drug discovery and development: AI-ready data accelerates drug discovery by enabling the analysis of massive datasets of molecular structures, clinical trials, and patient outcomes. This allows researchers to identify promising drug candidates and optimize drug development timelines.
- Finance:
- Fraud detection: AI models analyzing AI-ready data from transaction histories, customer profiles, and market trends can detect fraudulent activities in real-time, protecting customers and reducing financial losses. For instance, AI-powered systems can identify unusual spending patterns or suspicious account activity, alerting authorities to potential fraud.
- Risk management: AI-ready data enables advanced risk management by analyzing financial data to assess creditworthiness, manage investment portfolios, and predict market volatility. This empowers financial institutions to make more informed and proactive risk mitigation decisions.
- Personalized financial advice: AI-powered financial advisors leverage AI-ready data to provide personalized recommendations on investments, budgeting, and financial planning, catering to individual needs and goals.
- Manufacturing:
- Predictive maintenance: AI-ready data from sensors, machinery logs, and production records allows for predictive maintenance, minimizing downtime and improving operational efficiency. AI models can analyze sensor data to identify early signs of equipment failure, enabling preventative maintenance and maximizing equipment lifespan.
- Process optimization: AI models analyze manufacturing data to optimize production processes, identify bottlenecks, and improve resource utilization, leading to significant cost savings and increased output. By analyzing real-time data from production lines, AI can identify inefficiencies and optimize workflows, improving overall productivity.
- Quality control: AI-powered vision systems, trained on AI-ready data, can inspect products for defects in real-time, ensuring quality control and reducing waste. AI-enabled quality inspection can automate defect detection, leading to improved product quality and reduced costs associated with manual inspection.
- Retail:
- Personalized recommendations: AI-ready data from customer purchase histories, browsing behavior, and demographics empowers personalized product recommendations, boosting sales and customer satisfaction. AI-powered recommendation engines can analyze customer data to predict preferences and recommend relevant products, increasing conversion rates and driving customer engagement.
- Inventory management: AI models analyze sales data and demand patterns to optimize inventory levels, reducing stockouts and minimizing waste. AI-powered inventory management systems can predict future demand and optimize inventory levels, ensuring that the right products are available at the right time while minimizing holding costs and waste.
- Customer segmentation: AI can analyze customer data to segment customers based on their behavior, preferences, and demographics, enabling targeted marketing campaigns and personalized experiences. This allows businesses to tailor their marketing efforts to specific customer segments, improving campaign effectiveness and customer engagement.
- Education:
- Personalized learning: AI-ready data from student performance records, learning styles, and engagement levels allows for personalized learning experiences, tailored to individual student needs. AI-powered learning platforms can adapt to individual learning styles, providing customized content and pacing, improving learning outcomes.
- Automated assessment: AI can automate the grading process, freeing up teachers’ time and providing immediate feedback to students. AI-powered grading systems can assess student work objectively and efficiently, providing timely feedback and allowing teachers to focus on more personalized instruction.
- Predictive analytics: AI models can analyze student data to identify students at risk of failing or dropping out, enabling early intervention and support. By predicting potential challenges, educators can intervene proactively to provide targeted support and prevent academic difficulties.
- Transportation:
- Autonomous vehicles: AI-ready data from sensors, GPS data, and mapping information powers the development of self-driving vehicles, improving road safety and efficiency. AI algorithms process real-time sensor data to navigate, make decisions, and interact with the environment, enabling autonomous driving.
- Traffic management: AI models analyze traffic data to optimize traffic flow, reduce congestion, and improve public transportation systems. By analyzing real-time traffic data, AI can optimize traffic light timings, suggest alternative routes, and improve the efficiency of public transportation systems.
- Fleet management: AI-powered fleet management solutions leverage AI-ready data to optimize route planning, driver scheduling, and vehicle maintenance, improving efficiency and reducing costs. AI can analyze data to optimize delivery routes, predict maintenance needs, and improve fuel efficiency, contributing to more cost-effective and sustainable fleet operations.
These are just a few examples of the wide-ranging applications of AI-ready data. As AI technology continues to advance, we can expect to see even more innovative applications emerge across every industry, driven by the power of high-quality data.
Benefits of AI-ready data
AI-ready data is a cornerstone of effective artificial intelligence applications, offering numerous advantages that drive better performance and outcomes across various domains. Here are some key benefits of having AI-ready data:
Enhanced accuracy and reliability: AI-ready data ensures that AI models operate with high accuracy and reliability. When data is well-structured, clean, and free of inconsistencies, it allows AI algorithms to produce more precise and dependable results. This accuracy is crucial for making informed decisions and achieving reliable predictions.
Improved model performance: Properly prepared data enhances the performance of AI models by providing the necessary context and relevance. AI-ready data enables models to learn more effectively, leading to improved performance in tasks such as classification, regression, and pattern recognition. Better data quality translates into more effective and efficient AI solutions.
Faster time-to-insight: With AI-ready data, organizations can achieve faster time-to-insight. Clean, well-organized, and relevant data speeds up the process of training and deploying AI models. This accelerated insight allows businesses to respond more quickly to market changes, customer needs, and operational challenges.
Cost savings: Investing in AI-ready data can lead to significant cost savings by reducing the need for extensive data cleaning and preprocessing during the AI model development phase. High-quality data minimizes the risk of errors and inefficiencies, leading to lower costs associated with data management, model retraining, and corrective actions.
Enhanced decision-making: AI-ready data supports better decision-making by providing accurate and comprehensive insights. Well-prepared data allows AI models to generate actionable intelligence, helping organizations make data-driven decisions with greater confidence. This leads to more strategic and effective business choices.
Scalability and flexibility: AI-ready data facilitates scalability and flexibility in AI applications. When data is structured and standardized, it is easier to scale AI solutions across different departments or business units. This flexibility allows organizations to adapt AI models to various use cases and expand their applications as needed.
Increased competitive advantage: Organizations that leverage AI-ready data gain a competitive advantage by harnessing the full potential of AI technologies. High-quality data enables more sophisticated and accurate AI models, leading to better customer insights, improved operational efficiency, and innovative solutions that set the organization apart from competitors.
Regulatory compliance: Ensuring data readiness helps organizations comply with regulatory requirements related to data quality, privacy, and security. Properly managed and validated data supports adherence to regulations and standards, reducing the risk of legal and compliance issues.
Better customer experiences: AI-ready data enhances the ability to deliver personalized and relevant customer experiences. By using clean and enriched data, organizations can tailor their products, services, and interactions to meet individual customer needs and preferences, leading to increased satisfaction and loyalty.
In summary, AI-ready data provides a foundation for successful AI implementation, leading to enhanced accuracy, improved performance, cost savings, and better decision-making. By ensuring data readiness, organizations can fully leverage the capabilities of AI and achieve meaningful benefits across their operations.
Tools and technologies for AI-ready data
The journey from raw data to AI-ready data involves various tools and technologies designed to streamline and enhance data preparation, management, and analysis. These tools and technologies play a crucial role in ensuring that data is accurate, complete, and formatted for optimal use in AI models. Here’s an overview of the key tools and technologies that support the development of AI-ready data:
Data integration tools
- ETL (Extract, Transform, Load) Tools: ETL tools such as Apache NiFi, Talend, and Informatica enable the extraction of data from various sources, its transformation into a usable format, and its loading into target systems. These tools help in consolidating data from multiple sources, ensuring consistency and accessibility for AI applications.
- Data pipelines: Tools like Apache Airflow and AWS Data Pipeline automate and orchestrate the flow of data across systems. They facilitate the continuous movement of data, ensuring that it is updated and synchronized for real-time or batch processing.
- Data virtualization tools: Tools like Denodo and Azure Data Factory allow for data virtualization, which provides a unified view of data from multiple sources without physically moving or copying it. This can simplify data access and reduce the overhead of data integration.
Data cleaning and preprocessing tools
- Data cleaning platforms: Tools such as Trifacta and DataRobot provide functionalities for cleaning and preprocessing data. They offer features for handling missing values, outlier detection, and data normalization, ensuring that data is accurate and ready for analysis.
- Python libraries: Libraries like Pandas, NumPy, and Scikit-learn are essential for data manipulation and preprocessing. These open-source libraries provide powerful functions for cleaning, transforming, and preparing data for AI models.
Data enrichment tools
- Data enrichment services: Platforms like Clearbit and ZoomInfo offer data enrichment services that augment existing data with additional context and features. These services can enhance customer profiles with demographic, firmographic, and behavioral data.
- External data sources: Integrating external data sources such as weather data, financial market data, and social media sentiment through APIs and data feeds can provide additional insights and context for AI models.
Data transformation and structuring tools
- Data transformation platforms: Tools like Apache Spark and Google Dataflow facilitate large-scale data transformation and processing. They support data conversion, aggregation, and structuring, enabling the preparation of data in formats suitable for AI analysis.
- Natural Language Processing (NLP) tools: For structuring unstructured data, NLP tools such as SpaCy and NLTK are used to convert text data into structured formats. These tools help in text mining, sentiment analysis, and feature extraction.
Data validation tools
- Data quality management tools: Platforms like Talend Data Quality and Ataccama provide functionalities for data validation, profiling, and quality monitoring. They help ensure that data meets the required accuracy, completeness, and consistency standards.
- Data profiling tools: Tools such as IBM InfoSphere and Microsoft SQL Server Data Quality Services offer data profiling capabilities to analyze data characteristics, identify potential issues, and ensure data integrity.
AI and machine learning platforms
- Machine Learning(ML) frameworks: Tools like TensorFlow, PyTorch, and Scikit-learn are widely used for developing and training AI models. They support the integration of AI-ready data into machine learning workflows, enabling sophisticated analysis and predictions.
- AutoML platforms: Automated Machine Learning (AutoML) platforms like Google AutoML and H2O.ai simplify the process of building and deploying AI models by automating tasks such as feature selection, model training, and hyperparameter tuning.
Data governance and security tools
- Data governance platforms: Tools such as Collibra and Alation provide functionalities for managing data policies, ensuring data lineage, and maintaining compliance with regulations. They support data governance practices and promote data stewardship.
- Data catalogs: Tools like Alation, Data.World, and Collibra offer data catalogs, which are crucial for discovering, understanding, and managing data assets within an organization. This is essential for making data accessible and understandable for AI projects.
- Data security solutions: Technologies like encryption, access controls, and anonymization tools safeguard data from unauthorized access and breaches. Solutions such as IBM Guardium and Microsoft Azure Security Center enhance data privacy and security.
Data observability tools
- Data observability tools: Tools like Monte Carlo, Datafold, and Dagster provide data observability, which focuses on monitoring data quality, detecting anomalies, and understanding data drift. This is important for ensuring AI model performance over time.
Feature stores
- Feature stores: Tools like Feast and Hopsworks offer feature stores, which are dedicated repositories for storing and managing features used in machine learning models. They help to streamline feature engineering, improve model reproducibility, and facilitate model training and deployment.
Model monitoring and explainability tools
- Model monitoring and explainability tools: Tools like EvidentlyAI and What-If Tool can help monitor the performance of AI models over time, detect data drift, and explain model predictions. This helps to ensure model accuracy and trustworthiness.
Data visualization tools
- Visualization platforms: Tools like Tableau, Power BI, and Looker enable the creation of interactive dashboards and reports. They help in visualizing AI-ready data, facilitating insights, and aiding decision-making processes.
These tools and technologies collectively support the transformation of raw data into AI-ready data, ensuring that it is clean, enriched, structured, and validated for effective use in AI models. Leveraging these tools can enhance the efficiency and effectiveness of data preparation processes, paving the way for successful AI implementations.
Best practices for optimizing data readiness for AI
Ensuring your data is AI-ready involves adopting a set of best practices that enhance its quality and suitability for AI applications. Implementing these practices will help organizations fully leverage AI technologies and drive successful outcomes. Here are key best practices to consider:
Formalize AI-ready data: Incorporate AI-ready data considerations into your overall data management strategy. This involves employing tools and methodologies such as active metadata management, data observability, and data fabric. Clearly define roles and responsibilities for managing data to ensure it supports AI applications effectively.
Adopt a data-centric approach: Acknowledge the critical role of high-quality, representative data in AI model performance. Ensure your data is diverse and reflective of the real-world scenarios the AI models will encounter. Additionally, promotes diversity among models and personnel to maintain AI values and uphold ethical standards.
Leverage data management expertise: Utilize DataOps and MLOps practices to support the entire AI lifecycle. Integrate data management requirements into the model deployment process and establish metrics for monitoring and governing data. This will help ensure that data management practices align with AI needs throughout the model’s lifecycle.
Enforce data fitness policies: Define and measure data standards to assess AI readiness, including aspects such as data lineage, quality, governance, versioning, and automated testing. Regularly validate data fitness to ensure it meets the necessary criteria for successful AI implementation and scaling.
Explore advanced data management tools: Investigate and adopt advanced data management tools that offer augmented capabilities and integrate seamlessly with AI technologies. Look for tools that support innovative approaches such as federated machine learning and retrieval-augmented generation (RAG) to enhance your data management practices.
By adhering to these best practices, organizations can ensure their data is well-prepared for AI applications, leading to more accurate, reliable, and effective AI outcomes.
How can LeewayHertz help you get AI-ready data?
LeewayHertz recognizes the critical role of high-quality data in achieving successful AI outcomes. We provide a comprehensive suite of services to ensure your data is prepared, organized, and ready for use by AI algorithms, maximizing the value of your AI initiatives.
Our AI-ready data services include:
- Data cleaning and preprocessing: We employ robust data cleaning techniques to identify and rectify errors, inconsistencies, and missing values within your datasets. Our expert team uses advanced algorithms and industry-standard practices to ensure data accuracy and reliability, providing a solid foundation for AI model training and analysis.
- Data structuring and transformation: We understand the importance of organized data for AI applications. Our specialists transform unstructured and semi-structured data into formats compatible with AI models. This involves organizing data into tables, databases, or other structured formats, ensuring consistent data representation and seamless integration with AI systems.
- Data labeling and annotation: We provide specialized data labeling and annotation services to enrich your datasets for AI model training. Our team carefully labels and annotates images, text, audio, and video data, enabling AI models to learn from labeled examples and perform tasks such as object recognition, sentiment analysis, and speech recognition with greater accuracy.
- Data security and privacy: We prioritize the security and privacy of your data, adhering to industry best practices and regulatory compliance requirements. We implement robust security measures to safeguard your data throughout the entire data preparation and AI development process, ensuring data confidentiality and integrity.
LeewayHertz is your trusted partner in achieving AI-ready data. We work collaboratively with our clients to understand their specific requirements and provide customized solutions that cater to their unique needs. Contact us today to discuss how we can help you unleash the power of your data and drive success in your AI journey.
Future trends and innovations in AI-ready data
As artificial intelligence continues to evolve, so does the landscape of AI-ready data. Emerging trends and innovations are shaping how data is prepared, managed, and utilized to maximize the potential of AI technologies. Here’s a look at some key future trends and innovations in AI-ready data:
Advanced data integration techniques: The future of AI-ready data will see increasingly sophisticated data integration methods. As organizations gather data from diverse sources, integrating these datasets effectively will become crucial. Advanced techniques such as data fusion, real-time data streaming, and cross-platform integration will enhance the ability to consolidate and harmonize data from various sources, improving its quality and usability for AI models.
Automated data preparation: Automation in data preparation is set to transform how organizations handle data readiness. Machine learning and AI technologies are increasingly being applied to automate data cleaning, transformation, and enrichment tasks. Automated tools will streamline these processes, reducing manual effort, minimizing errors, and accelerating the time required to prepare data for AI applications.
Enhanced data privacy and security: With growing concerns over data privacy and security, future innovations will focus on enhancing these aspects. Techniques such as federated learning, which allows models to be trained across decentralized data sources without sharing the data itself, will become more prevalent. Additionally, advances in encryption and anonymization technologies will help safeguard sensitive data while still enabling effective AI analysis.
Real-time data processing: Real-time data processing will become increasingly important as AI applications demand up-to-date information for accurate predictions and decisions. Innovations in edge computing and streaming analytics will enable organizations to process and analyze data in real-time, providing immediate insights and facilitating dynamic, data-driven decision-making.
Improved data quality frameworks: Future developments will bring more robust frameworks for ensuring data quality. New standards and methodologies will emerge to evaluate and enhance data accuracy, consistency, and completeness. These frameworks will help organizations maintain high data quality throughout its lifecycle, ensuring that AI models are built on a solid foundation.
Enhanced data annotation and labeling: As AI models become more sophisticated, the need for high-quality annotated and labeled data will grow. Innovations in data annotation, such as semi-supervised learning and crowdsourced labeling, will improve the efficiency and accuracy of data labeling processes. These advancements will support the development of more effective and reliable AI models.
Greater focus on data ethics and governance: The ethical use of data will continue to be a major focus, with advancements in data governance and ethical frameworks. Organizations will implement more comprehensive data governance policies to address issues related to fairness, transparency, and accountability. Innovations in ethical AI practices will ensure that data is used responsibly and in compliance with regulatory standards.
Integration of AI-driven data management: AI-driven data management solutions will play a key role in the future of AI-ready data. AI technologies will be used to optimize data storage, retrieval, and management processes, enabling more efficient data handling. These solutions will enhance data governance, automate data management tasks, and improve overall data quality.
Expansion of synthetic data: Synthetic data, generated using algorithms to mimic real-world data, will gain prominence as a tool for AI development. This approach allows for the creation of large, diverse datasets that can be used to train AI models without relying solely on real-world data. Innovations in synthetic data generation will provide additional flexibility and scalability for AI applications.
Evolution of data collaboration platforms: Future trends will include the development of advanced data collaboration platforms that facilitate secure and efficient data sharing across organizations and industries. These platforms will enable seamless collaboration while maintaining data integrity and privacy, supporting more comprehensive and effective AI solutions.
The future of AI-ready data is characterized by advancements in integration, automation, privacy, and real-time processing. These innovations will enhance the quality and usability of data, driving more effective and ethical AI applications and contributing to the continued evolution of artificial intelligence technologies.
Endnote
In conclusion, AI-ready data is fundamental to unlocking the full potential of artificial intelligence. As organizations increasingly rely on AI technologies, the quality and preparedness of their data become crucial. Ensuring that data is clean, enriched, transformed, and validated is essential for building accurate and reliable AI models.
The benefits of AI-ready data are evident in its ability to drive better decision-making, improve operational efficiency, and enhance predictive accuracy. By addressing common roadblocks like structural errors, data silos, and outdated information, organizations can ensure their data is well-suited for AI applications. Implementing best practices—such as formalizing data management strategies, adopting a data-centric approach, leveraging expertise, and enforcing data fitness policies—can significantly enhance the effectiveness of AI initiatives.
Looking ahead, the landscape of AI-ready data will continue to evolve with advancements in technology and new innovations. Embracing emerging trends like data democratization, intelligent data governance, and the integration of structured and unstructured data will be key to staying ahead. As organizations navigate these changes, maintaining a focus on data quality and alignment with AI requirements will be crucial.
Ultimately, the journey toward AI readiness is ongoing. By continuously refining data management practices and staying informed about new developments, organizations can better harness the power of AI to drive meaningful outcomes and maintain a competitive edge in the evolving digital landscape.
Ready to make your data AI-ready and drive transformative results? Connect with LeewayHertz’s AI experts to explore how our advanced solutions can help you optimize your data for successful AI implementation.
Start a conversation by filling the form
All information will be kept confidential.
Insights
AI in trend analysis: Predicting the pulse of the market
In today’s fast-paced, data-driven world, deciphering patterns and trends is more crucial than ever, serving as a compass for organizations and individuals navigating the extensive seas of information.
Building smarter classrooms: The benefits and use cases of AI in education
AI can monitor online exams to prevent cheating and ensure that exams are conducted fairly to reduce the workload on teachers while providing a more secure testing environment for students.
AI in banking and finance: Use cases, applications, AI agents, solutions and implementation
AI in banking and finance offers various opportunities for process optimization, risk management, and customer engagement. One of the key areas where AI demonstrates its potential is in data analysis.