Customer churn prediction using machine learning: A comprehensive overview
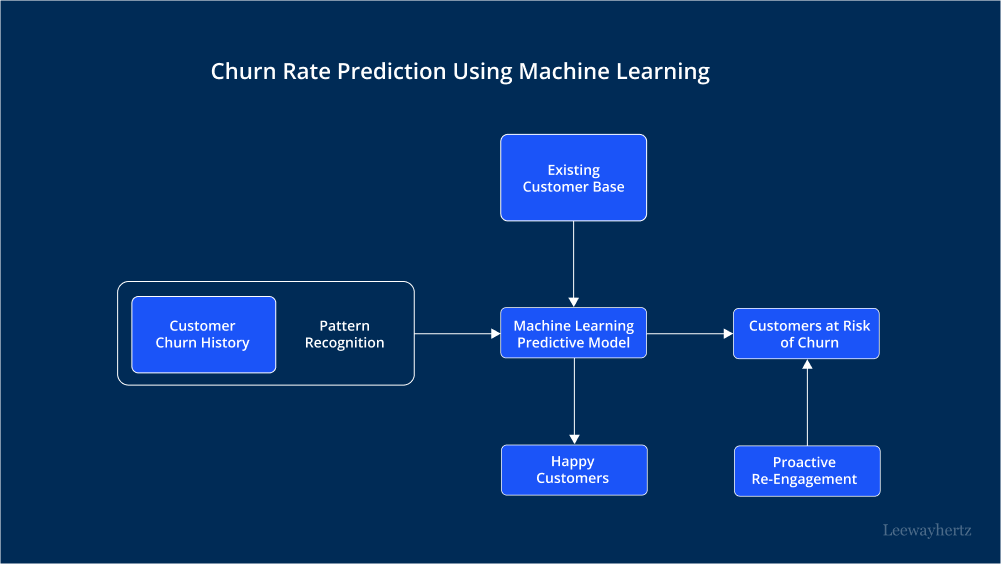
But no two companies are alike. An acceptable churn rate for one may be catastrophic for another. Even identifying when churn has occurred can be challenging: It may be sudden, deliberate, gradual, implicit, and unconscious. For example, a SaaS subscriber may let a service expire for unknown reasons and then rejoin a month later. Does that count as churn? If so, how is it quantified?
Every company is bound to have some level of churn. No matter how great your company’s products or services are, people and markets are always changing. The key is discovering the sweet spot where you are not forced into an economically unsustainable model of finding new customers to replace old ones. This challenge isn’t new. But today’s digital landscape allows us to take a more granular look at customer behavior—one that calibrates the retention versus acquisition question to individual behaviors.
Although it’s deceptively simple to calculate turnover with a simple equation—divide customers lost by the total number of customers in a given period—learning how, when, and why churn is occurring is more nuanced. You need a comprehensive view of the entire customer experience. One way to navigate that complexity is with data and Artificial Intelligence (AI).
This article delves into the realm of customer churn analysis. It explores the significance of understanding customer churn, the challenges encountered during the analysis process, and the pivotal role of AI, ML, and deep learning, emphasizing the importance of a comprehensive churn prediction model across various industries.
- Understanding customer churn
- Customer churn prediction across industries using machine learning
- Designing churn prediction workflow
- How LeewayHertz’s generative AI platform optimize customer churn analysis workflows?
- The significance of AI, ML and deep learning in churn prediction
- Advanced machine learning models for churn prediction
Understanding customer churn
Customer churn is when customers cease their relationship with a company or brand by discontinuing their subscription, canceling a service, or not making any further purchases. It is a critical metric for businesses as it directly impacts their revenue and growth. Understanding customer churn involves analyzing the reasons behind customer attrition and identifying patterns or trends that lead to customer disengagement. By gaining insights into why customers churn, businesses can take proactive measures to retain valuable customers and improve overall customer satisfaction.
It is a significant concern for businesses in various industries, including telecommunications, Software-as-a-service (SaaS), e-commerce, and more. Understanding and managing customer churn is essential for sustainable growth and maintaining a healthy customer base.
Types of customer churn: Customer churn can be classified into two main types:
Customer churn is a common problem across industries
- Voluntary churn: This occurs when customers willingly decide to terminate their relationship with a company. It might be due to factors like dissatisfaction with the product or service, competitive offers from other companies, changing needs, or financial constraints.
- Involuntary churn: Involuntary churn takes place when customers leave the company due to reasons beyond their control. For instance, this might occur when a customer passes away, changes their location to an area where the service is unavailable or faces a technical issue that remains unresolved.
Customer churn is a common problem across industries
Customer churn is not confined to a specific industry; it is a common challenge faced by businesses across various sectors, including telecommunications, e-commerce, banking, SaaS, and more. Regardless of the industry, organizations encounter customer churn for various reasons such as fierce competition, changing customer preferences, inadequate customer support, or subpar product or service offerings. Recognizing that churn is a universal concern highlights the importance of implementing effective churn prediction strategies to address this problem.
Customer churn is a common problem across industries due to several interconnected factors and challenges businesses face to retain customers. Here are some key reasons why customer churn is prevalent in various sectors:
Increased competition: Most industries are highly competitive, with numerous companies vying for the attention and loyalty of customers. When customers have multiple options, they are more likely to switch to a competitor offering better value or incentives.
Launch your project with LeewayHertz!
Enhance your business’s performance with AI-driven customer churn prediction! Reach out to our team of AI experts to create a customized churn prediction model for your company.
Global connectivity: The advent of the internet and global connectivity has made it easier for customers to research, compare, and switch between products and services. It has empowered customers with more information, making them less hesitant to try alternatives.
Quality of products and services: If a company’s products or services fail to meet customers’ needs or standards, they are likely to explore other options. Ensuring consistent quality is essential for customer satisfaction and retention.
Lack of personalization: Customers appreciate personalized experiences that cater to their specific preferences. When companies fail to personalize interactions and offerings, customers may feel undervalued and seek more attentive providers.
Customer service issues: Poor customer service is a significant driver of churn. When customers encounter unresolved problems or feel that their concerns are not adequately addressed, they may choose to switch to a company that provides better support.
Pricing and value perception: Pricing plays a crucial role in customer retention. If customers believe they are not receiving sufficient value for the price they pay, they may seek alternatives that offer a better cost-to-value ratio.
Life changes and circumstances: Customers’ needs and circumstances can change over time. Relocation, financial difficulties, or shifts in personal preferences can prompt churn even if the company’s offerings remain satisfactory.
Subscription-based business models: Industries that operate on subscription models, such as SaaS companies or streaming services, are particularly susceptible to customer churn. Customers can easily cancel their subscriptions, leading to recurring challenges in maintaining a steady customer base.
Lack of data-driven insights: Some companies struggle to collect and analyze customer data effectively. Without insights into customer behavior and preferences, they may miss opportunities to address churn indicators proactively.
Customer acquisition focus: Many companies prioritize customer acquisition over customer retention. While acquiring new customers is essential, neglecting existing customers can lead to higher churn rates and lower long-term profitability.
Inadequate churn prevention strategies: Some businesses may lack effective churn prevention strategies or resources to implement them. Failing to focus on retention and loyalty initiatives can contribute to higher churn rates.
Addressing customer churn requires a comprehensive and proactive approach that involves understanding customer needs, delivering exceptional experiences, leveraging data analytics, and continuously improving products and services. Companies can mitigate churn by taking customer retention seriously and building long-term relationships with their clientele.
Importance of customer churn prediction
Customer churn prediction plays a pivotal role in helping businesses take proactive measures to retain customers and mitigate the negative impact of churn. By predicting which customers are likely to churn, companies can focus their efforts on implementing targeted retention strategies for those at risk, thereby increasing the likelihood of customer loyalty. Churn prediction empowers businesses to allocate resources efficiently, improve customer service, enhance product offerings, and strengthen overall customer experience. Ultimately, accurate churn prediction translates to higher customer retention rates, improved revenue, and sustainable growth.
Customer churn prediction is a strategic tool that empowers companies to proactively address customer attrition and implement targeted retention efforts. Let’s delve into the detailed significance of customer churn prediction:
Cost savings: Customer acquisition costs are generally higher than retaining existing customers. Predicting and preventing customer churn can lead to substantial cost savings. By focusing resources on retaining at-risk customers, businesses can avoid spending excessive amounts on acquiring new customers to replace those lost due to churn.
Improved customer retention strategies: Churn prediction provides valuable insights into customer behavior and preferences. Armed with this knowledge, companies can fine-tune their customer retention strategies. They can design personalized interventions, loyalty programs, and customer support initiatives to enhance the overall customer experience and build stronger relationships.
Enhanced customer satisfaction: Predicting and addressing customer churn helps resolve issues and concerns before they escalate. By being proactive, businesses can demonstrate their commitment to customer satisfaction, leading to higher levels of trust and loyalty among their clientele.
Better resource allocation: Businesses can strategically prioritize their efforts and allocate resources more effectively by leveraging customer churn prediction. Instead of employing a one-size-fits-all approach, companies can focus on retaining customers who are most likely to churn, maximizing the impact of their retention initiatives.
Competitive advantage: Companies that can accurately predict and reduce churn gain a significant advantage in competitive markets. By nurturing a loyal customer base, businesses can differentiate themselves from competitors and position themselves as industry leaders.
Increased revenue and profits: Retaining customers through churn prediction directly impacts a company’s bottom line. Loyal customers tend to spend more, purchase additional products or services, and refer others to the business. Thus, reducing churn leads to increased revenue and long-term profitability.
Real-time intervention: AI-powered churn prediction systems can work in real-time, constantly monitoring customer behavior. When a customer displays early signs of potential churn, businesses can immediately intervene to resolve issues, address concerns, or offer personalized incentives. Real-time intervention increases the chances of retaining customers on the verge of churning.
Data-driven decision-making: Customer churn prediction relies on data analysis and AI algorithms. It promotes data-driven decision-making, allowing businesses to base their strategies on empirical evidence rather than assumptions or guesswork.
Continuous improvement: Churn prediction models can be continuously refined and updated based on new data. As the churn prediction model becomes more accurate over time, businesses can stay ahead of evolving customer behavior and churn trends, adapting their strategies accordingly.
Customer-centric approach: Customer churn prediction fosters a customer-centric approach to business. By focusing on customer needs and preferences, companies can better align their offerings with customer expectations, leading to increased customer satisfaction and loyalty.
In today’s competitive business landscape, customer churn prediction is not just a useful tool; it’s a strategic imperative. The ability to anticipate and prevent customer churn through AI and data analytics empowers businesses to optimize their resources, improve customer retention strategies, boost revenue, and gain a competitive advantage. By staying ahead of churn trends and proactively retaining customers, companies can foster long-term customer loyalty and achieve sustainable growth and success.
Customer churn prediction across industries using machine learning
AI-powered churn prediction can be applied across various industries to help businesses identify and retain customers. Here are some AI use cases for churn prediction:
Telecommunications
Problem: Telecom companies can use churn prediction to identify customers at risk of leaving their services.
AI solution: Implementing machine learning models to analyze call patterns, data usage, and customer service interactions to predict potential churn. This empowers telecom companies to proactively retain customers by deploying targeted offers and personalized incentives.
Subscription services (e.g., streaming platforms)
Problem: Subscription-based businesses need to minimize subscriber churn to maintain revenue.
AI solution: Applying machine learning algorithms to analyze user engagement, content preferences, and usage patterns to predict when a subscriber might cancel their subscription. This enables targeted content recommendations and retention strategies.
E-commerce
Problem: E-commerce platforms face the challenge of high customer attrition rates and a need to enhance customer lifetime value.
AI solution: Leveraging machine learning models to analyze customer purchasing behavior, browsing history, and interactions with the platform. Predictions can help e-commerce businesses offer personalized promotions, discounts, or product recommendations to retain customers.
Banking and financial services
Problem: Banks want to prevent customer attrition and retain valuable account holders.
AI solution: Using machine learning to analyze transaction history, account activity, and customer service interactions to predict potential churn. Banks can then offer personalized financial products or services to retain customers.
SaaS (Software as a Service) providers
Problem: SaaS providers face the challenge of minimizing customer churn and enhancing user engagement to ensure long-term subscription commitment and satisfaction.
AI solution: Implementing machine learning models to analyze user interactions with the software, feature usage, and support ticket data. This helps in predicting when a customer might discontinue the service, allowing proactive engagement and feature improvements.
Gaming industry
Problem: Online gaming platforms want to retain players and prevent them from leaving for competing platforms.
AI solution: Utilizing machine learning to analyze player behavior, in-game activities, and social interactions. Churn prediction can be used to offer targeted promotions, new content, or personalized gaming experiences to keep players engaged.
Insurance
Problem: Insurance companies want to retain policyholders and prevent them from switching to competitors.
AI solution: Applying machine learning models to analyze claims history, customer interactions, and policy changes to predict potential churn. Insurers can then offer personalized policy adjustments or discounts to retain customers.
Retail
Problem: Retailers aim to reduce customer churn and enhance loyalty.
AI solution: AI helps retailers reduce customer churn by utilizing machine learning to analyze purchase history, customer feedback, and engagement with loyalty programs. Predicting potential churn enables retailers to implement personalized marketing strategies and loyalty rewards, ultimately enhancing customer retention.
Healthcare
Problem: Healthcare providers, including insurance companies and medical services, want to retain patients and policyholders.
AI solution: In healthcare, AI addresses the challenge of retaining patients and policyholders by implementing machine learning models. These models analyze patient history, appointment attendance, claims data, and interactions with healthcare services. Churn prediction allows healthcare providers to identify patients at risk of leaving and implement personalized healthcare plans, preventive measures, or loyalty programs to enhance patient retention.
Hospitality
Problem: Hotels and travel agencies want to retain customers and prevent cancellations or bookings with competitors.
AI solution: Using machine learning to analyze booking history, stay preferences, and customer feedback to predict potential churn. Hospitality businesses can then offer personalized discounts, upgrades, or loyalty programs to enhance customer loyalty.
Automotive
Problem: Car dealerships and service centers aim to retain customers for vehicle purchases and maintenance services.
AI solution: AI addresses the challenge of retaining customers for vehicle purchases and maintenance services in the automotive sector by analyzing customer service records, vehicle maintenance history, and purchase patterns. Predicting potential churn allows automotive businesses to proactively offer personalized service packages, discounts, and loyalty programs, fostering customer retention for future purchases and maintenance needs.
Logistics
Problem: Logistics companies want to retain clients and prevent them from switching to other providers.
AI solution: By analyzing shipping and delivery performance, client interactions, and contract renewal patterns, AI predicts potential churn in logistics clients. This enables logistics companies to provide personalized solutions, implement performance improvement plans, and introduce loyalty programs to enhance client retention.
Manufacturing
Problem: Manufacturers aim to retain clients and prevent them from seeking production services from other companies.
AI solution: AI analyzes production performance, order history, and client feedback to predict potential churn in manufacturing clients. Manufacturers can leverage AI insights to offer personalized production plans, quality improvement initiatives, and customized pricing structures, thereby retaining clients.
Implementing AI-powered churn prediction in these use cases allows businesses to proactively address customer concerns, personalize interactions, and implement targeted retention strategies, ultimately improving customer satisfaction and reducing revenue loss.
Designing churn prediction workflow
Churn prediction is crucial for businesses seeking to reduce customer attrition and improve customer retention strategies. To build an effective customer churn prediction model, it is essential to follow a well-structured workflow. Here are the key stages of the churn prediction workflow in detail:
1. Define the problem
Understanding the problem and defining the final goal are crucial initial steps in designing a churn prediction solution. Before delving into the analysis, it is essential to determine the insights needed and the questions to be answered through machine learning.
Classification: Classification is a type of ML problem where the goal is to determine which class or category a data point (customer, in our case) belongs to. Data scientists train the algorithm by using historical data with predefined target variables or labels (such as churner/non-churner). Classification helps businesses answer important questions, including:
- Will this customer churn or not?
- Will a customer renew their subscription?
- Will a user opt for a lower-priced plan?
- Are there any indications of unusual customer behavior?
Anomaly detection, a subset of classification, involves identifying outliers or data points that significantly deviate from the rest of the data. This helps in detecting unusual customer behavior, which might indicate potential churn risk.
Regression: Customer churn prediction can also be formulated as a regression task. Regression analysis is a statistical method utilized to estimate the association between a target variable and other influencing data values, all expressed in continuous form. In simple terms, regression predicts numerical values, while classification predicts categories.
With regression, businesses can forecast the specific period in which a customer is likely to churn or receive a probability estimate of churn for each customer. This allows companies to make more precise predictions about when a customer might churn, aiding in the design of targeted retention strategies.
2. Data collection and preprocessing
Identifying relevant data sources
The first step in designing a churn prediction workflow is to identify the data sources that will provide valuable insights into customer behavior. Relevant data sources may include customer transaction records, usage patterns, customer demographics, customer service interactions, and feedback data. Data from CRM systems, billing systems, marketing campaigns, and social media can also be valuable for churn prediction.
Cleaning and enhancing the churn dataset
Data cleaning is crucial to ensure the quality and reliability of the dataset. This involves handling missing values, correcting errors, and removing irrelevant or duplicate records. After cleaning, the dataset may need enhancement through data enrichment techniques. This can involve merging additional data from external sources to enrich customer profiles and gain more valuable features for the customer churn prediction model.
Feature engineering for churn prediction
Feature engineering is a vital step in churn prediction as it involves selecting and creating informative features that help the model understand customer churn patterns. Some essential churn-related features include customer tenure, frequency of interactions, average transaction amount, customer complaints, and customer engagement metrics.
- Temporal features: Temporal features play a crucial role in churn prediction, as customer behavior often changes over time. For example, features such as “time since last purchase” or “time since the last customer service interaction” can be powerful indicators of churn likelihood.
- Behavioral features: Behavioral features capture how customers engage with the product or service. Examples of behavioral features include the number of logins, click-through rates, or the usage of specific product features.
- Customer interaction features: These features capture the customer’s interaction with the company, such as call duration, frequency of customer service calls, and responses to marketing campaigns.
Selecting an observation window for churn prediction
Predictive modeling involves learning from observations made during a specific period, known as the observation window or customer event history, and making predictions about a future period called the performance window. Understanding when users typically churn is crucial in defining the observation and performance window lengths. Striking a balance between these windows is challenging, as a short observation window may lack sufficient data for accurate predictions, while a long performance window allows more time for re-engagement efforts. Iterative experimentation is key to finding the optimal windows, considering factors like churn rate, data availability, and re-engagement strategies. Continuous monitoring and adjustment lead to a refined churn prediction model that maximizes the effectiveness of retention efforts.
Methods for defining relevant and useful features
In churn prediction, it’s essential to identify the most relevant and useful features that significantly contribute to the model’s accuracy. Some powerful methods for achieving this:
- Permutation importance: Permutation importance is a potent technique that empowers data scientists to assess the impact of specific features on churn predictions. The method involves altering the order of data points in a particular feature column and measuring the resulting decrease in model accuracy. The greater the reduction in accuracy, the more important the feature is for making precise predictions. By leveraging permutation importance, data scientists can pinpoint the attributes that significantly contribute to the model’s ability to identify potential churners.
- ELI5 Python package: The ELI5 Python package is a valuable tool that provides visualizations and debugging capabilities for interpreting machine learning classifiers. It enables data scientists to gain insights into how models arrive at their predictions based on specific features. ELI5’s intuitive visualizations offer a deeper understanding of the relationships between features and outcomes, aiding in the selection of critical attributes for churn prediction models.
- SHAP (SHapley Additive exPlanations): The SHAP framework is a powerful tool for interpreting the decisions of “any machine learning model.” An importance value is assigned by SHAP to each feature for a particular prediction, quantifying the contribution of each attribute to the model’s output. This method helps identify the most significant features and sheds light on the interplay between multiple attributes, providing a more comprehensive understanding of churn drivers.
Addressing imbalanced data in churn prediction
Churn prediction datasets are often imbalanced, with the number of churn instances being significantly lower than non-churn instances. This imbalance can lead to biased models that perform poorly in predicting churn. Techniques such as resampling (oversampling or undersampling) and synthetic data generation (SMOTE) can effectively address data imbalance.
- Resampling techniques: Resampling techniques can be applied to address data imbalance, such as oversampling the minority class (churn) or undersampling the majority class (non-churn). These techniques effectively balance the class distribution and help the model learn from the churn and non-churn instances.
- Synthetic data generation: Another approach to deal with imbalanced data is to use synthetic data generation methods like SMOTE (Synthetic Minority Over-sampling Technique). SMOTE generates synthetic samples for the minority class, effectively increasing its representation in the dataset.
Data normalization for churn prediction
Normalization is crucial for algorithms that rely on distance-based calculations, such as Support Vector Machines or Neural Networks. Normalizing the features to the same range prevents certain features from dominating the model solely due to their magnitude. Techniques like Min-Max scaling and Z-score standardization are commonly used for data normalization.
- Min-Max scaling: One common normalization technique is Min-Max scaling, which rescales the features to a specific range (e.g., [0, 1]) based on their minimum and maximum values.
- Z-score standardization: Another approach is Z-score standardization, which transforms features to have a mean of 0 and a standard deviation of 1. This method is useful when the data has a Gaussian distribution.
3. Exploratory Data Analysis (EDA)
EDA is a critical step in the churn prediction process that involves exploring and visualizing the data to gain valuable insights, identify patterns, and understand the relationships between variables. EDA provides a foundation for building an effective churn prediction model and guiding feature selection. Let’s dive into the key components of EDA in the context of customer churn prediction:
Visualizing churn patterns and trends
Visualizations are powerful tools for understanding churn patterns and trends over time. Line plots and bar charts can be used to visualize the churn rate over different time periods, such as weeks, months, or quarters. This helps identify any seasonality or periodic patterns in customer churn.
Heatmaps and clustering can also be employed to visualize churn patterns across different customer segments. Heatmaps can show churn rates based on customer demographics or behaviors, while clustering algorithms can group customers into segments with similar churn behavior. Such visualizations provide valuable insights into which customer groups are more likely to churn and when churn rates are highest.
Analyzing feature correlations in churn data
Feature correlation analysis helps identify relationships between variables in the churn dataset. Understanding these correlations is vital for feature selection, as highly correlated features may introduce redundancy and lead to model overfitting.
Correlation matrices and scatter plots are commonly used to analyze feature correlations. A correlation matrix shows the pairwise correlation coefficients between features, while scatter plots visually depict the relationship between two features. Identifying correlations between churn-related features can help focus on the most influential variables for churn prediction.
Importance of feature selection for churn prediction
Feature selection is crucial in building an accurate and interpretable churn prediction model. This process entails selecting the most relevant and informative features to train the model and eliminating irrelevant or redundant ones. Ensemble methods such as gradient boosting machines or random forests allow us to acquire feature importance scores. These scores rank features based on their contribution to the model’s predictive performance. Features with high importance scores are likely to be strong predictors of churn and should be prioritized in the final model. Feature selection improves the model’s accuracy and reduces its complexity, making it more interpretable and easier to maintain.
Uncovering customer segments for churn insights
Customer segmentation is a potent technique for understanding churn behavior in different customer groups. By grouping customers with similar characteristics or behaviors, businesses can tailor their retention strategies to meet the specific needs of each segment. Clustering algorithms like K-Means or Hierarchical Clustering can be applied to uncover customer segments based on churn-related features. These algorithms partition the data into distinct clusters, with each cluster representing a different customer segment. Analyzing churn patterns within each segment can reveal valuable insights, such as the most significant factors driving churn within specific customer groups. Customer segmentation can help businesses identify high-value customers at risk of churning and design personalized retention campaigns to target those segments effectively.
4. Model selection and training
Model building and selection are critical stages in the churn prediction process. These steps involve choosing appropriate machine learning algorithms, training models, evaluating their performance, and selecting the best model for predicting customer churn accurately. Let’s explore the key components of model building and selection in detail:
Choice of machine learning algorithms
Selecting suitable machine learning algorithms is the first step in building a churn prediction model. Commonly used algorithms include logistic regression, decision trees and random forests, support vector machines, gradient boosting machines, and neural networks. The selection of the algorithm depends on factors such as dataset size, data complexity, interpretability requirements, and available computational resources.
Training and evaluating models
After selecting the algorithms, the next step is to train the models on the churn dataset. The dataset is divided into training and testing sets to assess model performance.
Training the models involves feeding the algorithms with input features and corresponding churn labels. The models learn from the data to make predictions on unseen instances. During training, model hyperparameters may be adjusted to improve performance. The trained models are then evaluated using metrics such as accuracy, precision, recall, the area under the Receiver Operating Characteristic (ROC) curve and F1 score.
Model comparison and selection
Once multiple models have been trained and evaluated, it is essential to compare their performance to select the best-performing model for churn prediction.
The selection of the evaluation metric depends on the specific business needs. For example, if minimizing false negatives (predicting a non-churn customer as churn) is crucial, recall (true positive rate) becomes a significant metric. On the other hand, if maintaining high precision (positive predictive value) is vital, precision becomes the primary metric.
A validation set or cross-validation techniques are employed to simulate the model’s performance on unseen data to facilitate model selection. Cross-validation helps avoid overfitting and provides a more robust estimation of the model’s generalization performance.
Model ensemble for improved accuracy
Ensemble techniques combine predictions from multiple base models to generate a significantly improved and reliable final prediction. Ensemble methods can help mitigate the impact of individual model weaknesses and improve overall model accuracy.
Some popular ensemble techniques for churn prediction include:
- Bagging: Bagging involves building multiple base models using bootstrapped subsets of the training data and then combining their predictions through averaging or voting. It helps to reduce overfitting and increase the overall accuracy and stability of the churn prediction model.
- Boosting: Boosting is another popular ensemble technique where multiple base models are trained sequentially, with each model giving more weight to the misclassified instances from the previous models. The final prediction is the weighted combination of the individual model predictions, and this approach improves the model’s predictive power and generalization.
- Stacking: Stacking combines the predictions of multiple base models by using another model (meta-model) to learn how to merge the base models’ outputs best. The base models serve as input features to the meta-model, and this process enhances the overall predictive performance and adaptability of the churn prediction model.
- AdaBoost: AdaBoost is a specific variant of boosting that assigns higher weights to misclassified instances, and the subsequent base models focus on these samples to improve their prediction accuracy. It is particularly useful for dealing with class imbalance in churn prediction datasets.
Ensemble techniques can significantly improve model performance, particularly when the base models complement each other in capturing different aspects of churn patterns.
5. Model optimization and hyperparameter tuning
Model optimization and hyperparameter tuning are crucial steps in fine-tuning machine learning models for accurate and robust churn prediction. These steps aim to improve model performance, avoid overfitting, and enhance the model’s generalization to unseen data. Let’s delve into the key components of model optimization and hyperparameter tuning:
Cross-validation techniques
Cross-validation is a critical technique used to assess a model’s generalization performance and reduce bias in model evaluation. Instead of evaluating the model on a single train-test split, cross-validation involves dividing the data into multiple subsets (folds). The training process involves utilizing a subset of the data for model training, and subsequently, the model’s performance is assessed on the remaining fold of the data. This process is repeated several times, with each fold serving as the test set once.
Commonly used cross-validation techniques include:
- k-Fold cross-validation: This method divides the data into k subsets, and each time, the model is trained on k-1 subsets while being tested on the remaining subset. This process is iterated k times, and the final performance metrics are obtained by averaging the results.
- Stratified k-Fold cross-validation: Ensures that each fold maintains the same class distribution as the original dataset. This is especially important when dealing with imbalanced churn datasets.
Cross-validation offers a more dependable estimate of the model’s performance on unseen data and aids in identifying concerns like overfitting and underfitting.
Grid search and random search
Hyperparameter tuning involves the task of selecting the best hyperparameters for a machine-learning model. These hyperparameters are set before the model is trained and include variables such as the number of hidden layers in a neural network, the learning rate, and the number of estimators in an ensemble model. Grid search and random search are commonly used techniques for hyperparameter tuning:
- Grid search: Performs an exhaustive search through a predefined set of hyperparameter values to find the combination that yields the optimal model performance.
- Random search: Randomly samples hyperparameter values from predefined distributions, making it computationally less expensive, especially when dealing with a large number of hyperparameters and possible values.
6. Churn prediction model deployment and integration
Deployment and integration are crucial stages in the customer churn prediction process, where the developed churn prediction model is put into practical use and integrated into existing business processes. Let’s explore each component in detail:
Model deployment options
Model deployment involves making the churn prediction model accessible and usable by the business stakeholders. There are various deployment options to consider:
- Application Programming Interface (API) deployment: Deploying the churn prediction model as an API allows other applications and systems to make real-time predictions by sending input data to the API endpoint.
- Batch deployment: In batch deployment, the churn prediction model is applied to a large batch of data at once, making predictions for multiple customer records simultaneously. This is suitable for scenarios where real-time prediction is not required, such as periodic churn analysis.
- Model export: Exporting the trained churn prediction model to a file format (e.g., pickle, PMML) allows for easy import and use in different environments.
Real-time vs. batch prediction
The choice between real-time and batch prediction depends on the business requirements and the frequency at which churn predictions are needed:
Real-time prediction: The churn prediction model provides immediate predictions in response to incoming customer data. This is particularly helpful for applications that require instant decision-making, such as personalized offers or retention interventions triggered by customer behavior.
Batch prediction: Batch prediction, on the other hand, involves making churn predictions in bulk for a group of customers. This approach is suitable for scenarios where predictions, such as monthly or quarterly churn reports, can be made less frequently.
Integrating models into business processes
It must be seamlessly integrated into the existing business processes to derive maximum value from the churn prediction model. Integration involves incorporating churn predictions into various customer-facing and internal systems, such as:
- Customer Relationship Management (CRM) systems: Integrating churn predictions into CRM systems enables targeted retention actions during interactions.
- Marketing automation platforms: Integrating churn predictions with marketing automation platforms allows personalized campaigns for retaining high-risk customers.
- Customer segmentation: Integrating churn predictions into customer segmentation processes helps tailor retention strategies.
- Decision support systems: Churn predictions can be integrated into decision support systems for data-driven decisions.
Handling privacy and ethical concerns
Customer churn prediction involves working with sensitive customer data raising privacy and ethical concerns. Businesses must take measures to ensure the responsible use of customer data:
- Data privacy compliance: Ensuring compliance with relevant data protection regulations (e.g., GDPR, CCPA) and obtaining necessary permissions for data use.
- Data anonymization: Removing or encrypting personally identifiable information (PII) from the churn dataset to protect customer privacy.
- Transparency and explainability: Ensuring that the churn prediction model is transparent and explainable, allowing stakeholders to understand how predictions are made.
- Ethical use of predictions: Ensuring that churn predictions are used ethically, respecting customer rights and interests.
7. Monitoring and maintenance of the churn prediction model
Monitoring and maintenance are essential aspects of the customer churn prediction process to ensure the continued effectiveness and reliability of the churn prediction model. As customer behaviors and market dynamics change over time, continuous monitoring and updates are necessary to keep the model accurate and up-to-date. Let’s explore the key components of monitoring and maintenance in customer churn prediction:
Model performance monitoring
Regularly monitoring the churn prediction model’s performance is crucial to detect any potential issues and assess its effectiveness over time. Key performance metrics, such as precision, recall, accuracy, and F1 score, should be tracked periodically. Continuous model performance evaluation helps in identifying signs of model degradation or shifts in customer behavior that may impact predictive accuracy. A decline in performance may indicate the need for model retraining or updates to address changing patterns in customer churn.
Data quality and distribution
Data quality plays a crucial role in the performance of the churn prediction model. Changes in data sources, data collection processes, or the business environment can lead to shifts in data distribution. Therefore, it is essential to monitor data quality and ensure that the input data for the model remains accurate and consistent. Data drift, where the data distribution shifts over time, can negatively impact model performance. Regularly checking for data drift and adapting the model to changes in data distribution helps maintain predictive accuracy.
Model retraining and updates
As the churn prediction model is deployed and used in real-world scenarios, it may encounter new customer behaviors or patterns not present in the training data. This phenomenon is known as concept drift. When concept drift occurs, model retraining becomes necessary to ensure the model adapts to the evolving data. Regularly scheduled model retraining ensures that the model captures the latest churn patterns and provides up-to-date predictions. The frequency of retraining depends on the pace of change in the business environment and customer behavior.
A/B testing and model comparison
To continuously improve the churn prediction model, businesses can conduct A/B testing, where different model versions are tested simultaneously. This allows for a direct comparison between the existing model and potential enhancements. A/B testing helps validate the impact of model updates on performance, allowing businesses to make informed decisions about model improvements.
Error analysis and feedback
Analyzing prediction errors and gathering feedback from stakeholders, such as customer service representatives or marketing teams, provides valuable insights for model improvement. Identifying the types of customer churn instances the model struggles to predict correctly can guide efforts to improve model accuracy in specific scenarios.
Version control and documentation
Maintaining version control for the churn prediction model and its components helps track changes, ensuring reproducibility and consistency. Detailed documentation of the model architecture, hyperparameters, feature engineering techniques, and data preprocessing steps aids in understanding the model’s decision-making process and facilitates collaboration among team members.
By following this structured workflow, businesses can effectively build, deploy, and maintain churn prediction models, leading to better customer retention strategies and improved business outcomes.
If your business is grappling with the challenges of customer churn and seeking a technology partner, LeewayHertz is your reliable ally. Specializing in customer churn prediction using advanced AI models and solutions, LeewayHertz offers consulting and development services to elevate your strategies for retaining valuable customers. With a proven track record in deploying advanced AI techniques, LeewayHertz can kickstart or enhance your AI journey, providing tailored solutions to address the specific needs of your business.
How LeewayHertz’s generative AI platform optimize customer churn analysis workflows?
LeewayHertz’s generative AI platform, ZBrain, stands as a valuable asset for businesses aiming to improve customer churn analysis processes, promoting operational efficiency and sustained growth. ZBrain empowers organizations to craft tailored LLM-based applications, enabling refinement of workflows, improvement of customer interactions, and heightened accuracy in churn analysis operations. Leveraging advanced language models such as GPT-4, Vicuna, Llama 2, and GPT-NeoX, ZBrain adapts to various data formats, including text, images, and documents, ensuring versatile applications for informed decision-making and enhanced productivity while maintaining strict data privacy standards.
In the domain of customer churn analysis, prevalent challenges include inaccurate churn predictions, suboptimal utilization of customer data, and the need for swift decision-making to retain valuable customers. ZBrain adeptly addresses these challenges through its dedicated Flow feature, offering an intuitive interface for effortless business logic creation without coding requirements. The platform seamlessly integrates diverse large language models, prompt templates, and media models, providing a user-friendly experience through a drag-and-drop interface for the development and modification of sophisticated, intelligent applications.
By harnessing the capabilities of AI-driven automation and advanced data analysis, ZBrain’s flows play a transformative role in converting intricate customer data into actionable insights. This results in improved accuracy in churn predictions, reduced error rates, and elevated overall quality in customer churn analysis, offering comprehensive solutions to key challenges faced in this domain. Here is an example –
AI-driven customer churn analysis
ZBrain redefines customer churn analysis for banking and finance institutions through a streamlined and efficient process. Leveraging ZBrain Flow, the platform securely gathers and analyzes crucial information such as customer transaction history, account details, demographic data, interaction logs, and feedback surveys. This automated data collection ensures a comprehensive understanding of customer behavior and potential churn indicators, setting the stage for effective analysis. The LLM-based applications developed using ZBrain go beyond conventional methods, enhancing planning accuracy, optimizing resource allocation, and streamlining customer churn prediction. This transformative approach leads to heightened operational efficiency, reduced waste, and improved resource utilization for financial institutions. Explore this flow to delve into the agile and efficient customer churn analysis facilitated by ZBrain, allowing financial institutions to adapt swiftly to market demands and ensure optimal productivity. The streamlined process not only saves time and costs but also significantly improves customer retention by empowering institutions to make informed, data-driven decisions and provide personalized solutions.
The significance of AI, ML, and deep learning in churn prediction
AI, ML, and deep Learning play a pivotal role in redefining customer churn prediction from a conventional rule-based method to a data-driven and predictive strategy. AI and ML algorithms analyze large volumes of historical customer data, including demographic information, transaction history, behavior patterns, and interactions with the company.
These algorithms can build churn prediction models to forecast customer churn by identifying hidden patterns and correlations. Deep learning, a subset of ML, leverages neural networks to automatically extract complex features from data, further enhancing churn prediction accuracy. Let’s explore the specific roles of AI, ML, and deep learning in churn prediction:
Data processing and analysis: AI, ML, and deep Learning technologies excel at processing and analyzing large volumes of structured and unstructured data. In churn prediction, these technologies have the capability to integrate data from diverse sources, including customer interactions, purchase history, demographic information, and customer feedback. This comprehensive analysis provides valuable insights into customer behavior and churn patterns.
Feature extraction and selection: AI and ML algorithms can automatically identify relevant features or variables that contribute to customer churn. By carefully selecting the most impactful predictors, the churn prediction models can concentrate on the key factors influencing churn, thereby streamlining the churn prediction process.
Predictive modeling: Machine learning algorithms play a central role in building churn prediction models. Based on historical data, supervised learning techniques, such as logistic regression, decision trees, random forests, and support vector machines, are commonly employed to classify customers as potential churners or loyal customers.
Deep learning for complex patterns: Deep learning, a subset of ML, utilizes neural networks to process extensive datasets and reveal intricate patterns that may prove challenging to detect through conventional ML methods. Deep learning can identify intricate relationships and dependencies between customer attributes and churn indicators in churn prediction.
Real-time churn prediction: AI technologies enable real-time churn prediction, allowing businesses to monitor customer behavior continuously. AI-powered systems can identify early signs of potential churn, triggering immediate alerts and interventions to prevent customer attrition before it occurs.
Personalization and customer segmentation: ML-powered churn prediction models can segment customers based on various attributes and behaviors, creating targeted and personalized interventions to retain customers. Businesses can significantly enhance the chances of retaining their customers by tailoring offers, discounts, or recommendations according to individual customer preferences.
Model optimization and continuous improvement: AI and ML-based churn prediction models can be continuously optimized and refined over time as new data becomes available. Regular model updates ensure that the churn prediction algorithms remain accurate and effective, adapting to changing customer behavior and churn patterns.
Sentiment analysis and customer feedback: AI-powered sentiment analysis tools can automatically process customer feedback, including reviews, surveys, and social media posts. This analysis empowers businesses with valuable insights into customer sentiment, allowing them to identify and address potential issues proactively.
Explainable AI for business insights: Some AI techniques, like explainable AI, can provide transparent explanations for churn predictions. This helps businesses understand the factors influencing churn decisions, enabling them to make more informed and actionable decisions to improve customer retention.
Integration with CRM and marketing platforms: ML-powered churn prediction models can be integrated seamlessly with Customer relationship management systems and marketing platforms. This integration enables businesses to automate churn prevention strategies, making customer retention efforts more efficient and effective.
AI, ML, and deep learning play critical roles in churn prediction by enabling data-driven insights, building accurate customer churn prediction models, and facilitating real-time interventions to prevent customer attrition. These technologies have transformed churn prediction from a reactive process to a proactive and personalized approach, empowering businesses to retain valuable customers and achieve sustainable growth.
Launch your project with LeewayHertz!
Enhance your business’s performance with AI-driven customer churn prediction! Reach out to our team of AI experts to create a customized churn prediction model for your company.
Advanced machine learning algorithms for churn prediction
Customer churn is a critical concern for businesses across various industries. Companies are turning to advanced machine learning models to mitigate churn and retain valuable customers. We will explore several powerful machine learning algorithms used for churn prediction: logistic regression, decision trees and random forests, support vector machines, gradient boosting machines, XGBoost, LightGBM, CatBoost and neural networks.
Logistic regression
Logistic regression is a widely used and interpretable algorithm for binary classification problems like churn prediction. Despite its simplicity, it remains an essential tool in the machine learning toolbox. Logistic regression models the probability of a customer churning as a function of various predictor variables. The main advantage of logistic regression is its interpretability. The model can provide insights into the impact of each predictor variable on the likelihood of churn. Additionally, it requires relatively fewer computational resources and is less prone to overfitting when compared to more complex models. However, logistic regression may struggle to capture complex relationships in the data, and its predictive power might be limited compared to more advanced algorithms. As a result, it is often used as a baseline model for churn prediction before exploring more sophisticated approaches.
Decision trees and random forests
Decision trees are non-linear models that recursively split the data based on the most informative features to create a tree-like structure. In this representation, every internal node signifies a decision made using a specific feature, while each leaf node represents a class label, indicating either “churn” or “no churn.” Random forests, an ensemble method, take the idea of decision trees further by building multiple trees and aggregating their predictions to make a final prediction. The key advantage of random forests is their ability to handle non-linear relationships and interactions between features, making them more accurate than individual decision trees. Decision trees and random forests offer interpretability, as the decision-making process is evident from the tree structure. However, they might not perform well with high-dimensional data and can be sensitive to noisy features.
Support Vector Machines (SVMs)
Support vector machines are powerful supervised learning algorithms that can handle both non-linear and linear classification tasks. SVM aims to find a hyperplane that best separates churn and non-churn instances in a high-dimensional feature space. The strengths of SVM include its ability to handle complex decision boundaries and capture intricate patterns in the data. Through the use of the kernel trick, SVM can efficiently handle non-linear relationships. However, SVMs may become computationally expensive, particularly with large datasets, and interpreting them can be challenging due to the complexity of the separating hyperplane.
Gradient Boosting Machines (GBMs)
Gradient Boosting Machines are a popular ensemble technique that builds multiple weak learners (usually decision trees) sequentially, each focusing on the errors of its predecessor. GBM excels at capturing complex relationships and interactions in the data, making it highly effective for churn prediction. It can handle missing data well and requires little data preprocessing. However, GBM may overfit if not carefully tuned, which can be a time-consuming and computationally intensive process.
XGBoost
XGBoost (Extreme Gradient Boosting) is an enhanced version of gradient boosting machines that further improves predictive performance. XGBoost employs a more regularized model formalization to control overfitting and introduces a novel “gradient boosting” algorithm. Its advantages include scalability, efficiency, and flexibility. XGBoost has become popular in churn prediction tasks due to its outstanding performance and robustness.
LightGBM
LightGBM is a gradient-boosting framework that uses tree-based learning algorithms. It can be used for many machine-learning tasks, including classification and ranking. LightGBM is known for its faster training speed, higher efficiency, and greater accuracy. Additionally, it utilizes lower memory and is capable of handling large volumes of data. It also supports parallel and GPU learning, making it efficient for training large datasets. LightGBM is another powerful option for churn prediction.
CatBoost
CatBoost is another gradient-boosting framework that employs decision trees and handles both numerical and categorical features. CatBoost is suitable for various machine learning tasks, such as classification, regression, ranking, and more. CatBoost offers the advantage of enabling model training using both CPU and multiple GPUs. This dual capability expedites the training process and substantially enhances predictive performance. CatBoost is another valuable algorithm for churn prediction tasks.
Neural networks for churn prediction
Neural Networks, especially deep learning models, have shown remarkable success in various applications, including churn prediction. They can automatically learn complex features from raw data, making them highly adaptable to various churn-related information. Neural Networks can capture non-linear relationships, time dependencies, and intricate patterns that might be challenging for other models to discern. They can incorporate structured and unstructured data, such as customer demographics and textual feedback. Nonetheless, Neural Networks generally demand a larger volume of data and computational resources to achieve effective training, and their interpretability can pose challenges due to their black-box nature.
Final thoughts
Customer churn prediction is crucial for subscription-based companies, as it serves as an indicator of their business’s health. Through harnessing the capabilities of AI and ML, businesses can gain a valuable understanding of customer behavior and proactively pinpoint customers who are at risk of churn. This empowers companies to address product or pricing plan weaknesses, operational issues, and customer preferences, reducing churn and increasing customer satisfaction.
A critical factor in successful churn prediction is the careful selection of data sources and observation periods. Having a comprehensive view of the customer’s history of interactions enables the development of more accurate predictive models. The process of feature selection is equally important, as the quality and relevance of the dataset directly impact the precision of churn forecasts. Companies can achieve more precise predictions and make data-driven decisions using qualitative datasets.
Customer segmentation plays a significant role in optimizing churn prediction efforts. Large companies with diverse customer bases and multiple offerings can benefit from segmenting customers based on behavior or demographics. Segment-specific customer churn prediction models can then be deployed, tailoring retention strategies to each group’s unique characteristics and needs.
The work doesn’t end once the churn prediction models are deployed. Data scientists must continually monitor the models’ performance and be prepared to revise and adapt features as customer behaviors change. Maintaining the desired level of prediction accuracy requires continuous adjustments and updates to the models.
AI and ML have significantly impacted the way businesses handle customer churn prediction. Companies can obtain invaluable insights into customer behaviors and preferences by analyzing extensive volumes of customer data. With invaluable insights into customer behaviors, businesses can implement proactive retention strategies, optimize customer experiences, and ultimately increase customer loyalty and long-term value. With the continuous evolution of technology, AI and ML will become increasingly instrumental in enabling businesses to excel in a competitive and customer-centric environment.
Boost your business’s success with AI-powered customer churn prediction! Contact LeewayHertz’s AI experts to design and develop a churn prediction model for your business.
Start a conversation by filling the form
All information will be kept confidential.