Composite AI: Benefits, applications, implementation strategies, best practices, and future prospects
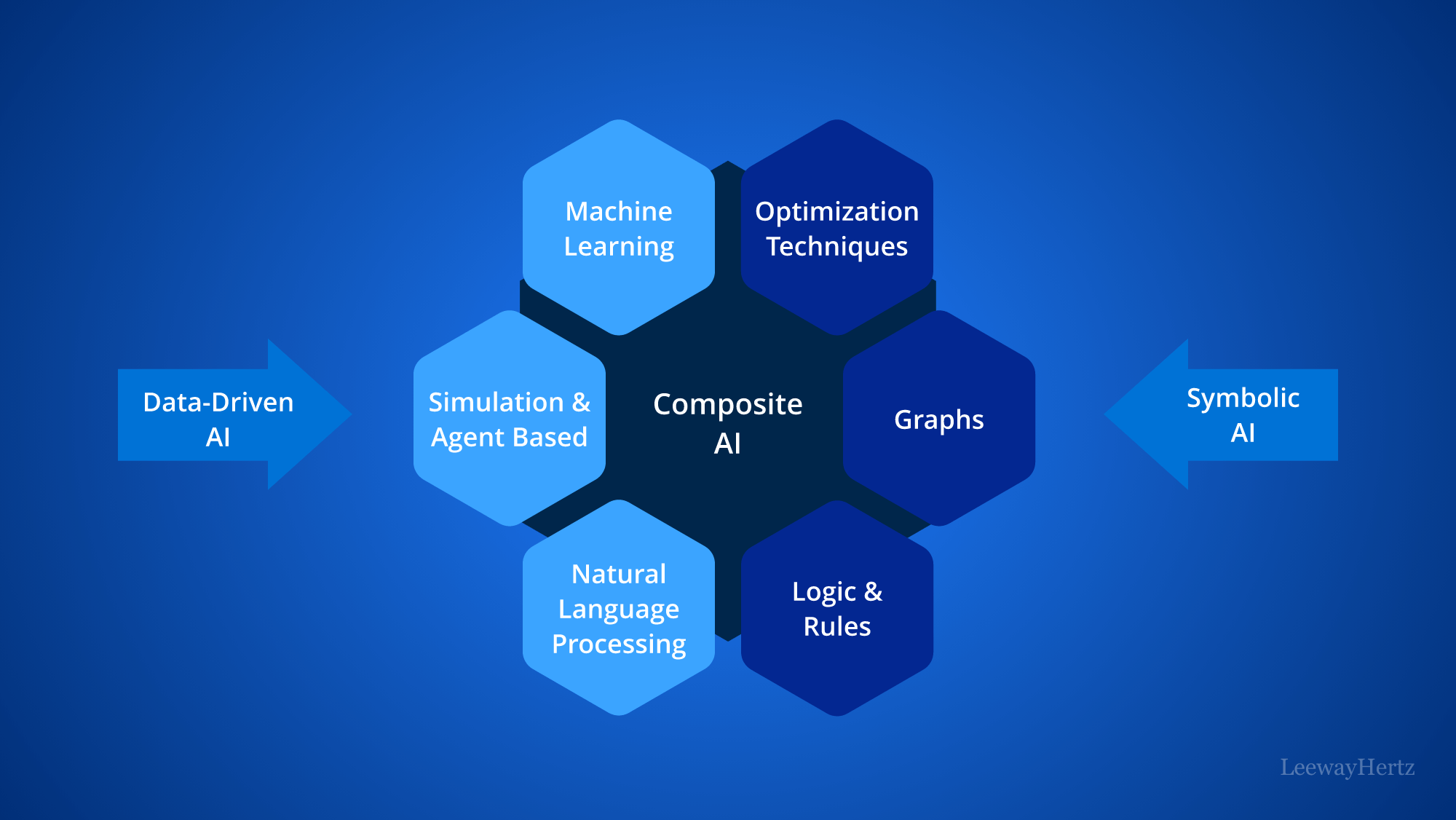
Composite AI is rapidly gaining attention as a transformative approach that combines various artificial intelligence techniques to tackle complex challenges. Unlike traditional AI, which often relies on a single methodology, composite AI integrates multiple AI strategies—such as machine learning, natural language processing, and computer vision—into a cohesive system. This integrated approach enhances problem-solving capabilities, making it possible to address more intricate issues with greater precision.
The market for composite AI is growing swiftly, with industry estimates projecting its value from USD 0.9 billion in 2023 to reach USD 4.4 billion by 2028, at a CAGR of 36.5% during the forecast period. This substantial growth highlights the increasing recognition of composite AI’s potential across sectors like healthcare, finance, and manufacturing. For example, in healthcare, composite AI is being used to improve diagnostics by combining imaging analysis with patient data, leading to more accurate and timely treatment decisions.
As organizations and industries seek to leverage composite AI’s benefits, it’s crucial to understand both its potential and the challenges it presents. This article explores what composite AI is and how it integrates different AI techniques. It compares composite AI with other AI approaches, examines its applications across various industries, and discusses the advantages it offers. Additionally, the article delves into best practices for implementing composite AI, addresses ethical considerations, and looks at its future developments. By providing a comprehensive overview, the article aims to equip readers with a clear understanding of composite AI and its impact on the evolving AI landscape.
- What is composite AI?
- Why is composite AI important now?
- Comparison of composite AI with other AI approaches
- Applications of composite AI across industries
- Advantages of composite AI
- Implementing composite AI: A step-by-step approach
- How can LeewayHertz help implement composite AI?
- Ethical considerations in composite AI: Navigating the complex landscape
- Addressing the limitations or potential drawbacks of composite AI
- The future of composite AI: Beyond the horizon
What is composite AI?
Composite AI refers to an advanced AI approach that integrates multiple Artificial Intelligence (AI) technologies to create a more sophisticated, flexible, and intelligent system. Instead of relying on a single AI technique, composite AI combines various methods to enhance performance and address complex problems more effectively.
Key components of composite AI
- Machine Learning (ML): Machine Learning is a core component of composite AI, involving algorithms that learn from data to make predictions or decisions. ML techniques such as supervised learning, unsupervised learning, and reinforcement learning contribute to pattern recognition and predictive analytics.
- Deep learning: Deep learning, a subset of ML, uses neural networks with multiple layers to model complex data patterns. It excels in tasks involving large volumes of unstructured data, such as image and speech recognition, making it integral to composite AI systems that handle diverse data types.
- Simulation and agent-based technique: Simulation and agent-based techniques allow for the modeling and testing of complex systems and behaviors within composite AI. These components help in understanding and predicting the dynamics of interconnected agents and environments.
- Natural Language Processing (NLP): NLP enables machines to understand, interpret, and generate human language. By integrating NLP, Composite AI systems can process textual information, perform sentiment analysis, and facilitate interactions through chatbots and virtual assistants.
- Computer vision: Computer vision allows systems to interpret visual data from the world, such as recognizing objects and understanding scenes. This component is essential for applications in areas like medical imaging, autonomous vehicles, and surveillance.
- Graphs: Graph-based techniques and structures, such as knowledge graphs and ontologies, provide a structured representation of information and the relationships between entities. These components enhance the contextual understanding and reasoning capabilities of composite AI systems.
- Optimization techniques: Optimization algorithms and techniques are used to find the best solutions or configurations within composite AI systems. They contribute to decision-making, resource allocation, and the overall performance and efficiency of AI systems.
- Symbolic AI: Symbolic AI focuses on the representation and manipulation of symbolic knowledge, complementing the data-driven and statistical approaches in composite AI. It helps in tasks like knowledge representation, reasoning, and explainable AI.
- Logic and rules: Logic and rule-based approaches in composite AI involve the use of formal logic, reasoning, and rule-based systems to encode domain knowledge and decision-making processes.
- Robotics and automation: Robotics and automation involve AI technologies that control physical machines and processes. In composite AI, these components enable the automation of tasks and interactions with the physical environment, enhancing system capabilities in real-world applications.
- Data fusion and integration: Data fusion combines information from multiple sources and modalities to create a unified view. In composite AI, this process integrates structured and unstructured data, providing a more comprehensive understanding and improving decision-making.
- Decision-making algorithms: Decision-making algorithms process integrated data and insights to make informed decisions. These algorithms use reasoning, optimization, and predictive modeling to enhance decision-making processes in composite AI systems.
Key aspects of composite AI
- Multi-technology integration: Composite AI leverages the strengths of various AI technologies, combining them to address complex tasks that individual technologies might struggle with. This integration allows for more robust and versatile AI solutions.
- Enhanced data processing: The ability to handle and synthesize data from diverse sources is a crucial aspect of composite AI. By integrating data fusion and advanced analytics, composite AI systems can provide more accurate and actionable insights.
- Contextual understanding: Composite AI systems can achieve a deeper level of contextual understanding by integrating knowledge graphs and NLP. This capability allows them to interpret complex scenarios and make decisions based on a richer understanding of the context.
- Scalability and flexibility: The modular nature of composite AI allows for scalable and adaptable solutions. Organizations can customize and expand their AI systems by incorporating new technologies and methods as needed, ensuring that the system evolves with changing requirements.
- Interdisciplinary approach: Composite AI represents an interdisciplinary approach, combining insights from various fields such as computer science, data science, and domain-specific knowledge. This holistic perspective enables the development of more comprehensive AI solutions.
By focusing on these key components and aspects, composite AI offers a sophisticated framework for addressing complex challenges and delivering advanced capabilities across a range of applications.
Optimize Your Operations With AI Agents
Optimize your workflows with ZBrain AI agents that automate tasks and empower smarter, data-driven decisions.
Why is composite AI important now?
The rise of AI adoption in business is reshaping industries, but traditional single-method approaches often fall short when addressing intricate and multifaceted problems. Additionally, many successful AI implementations rely on extensive datasets, which are not always readily available or accessible.
Composite AI addresses these limitations by integrating various AI technologies into a cohesive framework. This unified approach offers several critical advantages:
- Facilitating human-like decision-making: Composite AI combines different analytical methods to mimic complex human decision-making processes. This capability allows systems to tackle nuanced and intricate problems with greater accuracy and relevance, enhancing the quality of decisions and outcomes.
- Reducing the need for large data science teams: By leveraging multiple AI techniques in a cohesive manner, composite AI can streamline processes and reduce the dependency on large data science teams. This efficiency helps organizations optimize their resources and focus on strategic initiatives rather than extensive data preparation and analysis.
- Extracting insights from smaller datasets: Composite AI’s ability to integrate various data sources and techniques means that it can derive valuable insights even from smaller or less comprehensive datasets. This flexibility allows businesses to make informed decisions without requiring massive volumes of data.
These benefits highlight why Gartner has recognized composite AI as one of the top emerging technologies with substantial potential to impact business operations in the coming years. Its ability to address complex challenges, optimize resource usage, and provide actionable insights from limited data makes composite AI a critical asset in today’s rapidly evolving technological landscape.
Comparison of composite AI with other AI approaches
Here’s a comparison of composite AI with other AI approaches:
Aspect | Composite AI | Traditional AI | Generative AI |
---|---|---|---|
Definition | Combines multiple AI techniques to leverage their strengths and address complex problems | Utilizes single AI techniques to solve specific problems | Generates new content based on patterns learned from existing data |
Approach | Integrative approach using multiple models or techniques | Single-model approach, often rule-based or statistical | Creates new data or content by understanding patterns in existing data |
Flexibility | Highly flexible, can adapt to various problems by integrating different methods | Less flexible, limited to the capabilities of one technique | Flexible in generating content, but less focused on solving specific tasks |
Complexity | Can be complex due to the integration of multiple AI techniques | Generally less complex, focused on specific tasks | Can be complex, especially with deep learning models |
Applications | Suitable for complex and multi-faceted problems across different domains | Best for specific, well-defined problems | Ideal for creative tasks like text generation, image creation |
Data requirements | Requires diverse data to train and integrate multiple models | Often requires less diverse data, focused on a specific task | Requires large datasets for training models to generate new content |
Performance | Can achieve high performance by leveraging strengths of different techniques | Performance is limited to the capabilities of the single technique used | Performance depends on the quality of the training data and model architecture |
Scalability | Scalable by adding or integrating new techniques as needed | Scalability depends on the specific technique used | Scalable in generating content but may require significant computational resources |
Cost and resource usage | Potentially higher cost and resource usage due to multiple models | Generally lower cost and resource usage, focused on one model | Can be high, especially with large models and extensive training data |
Interpretability | Can be complex to interpret due to multiple models and their interactions | Often more interpretable if based on simpler models | Typically less interpretable due to the nature of generative models |
Optimize Your Operations With AI Agents
Optimize your workflows with ZBrain AI agents that automate tasks and empower smarter, data-driven decisions.
Applications of composite AI across industries
Composite AI is rapidly finding its way into various industries, transforming processes and driving innovation. Here are some of the most prominent applications across different sectors:
- Healthcare:
- Precision medicine and personalized treatment plans: By combining genomic data analysis with medical records, lifestyle information, and even wearable sensor data, composite AI can create truly personalized treatment plans. This involves identifying individual genetic predispositions, predicting potential drug responses, and even recommending specific lifestyle interventions based on individual needs. This goes beyond simply tailoring treatment to specific diseases, but rather tailoring it to the unique characteristics of each individual patient.
- AI-powered disease prediction and early detection: Beyond pattern recognition, composite AI can leverage predictive modeling to identify individuals at high risk for specific diseases. This involves analyzing risk factors, medical history, genetic information, and even lifestyle data to predict the likelihood of developing a disease. This allows for proactive interventions, early detection, and personalized prevention strategies that could dramatically improve health outcomes.
- Accelerated drug discovery and development: Composite AI can transform drug discovery by not only analyzing vast chemical databases but also by simulating the behavior of drug candidates in the body. This involves combining machine learning algorithms with molecular modeling and simulation techniques to predict drug efficacy, identify potential side effects, and optimize drug formulations, leading to faster and more cost-effective drug development processes.
- Enhanced medical imaging analysis and diagnosis: AI can go beyond simply analyzing medical images; it can also learn from previous diagnoses and even provide insights that human experts can miss. This involves combining deep learning algorithms with expert systems, which can be customized to specific specialties like radiology or pathology. This results in more accurate and efficient diagnoses, potentially leading to earlier detection of diseases and improved treatment outcomes.
- Finance:
- Advanced fraud detection and prevention: By combining transactional data analysis with behavioral analytics, social media sentiment analysis, and even network analysis, composite AI can create a comprehensive fraud detection system. It can not only identify suspicious transactions in real time but also proactively identify potential fraudsters and predict emerging fraud patterns. This allows for more proactive fraud prevention measures and reduces financial losses.
- Hyper-personalized credit risk assessment: By integrating data from traditional credit scoring models with alternative data sources like social media activity, spending patterns, and even online behavior, composite AI can develop a more nuanced and accurate picture of individual creditworthiness. This can lead to more personalized loan offers, tailored interest rates, and improved access to financial services for previously underserved populations.
- AI-driven investment strategies and portfolio management: Composite AI can develop sophisticated investment strategies by combining machine learning algorithms for analyzing market data and predicting market trends with expert systems for incorporating financial knowledge and risk management strategies. This can involve identifying hidden investment opportunities, optimizing portfolio allocation, and even predicting market volatility, enabling investors to make more informed decisions and potentially achieve better returns.
- Personalized financial planning and wealth management: Composite AI can create personalized financial plans by combining financial data analysis with natural language processing to understand customer goals and aspirations. This could involve providing tailored recommendations for savings, investments, retirement planning, insurance, and even debt management, tailored to individual circumstances and risk tolerance.
- Manufacturing:
- Predictive maintenance and asset performance management: By combining sensor data analysis with machine learning algorithms, composite AI can predict equipment failures with greater accuracy and analyze historical data to identify root causes of failures. This enables more proactive maintenance, not just preventing breakdowns but also improving equipment lifespan and reducing overall maintenance costs. It also allows for predictive asset management, where manufacturers can optimize the use of their assets to maximize productivity and minimize downtime.
- AI-driven quality control and defect detection: AI can go beyond simply detecting defects; it can be used to identify the causes of defects. By analyzing real-time data from production lines, integrating with quality control systems, and leveraging machine learning for predictive modeling, composite AI can pinpoint the root causes of defects, leading to process improvements that prevent future issues. This also enables the development of self-learning quality control systems that constantly adapt and improve their accuracy.
- Autonomous robotics and collaborative automation: By combining machine learning for perception, rule-based systems for task execution, and natural language processing for human-robot interaction, composite AI can create highly flexible and adaptable robots. These robots can work alongside humans, learn new tasks, adapt to changing environments, and even collaborate with other machines to optimize production processes. This leads to increased automation, enhanced safety, and improved productivity.
- Smart factories and Industry 4.0: Composite AI is the driving force behind the smart factory concept, where data from sensors, machines, and even production processes is integrated to create a connected and intelligent manufacturing environment. This enables real-time monitoring, predictive analytics, automated decision-making, and seamless integration across the entire production lifecycle.
- Retail:
- Hyper-personalized shopping experiences: Composite AI can combine data from customer purchase history, browsing behavior, website interactions, social media activity, and even location data to create truly hyper-personalized shopping experiences. This could involve recommending products based on individual preferences, offering personalized discounts, and even tailoring website layouts and product displays to individual customer profiles.
- Predictive demand forecasting and inventory optimization: Composite AI can create highly accurate demand forecasts by combining data from sales history, seasonality trends, social media sentiment, and even weather forecasts. This allows retailers to optimize inventory levels, minimize stockouts and overstocking, and even predict potential supply chain disruptions.
- AI-driven customer engagement and marketing: Beyond simple targeted advertising, composite AI can enable personalized and interactive marketing campaigns. This could involve using chatbots to engage customers in real time, providing personalized recommendations within chat, and even suggesting products based on real-time browsing behavior.
- Omnichannel customer support and assistance: Composite AI can power omnichannel customer support systems by combining NLP to understand customer inquiries, machine learning to predict customer needs, and knowledge graphs to access product information. This could involve AI-powered chatbots for initial interactions, virtual assistants for more complex inquiries, and even automated self-service tools for common requests.
- Smart store design and customer journey optimization: By analyzing customer behavior data within stores, composite AI can optimize store layouts, product placement, and even the flow of customers through the store. This can improve the overall shopping experience, increase impulse purchases, and reduce wait times.
- Transportation and logistics:
- Dynamic traffic management and routing: By combining real-time traffic data, weather forecasts, and predictive models, a system leveraging composite AI principles can provide real-time recommendations for traffic signal adjustments, vehicle rerouting, and personalized navigation, leading to a more efficient and resilient transportation network. This optimizes traffic flow, reduces congestion, and minimizes travel times.
- Collaborative autonomous transportation: Beyond individual autonomy, composite AI can orchestrate a future of collaborative transportation systems. By enabling vehicles to communicate with each other and the infrastructure (V2X), AI can optimize traffic flow in real-time, enhance safety by anticipating and mitigating hazards, and pave the way for innovative mobility services like autonomous fleets. This could include fleets of autonomous delivery trucks, public transportation systems, and even air taxis.
- Predictive maintenance and fleet optimization: By analyzing sensor data from vehicles, incorporating historical maintenance records, and leveraging machine learning for predicting failures, composite AI can optimize maintenance schedules, reducing downtime, minimizing costs, and even improving vehicle performance. This also extends to fleet optimization, where AI analyzes driver behavior, route efficiency, and fuel consumption to optimize logistics and reduce emissions.
- Smart logistics and supply chain management: Composite AI can combine AI for demand forecasting, inventory management, and route optimization to create intelligent supply chain systems that optimize delivery schedules, predict disruptions, and manage inventory levels proactively. This can leverage data from sensors, weather forecasts, and even social media trends to anticipate demand fluctuations and adjust logistics in real time.
- Personalized mobility services: By integrating data from personal preferences, real-time traffic conditions, and available transportation options, composite AI can personalize mobility services for individuals. This could involve suggesting optimal travel routes, booking rides, or even providing tailored recommendations for carsharing, ride-hailing, or public transportation based on individual needs and preferences.
- Education:
- Adaptive learning platforms: Adaptive learning platforms use composite AI by integrating machine learning algorithms to predict student performance. They also use natural language processing to understand student responses and knowledge graphs to map learning pathways. This combination allows composite AI to create platforms that adjust the difficulty, pace, and content of learning materials in real-time, tailored to each student’s individual needs.
- Personalized tutoring and feedback: Composite AI can power virtual tutors that provide customized feedback, answer student questions, and even offer targeted recommendations for improvement. This could blend machine learning for analyzing student work, NLP for understanding questions and responses, and expert systems for providing tailored guidance.
- Early intervention and remediation: Using machine learning to detect early signs of learning difficulties, and NLP to analyze student writing and speech patterns, composite AI can identify students who need additional support. This enables timely intervention through personalized learning strategies, remedial activities, and even targeted mentorship programs.
- Predictive analytics for learning outcomes: Composite AI can analyze student data, historical performance, and even external factors like socio-economic background to predict future academic success and identify areas where interventions might be most effective. This allows educators to tailor educational resources and support strategies to individual students.
- AI-assisted teacher training: Composite AI can provide teachers with personalized professional development by analyzing teaching practices, identifying areas for improvement, and offering targeted training modules. This could combine machine learning for analyzing classroom data, NLP for understanding teacher feedback, and expert systems for providing customized training recommendations.
7. Insurance:
- Dynamic risk assessment and pricing: By combining machine learning to analyze vast data sets with expert systems to incorporate actuarial knowledge, composite AI can dynamically assess risk profiles, factoring in real-time data like driving behavior (from telematics), health monitoring (from wearables), and even social media interactions. This enables more accurate and personalized pricing, potentially leading to lower premiums for low-risk individuals.
- Intelligent claims processing: Composite AI combines NLP to understand claim narratives, machine learning to detect fraudulent patterns, and rule-based systems to automate claims processing. This can streamline the claims process while identifying potential fraud early on. This could include automatically verifying information, assessing damage based on photos and sensor data, and recommending appropriate payouts.
- Personalized policy design and recommendation: By analyzing customer data, risk profiles, and market trends, composite AI can recommend customized policies that truly meet individual needs, including coverage levels, pricing, and even optional add-ons. This can leverage NLP for understanding customer preferences, machine learning for identifying risk factors, and expert systems for tailoring policies to legal requirements.
- Proactive risk management and prevention: Composite AI can help insurers identify potential risks before they occur by analyzing data from wearables, smart homes, and even environmental sensors. This allows them to proactively engage with customers, offer tailored risk mitigation strategies, and even offer personalized discounts for engaging in healthy behaviors.
- Legal:
- Automated legal document generation: Combining natural language processing (NLP) with knowledge graphs and rule-based systems, composite AI can automatically generate legal documents like contracts, wills, and pleadings based on specific inputs and legal templates. This reduces the time and cost associated with drafting documents and minimizes errors.
- Intelligent legal assistants: Composite AI can integrate NLP for language understanding, machine learning for legal research, and expert systems for legal reasoning to create intelligent legal assistants that can help lawyers with tasks like case analysis, research, and document drafting. These assistants can also identify potential legal issues and suggest strategic solutions.
- Predictive legal analytics: By combining machine learning for predicting case outcomes with expert systems for legal reasoning, composite AI can help lawyers make more informed decisions regarding litigation strategy and settlement negotiations. It can analyze historical data, legal precedent, and even social media sentiment to predict the likelihood of success or failure in a given case.
- Real estate:
- Investment analysis: Composite AI can help investors identify promising real estate opportunities by leveraging various technologies. By using machine learning algorithms to analyze market data, trends, and historical performance, along with predictive analytics to forecast future performance, investors can make more informed decisions. Additionally, natural language processing (NLP) can be employed to analyze unstructured data, such as news articles and social media, providing a more comprehensive understanding of market dynamics.
- Hyper-personalized property recommendations: Composite AI can analyze a buyer’s preferences, financial situation, lifestyle, and market trends to recommend properties tailored to their unique needs, going beyond traditional search criteria. This could combine NLP for understanding preferences in natural language, machine learning for predicting future market trends, and expert systems to incorporate specific regulations and local market knowledge.
- Dynamic pricing and negotiation support: By combining real-time market data analysis with predictive models, composite AI can provide sellers with dynamic pricing recommendations and assist buyers in negotiating optimal prices, leveraging data from past transactions, market trends, and even social media sentiment to inform pricing strategies.
- Smart home management and maintenance: Composite AI can automate tasks like scheduling maintenance based on predictive models of equipment lifespan, monitoring energy consumption for efficiency, and even using voice assistants for tenant requests. This would blend sensor data analysis, machine learning for predicting equipment failures, and NLP for understanding tenant requests.
This is not an exhaustive list, but it demonstrates the vast potential of composite AI across various industries. As AI technology continues to advance, we can expect to see even more innovative applications emerging in the years to come.
Optimize Your Operations With AI Agents
Optimize your workflows with ZBrain AI agents that automate tasks and empower smarter, data-driven decisions.
Advantages of composite AI
Composite AI offers several significant benefits by integrating various AI techniques and models. These advantages enhance its effectiveness and versatility across different applications and industries. Here’s a detailed look at the key advantages of composite AI:
1. Enhanced accuracy and precision
- Integration of diverse techniques: By combining multiple AI methods, such as machine learning, natural language processing (NLP), and computer vision, composite AI can leverage the strengths of each technique to improve overall accuracy and precision. This integration helps in addressing complex problems more effectively than using a single AI technique alone.
- Improved decision-making: Composite AI provides more accurate predictions and insights by integrating data from various sources. This holistic view enhances decision-making, as it incorporates a broader range of information and analytical perspectives.
2. Flexibility and adaptability
- Versatility in applications: Composite AI can be applied to a wide range of use cases across different industries. Its ability to combine different AI techniques allows it to adapt to diverse scenarios and requirements, making it a flexible solution for various challenges.
- Scalability: The modular nature of composite AI enables it to scale by adding or integrating new techniques as needed. This adaptability ensures that the AI system remains relevant and effective as new technologies and methodologies emerge.
3. Improved performance and efficiency
- Optimized solutions: By integrating multiple AI models, composite AI can optimize solutions for complex problems. This optimization improves performance in areas such as predictive analytics, data processing, and automation, leading to more efficient operations.
- Enhanced speed: Composite AI can process and analyze large volumes of data more quickly by leveraging the strengths of different AI techniques. This increased speed accelerates insights and decision-making processes, leading to faster and more effective outcomes.
4. Better handling of complex problems
- Comprehensive analysis: Composite AI’s ability to integrate various analytical approaches enables it to handle complex problems that require a multi-faceted analysis. This comprehensive approach addresses problems from multiple angles, providing a more thorough understanding and solution.
- Enhanced problem solving: By combining techniques such as deep learning, symbolic AI, and optimization algorithms, composite AI can tackle problems that are beyond the capabilities of single-method AI systems. This integration leads to more robust and effective solutions.
5. Increased innovation and creativity
- Fostering innovation: The integration of different AI techniques encourages innovation by combining diverse approaches and methodologies. This fosters creativity in developing new solutions and applications, driving advancements in technology and industry practices.
- New use cases: Composite AI opens up new possibilities for applications that may not be achievable with traditional AI methods. Its versatility and adaptability lead to the exploration of novel use cases and innovative solutions.
6. Enhanced data utilization
- Holistic data integration: Composite AI integrates data from various sources and formats, including structured and unstructured data. This holistic approach ensures that all relevant data is utilized, leading to more comprehensive and accurate analyses.
- Improved data insights: By combining different analytical techniques, composite AI can uncover deeper insights from data. This enhanced data utilization provides more valuable information for decision-making and strategic planning.
7. Cost efficiency
- Optimized resource use: Composite AI can optimize resource allocation by integrating different AI models and techniques. This optimization reduces the need for separate systems and processes, leading to cost savings and improved efficiency.
- Reduced development time: Composite AI can accelerate the development process by using preexisting models and techniques. This reduction in development time translates to cost savings and quicker solution implementation.
8. Enhanced user experience
- Personalization: Composite AI’s ability to integrate various techniques allows for more personalized interactions and recommendations. This personalization improves user experience by tailoring solutions and services to individual preferences and needs.
- Improved interaction quality: By combining NLP with other AI techniques, composite AI enhances the quality of interactions, such as customer support and virtual assistants. This improvement leads to more effective and satisfying user experiences.
9. Robustness and reliability
- Error mitigation: The integration of multiple AI techniques helps in mitigating errors and reducing the likelihood of failures. By leveraging different approaches, composite AI can cross-verify results and ensure greater reliability in its outputs.
- Resilience: Composite AI’s ability to integrate diverse models and techniques makes it more resilient to variations and uncertainties. This resilience enhances its performance and reliability across different scenarios and applications.
These advantages highlight how composite AI can provide more effective, adaptable, and innovative solutions compared to single-method AI approaches. By leveraging the strengths of various AI techniques, composite AI enhances performance, accuracy, and user experience across diverse applications and industries.
Implementing composite AI: A step-by-step approach
While the concept of composite AI is compelling, its true potential lies in its real-world applications. This section explores the practical steps involved in implementing composite AI, guiding you through the journey from problem definition to deployment.
- Understanding the requirements: The first step in implementing composite AI is to clearly define your problem or task. Ask yourself:
- What are the desired outcomes? What specific business goals are you trying to achieve? (e.g., improved customer experience, reduced fraud, faster product development)
- What data is available? What data sources do you have access to, and what are their limitations? (e.g., structured databases, unstructured text, sensor data, image files)
- What are the constraints? What factors might influence your choices? (e.g., budget, time, computational resources, ethical considerations)
By meticulously analyzing these factors, you’ll have a solid foundation for selecting the right AI techniques and designing your composite AI system.
- Assembling a cross-functional team: Implementing composite AI requires a diverse team of experts, including data scientists, machine learning engineers, domain experts, and business stakeholders. This cross-functional collaboration is crucial for aligning the composite AI implementation with your organization’s strategic objectives and ensuring a holistic approach to problem-solving.
- Choosing the right AI techniques: There’s no one-size-fits-all approach to choosing AI techniques. The ideal combination depends on your specific goals, data characteristics, and desired outcomes.
Consider these factors:
- Problem complexity: For highly complex problems with intricate decision-making processes, you might consider a combination of machine learning, symbolic reasoning, and reinforcement learning.
- Data availability: If you have limited or incomplete data, techniques like transfer learning or few-shot learning can be valuable additions.
- Desired outcomes: For tasks that require real-time prediction, you might prioritize techniques like deep learning or time series analysis. For tasks focused on understanding human language, natural language processing (NLP) would be essential.
- Designing the composite AI architecture: The architecture defines the structure and flow of your composite AI system. Here are three common patterns:
- Parallel architecture: Different AI techniques operate independently, and their results are combined to make a final decision. This architecture is suitable for tasks that benefit from diverse perspectives, such as analyzing different data sources or incorporating multiple factors into a decision.
- Example: A fraud detection system might combine a machine learning model for analyzing transaction patterns with a rule-based system for detecting known fraudulent behaviors.
- Hierarchical architecture: AI techniques are arranged in a hierarchy, with simpler techniques feeding into more complex ones. This allows you to build sophisticated systems gradually, starting with basic tasks and then building upon them.
- Example: An image recognition system might use a basic image processing technique to extract features, which are then fed into a deep learning model for classification.
- Ensemble architecture: Multiple instances of the same AI technique are trained on different subsets of data, and their predictions are combined. This can enhance accuracy and robustness by leveraging the strengths of multiple models.
- Example: A credit scoring model might combine multiple machine learning models trained on different credit risk factors to provide a more comprehensive and reliable score.
Choosing the right architecture depends on factors such as data flow, complexity of the problem, and available resources.
- Data preparation and integration: Composite AI systems often rely on diverse data sources. Effective data preparation is critical for successful implementation. This includes:
- Preprocessing: Handling missing values, converting data types, and normalizing data to ensure consistency across different sources.
- Cleaning: Removing inconsistencies, duplicates, and outliers that can skew your analysis.
- Feature engineering: Creating new features from existing data to improve the performance of your AI models. This can involve combining features, creating interactions, or transforming data into a format more suitable for machine learning.
- Integration: Combining data from multiple sources into a unified format that can be used by your composite AI system. This might involve creating data pipelines, using ETL processes, or storing data in a data lake architecture.
- Training and optimization: Fine-tuning your models
Training your AI models is crucial for achieving optimal performance. Here are some essential steps:
- Individual training: Train each AI technique separately using the appropriate algorithms and data sets. This allows you to evaluate the individual performance of each model.
- Joint training: In some cases, you can train the AI components together to optimize their interaction, which can improve the composite system’s overall performance.
- Hyperparameter tuning: Fine-tune the parameters of your AI models to achieve optimal performance based on your chosen evaluation metrics. This is an iterative process that often involves experimenting with different parameter combinations.
- Deployment and monitoring: Once your composite AI system is trained and optimized, you need to deploy it in a real-world environment. Here are some key considerations:
- Infrastructure selection: Choose the right hardware and software infrastructure to support your system, considering factors such as computational requirements, data storage needs, and scalability. Cloud platforms like AWS, Azure, and Google Cloud are often ideal for deploying composite AI systems.
- Scalability: Ensure that your system can handle increasing data volumes and workloads as your business grows. Consider using containerization and distributed computing technologies to handle large-scale deployments.
- Security: Implement robust security measures to protect your system and data from unauthorized access or malicious attacks. Use encryption, access control mechanisms, and regular security audits.
- Monitoring: Continuously monitor your system’s performance to identify potential issues. Track metrics like accuracy, latency, resource utilization, and data drift to ensure optimal performance and identify any required adjustments.
- Governance and risk management: Establishing clear governance frameworks is crucial for the responsible development and deployment of composite AI. This includes defining guidelines, policies, and roles and responsibilities to address potential risks, such as data privacy, algorithmic bias, and system failures.
- Iterative development and continuous improvement: Adopting an iterative, agile approach to composite AI implementation is essential. Continuously evaluating the system’s performance based on real-world feedback and data and making necessary adjustments can lead to better adaptation and optimization over time.
Tools and platforms:
Numerous tools and platforms can facilitate the implementation of composite AI.
- Open-source libraries: Libraries like TensorFlow, PyTorch, and Scikit-learn offer powerful tools for building AI components.
- Cloud platforms: Cloud services like AWS, Azure, and Google Cloud provide infrastructure and tools for developing and deploying Composite AI systems.
- Software packages: Packages like MLflow and Kubeflow offer pre-built tools for implementing and deploying AI models, including those for composite AI.
Addressing challenges and best practices
Implementing composite AI presents unique challenges:
Data complexity: Handling diverse data sources and formats, ensuring consistent data quality, and integrating data from different systems.
Integration issues: Ensuring seamless data flow between different AI techniques and managing the interactions between various models.
Performance optimization: Achieving optimal performance while managing resource constraints and balancing the trade-offs between accuracy, efficiency, and latency.
Best practices:
- Start small: Begin with a simple composite AI system and gradually increase complexity. This allows you to test and validate your approach before scaling up.
- Iterative development: Continuously evaluate and optimize your system based on real-world data and feedback. This ensures that your system adapts and improves over time.
- Collaboration: Foster collaboration between experts in different AI disciplines, including data scientists, machine learning engineers, and domain experts.
By following these steps and addressing the challenges, you can successfully implement composite AI systems that deliver tangible benefits across industries.
Optimize Your Operations With AI Agents
Optimize your workflows with ZBrain AI agents that automate tasks and empower smarter, data-driven decisions.
How can LeewayHertz help implement composite AI?
LeewayHertz is a leading technology solutions provider specializing in artificial intelligence, including composite AI. With extensive experience in delivering customized AI solutions across various industries, LeewayHertz can help organizations leverage composite AI to solve complex business challenges and drive innovation. Here’s how LeewayHertz can support your composite AI journey:
- Comprehensive AI strategy and consultation: LeewayHertz offers strategic consulting services to help organizations understand the potential of composite AI and how it can be integrated into their business processes. By conducting in-depth assessments of your current capabilities and business objectives, LeewayHertz can develop a tailored AI roadmap that aligns with your specific needs.
- Custom AI solution development and integration: Our team of AI experts designs and develops custom AI solutions that integrate various AI techniques, such as machine learning, natural language processing, computer vision, and expert systems, to create a powerful, multi-faceted framework. We ensure seamless integration with your existing systems and platforms to enhance efficiency and maximize the return on investment.
- Scalable AI infrastructure: Implementing composite AI requires robust infrastructure capable of handling complex computations and large datasets. LeewayHertz provides scalable AI infrastructure solutions, including cloud-based environments, data storage, and processing capabilities, ensuring your AI models operate efficiently and can scale as your needs grow.
- End-to-end implementation support: LeewayHertz provides end-to-end support throughout the AI implementation process, from initial planning and development to deployment and monitoring. Our agile methodology ensures that AI solutions are delivered on time and within budget while maintaining high quality and reliability.
- Continuous learning and optimization: We believe that AI models should continuously evolve to adapt to changing data and environments. LeewayHertz provides continuous support to refine and optimize your AI models, ensuring they continue to deliver accurate and valuable insights over time.
- Ethical AI practices: LeewayHertz is committed to developing AI solutions that are ethical and transparent. We ensure that all AI models are designed and implemented following best practices for data privacy, fairness, and transparency, helping organizations mitigate risks and comply with regulatory requirements.
- Industry-specific expertise: With experience across various industries, including healthcare, finance, retail, manufacturing, insurance, legal, and real estate, LeewayHertz understands the unique challenges and opportunities within each sector. We tailor our AI solutions to address industry-specific needs, providing targeted benefits and enhancing business outcomes.
Leverage the expertise of LeewayHertz to implement AI solutions that integrate multiple techniques, driving innovation and growth.
Ethical considerations in composite AI: Navigating the complex landscape
As composite AI systems become increasingly sophisticated and integrated into various aspects of our lives, ethical considerations take on paramount importance. This section explores the unique ethical challenges posed by composite AI and outlines essential principles for responsible development and deployment.
- Transparency and explainability:
- Black box problem: Composite AI systems often involve intricate interactions between multiple AI techniques, making it difficult to understand how they arrive at specific decisions. This lack of transparency raises concerns about accountability and trust.
- Explainable AI (XAI): Developing methods to explain the reasoning behind composite AI outputs is crucial for building trust and ensuring fairness. XAI techniques can help users understand how decisions are made, increasing transparency and accountability.
- Bias and fairness:
- Amplified bias: Composite AI systems can inherit and even amplify biases present in the data they are trained on. This can lead to discriminatory outcomes, particularly in sensitive domains like healthcare, finance, and justice.
- Bias mitigation strategies: It is essential to implement measures to identify, mitigate, and prevent biases in both the training data and the AI algorithms themselves. Techniques like fairness-aware algorithms, data augmentation, and bias detection tools can be utilized.
- Privacy and data security:
- Data sensitivity: Composite AI systems often rely on large datasets containing sensitive personal information. Protecting individual privacy and ensuring data security is paramount.
- Privacy-preserving techniques: Employing techniques like differential privacy, federated learning, and homomorphic encryption can help protect user data while still enabling AI development.
- Accountability and responsibility:
- Who is responsible? Determining accountability for the actions of composite AI systems can be challenging, especially when multiple stakeholders are involved.
- Clear guidelines and regulations: Establishing clear guidelines and regulations for the development, deployment, and use of composite AI is essential for promoting responsible innovation and minimizing potential harm.
- Societal impact and job displacement:
- Potential disruptions: Composite AI advancements could lead to significant job displacement in certain sectors. It is vital to anticipate these disruptions and develop strategies for workforce adaptation and retraining.
- Ethical considerations: Ensuring that the benefits of composite AI are distributed fairly across society and that vulnerable populations are not disproportionately disadvantaged is essential.
- Human-AI collaboration:
- Augmenting, not replacing: Composite AI should be viewed as a tool to augment human capabilities, not to replace them entirely.
- Human oversight: Maintaining human oversight and control over composite AI systems is crucial for ensuring ethical decision-making and preventing unintended consequences.
- Continuous monitoring and evaluation:
- Regular audits: Regularly assess the ethical implications of composite AI systems and make necessary adjustments to address emerging challenges.
- Stakeholder engagement: Engage with diverse stakeholders, including experts, policymakers, and the public, to gather feedback and ensure ethical considerations are continuously integrated into the development and deployment of composite AI.
Ethical considerations should be interwoven into every stage of the composite AI lifecycle, from design to deployment and beyond. By embracing these principles, we can harness the transformative power of composite AI while ensuring it is used responsibly for the benefit of all.
Addressing the limitations or potential drawbacks of composite AI
While composite AI offers numerous advantages, it is important to acknowledge its potential limitations and drawbacks, as well as strategies to address them:
Complexity and integration challenges: Integrating multiple AI techniques into a cohesive composite AI system can be a complex and resource-intensive process. To address this, organizations can adopt modular and scalable architectures, leveraging standardized interfaces and enterprise-grade integration platforms. Establishing cross-functional teams with diverse expertise can also facilitate seamless integration.
Data requirements: Composite AI systems often require access to large, high-quality, and diverse datasets to train and optimize their various components. Strategies to address this include data engineering best practices, intelligent data collection, and curation, as well as the use of synthetic data generation and data augmentation techniques.
Scalability limitations: While composite AI systems are designed to be scalable, there may be practical limits to the number of AI techniques that can be effectively integrated. To overcome these limitations, organizations can adopt cloud-native architectures, leverage distributed computing resources, and implement advanced model management and orchestration capabilities.
Ethical risks: The integration of multiple AI techniques can amplify the risks associated with biased data, opaque decision-making, and unintended consequences. Addressing these ethical concerns requires the development of robust governance frameworks involving cross-functional teams, ethics boards, and continuous monitoring and auditing processes.
Cost and resource constraints: Developing and deploying composite AI can be resource-intensive. To optimize costs, organizations can explore cloud-based deployment models, leverage open-source tools and libraries, and prioritize use cases with the highest potential return on investment.
By acknowledging these limitations and proactively addressing them through strategic approaches, organizations can unlock the full potential of composite AI while mitigating risks and ensuring responsible deployment.
The future of composite AI: Beyond the horizon
Composite AI, the integration of multiple artificial intelligence techniques to solve complex problems, is already making significant strides across various industries. As we look to the future, the potential of composite AI promises to expand even further, driving innovation and transforming how we approach complex challenges. Here’s a look at what the future might hold for composite AI:
1. Advanced integration of AI techniques
- Seamless integration: Future advancements in composite AI will likely focus on the seamless integration of diverse AI techniques. As technology evolves, combining machine learning, natural language processing (NLP), computer vision, and other methodologies will become more refined, allowing for more sophisticated and cohesive AI systems.
- Unified frameworks: The development of unified frameworks and platforms will facilitate the integration of various AI models. These frameworks will enable easier coordination between different AI techniques, improving efficiency and effectiveness in solving complex problems.
2. Enhanced autonomy and adaptability
- Self-optimizing systems: Future composite AI systems will feature enhanced autonomy, with models capable of self-optimization. These systems will continuously adapt and improve based on real-time data, leading to more accurate and responsive AI solutions.
- Adaptive learning: Advanced adaptive learning capabilities will allow composite AI to adjust to new information and changing environments more effectively. This will enable AI systems to remain relevant and effective in dynamic and evolving contexts.
3. Greater personalization and customization
- Tailored solutions: The future of composite AI will see a shift towards highly personalized and customized solutions. AI systems will be able to provide more tailored recommendations and insights, catering to individual needs and preferences across various applications, from healthcare to finance.
- User-centric design: Enhanced focus on user-centric design will ensure that composite AI solutions are more intuitive and aligned with user requirements. This will improve user experience and satisfaction, making AI tools more accessible and effective.
4. Integration with emerging technologies
- Quantum computing: The integration of composite AI with quantum computing could transform its capabilities. Quantum computing’s ability to handle complex computations at unprecedented speeds will enhance the performance and scalability of composite AI systems.
- Edge computing: Advances in edge computing will enable composite AI to operate more efficiently in real-time, processing data closer to its source. This will reduce latency and improve the responsiveness of AI systems, especially in applications requiring immediate feedback.
5. Ethical and responsible AI development
- Ethical frameworks: The development of robust ethical frameworks will become increasingly important. Future composite AI initiatives will prioritize ethical considerations, ensuring fairness, transparency, and accountability in AI systems.
- Regulatory compliance: As composite AI technology advances, regulatory compliance will evolve to address new challenges and standards. Proactive engagement with regulators and adherence to emerging guidelines will be crucial for responsible AI development.
6. Collaborative AI ecosystems
- AI partnerships: The future will likely see an increase in collaborative AI ecosystems, where organizations and researchers work together to develop and deploy composite AI solutions. These partnerships will foster innovation and accelerate the adoption of advanced technologies.
- Cross-industry collaboration: Cross-industry collaboration will become more prevalent, as composite AI solutions are applied to a wide range of sectors. Sharing knowledge and resources across industries will drive the development of more comprehensive and versatile AI systems.
7. Long-term impact and sustainability
- Sustainable AI practices: Future developments in composite AI will emphasize sustainability, focusing on minimizing environmental impact and promoting responsible resource use. Sustainable AI practices will ensure that technological advancements benefit society while preserving the environment.
- Societal benefits: The long-term impact of composite AI will extend beyond technological advancements, contributing to societal well-being and progress. By addressing complex challenges and driving innovation, composite AI will play a key role in shaping a better future for individuals and communities.
The future of composite AI is filled with exciting possibilities and transformative potential. As technology continues to evolve, composite AI will become more integrated, adaptive, and personalized, driving advancements across various industries. Embracing ethical practices, fostering collaboration, and focusing on sustainability will be essential to realizing the full potential of composite AI and ensuring its positive impact on society.
Endnote
Composite AI represents a significant leap forward in the field of artificial intelligence, offering a versatile and powerful approach to solving complex business challenges. By integrating multiple AI technologies, such as machine learning, deep learning, natural language processing, and computer vision, composite AI provides a more comprehensive and adaptable solution compared to traditional AI methods. This multifaceted approach enables organizations to address intricate problems, make human-like decisions, and gain valuable insights even from smaller datasets.
In an era where data is abundant yet often fragmented, composite AI’s ability to synthesize and analyze diverse information sources is crucial. It not only enhances decision-making capabilities but also reduces the dependency on large data science teams, allowing businesses to optimize their resources effectively. As AI continues to reshape industries, the adoption of composite AI will likely become a key differentiator for organizations seeking to remain competitive and innovative.
The growing emphasis on AI-driven strategies underscores the importance of deploying a flexible, powerful, and unified AI framework. Composite AI is well-positioned to lead the next phase of AI innovation, empowering businesses to navigate complex challenges with greater efficiency, precision, and insight. As organizations continue to embrace AI, composite AI will play a pivotal role in driving more effective, intelligent, and informed decision-making across various sectors.
Connect with LeewayHertz AI experts today to explore how composite AI can transform your business. Let us help you leverage the power of advanced AI technologies to solve your most complex challenges.
Start a conversation by filling the form
All information will be kept confidential.
Insights
AI in supplier management: The new frontier in procurement innovation
The role of AI in supplier management is multifaceted, offering solutions that enhance operational processes, mitigate risks, and foster strategic decision-making.
AI in hospitality: Use cases, applications, solution and implementation
AI has been making significant advancements in the hospitality industry, reforming various aspects of guest experiences, operational efficiency, and overall management.
AI for claims processing: Use cases, benefits and development
AI plays a significant role in resolving challenges in claims processing by introducing automation, data analysis, and advanced decision-making capabilities.