AI in data analytics: Unlocking the future of decision-making
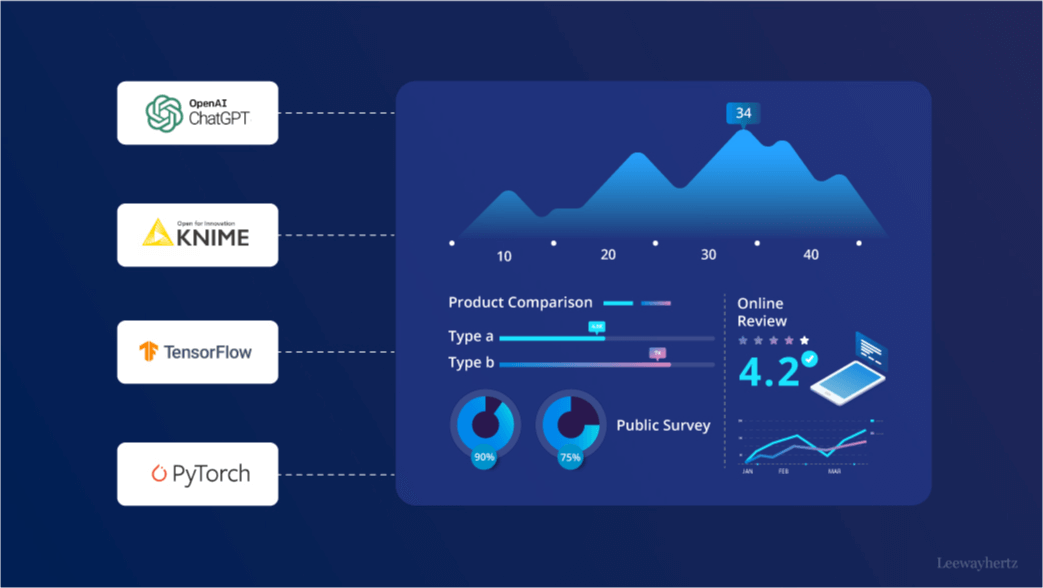
In today’s digital age, the world generates an astonishing amount of data that continues to grow at an unprecedented rate. Data analytics aims to harness this flood of information, turning raw data into actionable insights that fuel decision-making and enhance business performance. However, navigating this sea of data is no simple feat. It requires meticulous collection, processing, and analysis of data for which human efforts can sometimes fall short, leading to inefficiencies or inaccuracies. Enter the power duo: Artificial Intelligence (AI) and Machine Learning (ML). As extensions of the analytical human mind, these technologies dive deep into data, automating intricate workflows and showcasing adaptive learning and reasoning traditionally seen in human cognition.
Imagine a team of top-tier data scientists tirelessly sifting through data around the clock. That’s AI analytics for you— a facet of business intelligence where software mirrors human-like learning and reasoning in data analysis, testing every conceivable combination to identify relationships between data points. The result? Astounding speed, depth, and accuracy in data interpretation, something traditional analytics methods could only dream of.
The influence of AI and ML isn’t just confined to boardroom discussions. It’s tangible, seen in daily interactions, especially in digital advertising. Think of the last time you browsed online, only to be met with surprisingly accurate product advertisements based on your recent searches. Platforms like Google Ads utilize the prowess of AI and ML to discern user preferences, crafting personalized ad experiences that resonate with individual tastes.
Furthermore, the challenge of deciphering ‘Big Data,’ previously deemed impossible by conventional data tools, is now within grasp, thanks to AI advancements. This newfound capability ensures businesses mine data and unearth golden nuggets of insights from it— insights that guide marketing strategies target potential leads, and shape future trajectories.
This article explores the transformative power of AI in data analytics, from streamlining workflows to predicting intricate market trends across various industries. You will also learn about its advantages, diverse use cases, and role in healthcare, e-commerce, telecom and other major sectors. As we delve deeper into this article, we will unravel the multifaceted impact of AI on data analytics, offering a glimpse into a future where decisions are not just data-driven but AI-empowered.
- What is data analysis?
- Why use AI in data analytics?
- How is AI in data analytics used?
- How AI and ML can improve your data analytics workflow: A step-by-step process
- Benefits of AI in analytics
- How does AI data analysis differ from traditional data analysis?
- Use cases of AI in analytics
- How does LeewayHertz’s generative AI platform elevate data analytics processes?
- The impact of AI in data analytics across major industries
What is data analysis?
Data analysis is the practice of examining data to uncover patterns and insights in it. It is a crucial data analytics component and involves descriptive and predictive analysis. Descriptive analysis looks at current data to describe trends, while predictive analysis uses past and current data to forecast future events.
Businesses often face challenges in accessing and analyzing relevant data. While data may be available, companies may lack the necessary tools to process it. The data may be spread across multiple sources, requiring collection and conversion into a common format for analysis. Data gathering can sometimes be more difficult and time-consuming than the actual analysis, especially when it involves manual processes that are not easily repeatable.
According to Gartner’s Ascendency Model, there are four main types of data analytics:
- Descriptive analytics – This type of analysis answers questions like “how many, when, where, and what.” Business Intelligence (BI) tools and dashboards primarily focus on this type of analytics. It can include ad hoc reports created on-demand or canned reports following a template. [What Happened?]
- Diagnostic analytics – This type of analysis seeks to uncover the reasons behind certain events or trends by examining data to understand cause-and-effect relationships. [Why did it happen?]
- Predictive analytics – This type of analysis identifies trends, correlations, and causations through predictive and statistical modeling. [What will happen?]
- Prescriptive analytics – This type of analysis combines AI and big data to predict outcomes and recommend actions to achieve desired results. [How can we make it happen?]
Each of these types of data analysis serves a specific purpose, with some focused on understanding the past and others on anticipating the future. Regardless of the type, the primary objective of a data analyst is to leverage data to enhance efficiency and performance by identifying patterns.
Seven technologies are key to data analytics and Big Data:
- Predictive analytics: These are statistical algorithms that analyze data to predict future outcomes based on historical data.
- Hadoop: A software framework designed for processing and storing large amounts of data.
- Data mining: The examination of vast data sets to discover relationships and patterns.
- Machine learning: A subset of AI that enables computers to learn from experience, allowing them to create models that analyze large data sets and deliver more accurate results.
- Text mining: Similar to data mining, but focused on analyzing text to gain insights and understanding, often linked with Natural Language Processing (NLP).
- In-memory analytics: Analysis of data in system memory to obtain insights.
- Data management: Enhancing data storage, quality, and organization within organizations.
Optimize Your Operations With AI Agents
Optimize your workflows with ZBrain AI agents that automate tasks and empower smarter, data-driven decisions.
Why use AI in data analytics?
AI in data analytics stands as a transformative force for businesses, streamlining the process of harnessing vast troves of information. At its core, AI is designed to replicate human intelligence, allowing machines to function with a resemblance of human thought and decision-making. Unlike traditional business intelligence, which bases its insights on historical data, AI-driven analytics leans into the future, predicting behaviors and discerning upcoming trends.
Key components of this advanced analytical approach include predictive modelling, which uses AI to anticipate outcomes based on historical patterns; machine learning, which automates these predictions and adjusts based on new data; NLP, which bridges human language and machine comprehension; and data mining, which digs deep into datasets to uncover hidden patterns and correlations.
As businesses grapple with ever-expanding data, they turn to AI analytics to carve out actionable insights. This approach facilitates refined product development, sharper team performance, and a clear understanding of what strategies hit the mark.
Beyond just processing data, AI-driven systems translate raw numbers into meaningful insights with minimal human intervention. Whereas traditional software demands constant tweaks and adjustments, machine learning, a subset of AI, evolves autonomously after its initial setup, ensuring its adaptability. This self-reliant nature of AI significantly accelerates data processing and enhances precision.
Moreover, the fusion of AI into marketing has been a game-changer. It’s empowered businesses to automate complex procedures, curate personalized content, and forecast with unmatched precision, making AI an indispensable tool in modern data analytics.
How is AI in data analytics used?
AI-driven data analysis employs artificial intelligence techniques in conjunction with data science to streamline data processing, making it more efficient. The core aim is to extract critical information from both structured and unstructured data, which can aid in making insightful conclusions and strategic decisions. This means businesses no longer rely solely on human intervention for data interpretation; AI can autonomously spot trends and produce results based on training.
It’s vital to differentiate between data analytics and data analysis. While they may seem synonymous, data analytics is a sub-branch of business intelligence, relying on machine learning to gain insights. In contrast, data analysis involves inspecting, cleaning, and then interpreting data to yield valuable insights. The process can be summarized in four steps: data collection, data cleaning, data analysis, and data interpretation.
Various AI techniques facilitate these processes:
- Machine Learning (ML): ML algorithms are utilized to sift through vast data amounts, identifying patterns and shaping AI models based on the provided data. The model’s performance depends on the relevancy of the data it’s trained on, e.g., using social media data for sentiment analysis.
- Natural Language Processing (NLP): NLP bridges computers and human language. It enables AI systems to interpret vast text amounts, derive meanings, and even generate new content based on user input.
- Computer vision: This focuses on enabling computers to understand and interpret visual data, such as images and videos. AI can detect patterns, identify objects, and even track motion by analyzing such data.
- Text analysis: Leveraging a subset of ML known as NLP, text analysis breaks down text data, extracting unprecedented insights. By analyzing unstructured text, this method provides qualitative insights. One can use it to detect sentiment, extract keywords, classify topics, and more.
For instance, sentiment analysis categorizes opinions into positive, negative, or neutral. By using AI tools, businesses can train sentiment analyzers specific to their needs. Text classification and extraction techniques further allow for detailed analysis and information extraction.
Additionally, AI bots, commonly associated with chat interactions, are expanding their reach into data analysis. Thanks to their constant learning from accumulated data, their analytical capabilities always evolve, often surpassing human analysts in speed and efficiency.
Lastly, AI-powered predictive analysis stands out as a prominent feature. Unlike traditional methods, AI can process vast data sets, discern patterns, and predict future events. The synergy of large data sets, formidable processing power, and the self-learning nature of machine learning allows businesses to make forward-looking decisions, ensuring they remain at the forefront of their industries.
How AI and ML can improve your data analytics workflow: A step-by-step process
In today’s data-driven world, optimizing data analytics workflows is paramount. Here’s how integrating AI and ML can transform this process, making it more efficient and precise:
Data preparation
- Automated cleaning: Traditional data preparation can be tedious. AI and ML algorithms can identify and correct errors in datasets, ensuring data quality.
- Data integration: By recognizing patterns, ML can automate merging and integrating data from different sources, saving time and ensuring consistency.
- Handling missing values: AI can predict and fill missing values based on existing data patterns instead of manual imputation.
- Normalization & standardization: AI tools can automatically identify which data needs to be normalized or standardized, making datasets more comparable and suitable for analysis.
Data exploration
- Enhanced visualization: AI can help generate interactive dashboards that adjust based on user queries, offering dynamic insights.
- Pattern recognition: Using ML, vast amounts of data can be sifted through to uncover hidden patterns and insights that human analysts might overlook.
- Feature selection & engineering: ML can prioritize and transform features most relevant to the analytical model, enhancing its performance.
Data modeling
- Model selection: AI can automate the process of selecting the most appropriate modeling technique based on the nature of the data and the problem.
- Hyperparameter tuning: Instead of manual trial and error, ML algorithms can automatically optimize parameters, refining the model for better accuracy.
- Evaluation: AI tools can provide real-time feedback on model performance, allowing for faster iterations and improvements.
Data communication
- Natural language generation: AI can automatically transform complex data results into clear, natural language narratives, simplifying interpretation.
- Translation & multilingual support: AI-driven tools can instantly translate findings into various languages, broadening reach.
- Voice-enabled reporting: AI-driven speech synthesis can allow stakeholders to “listen” to insights, enhancing accessibility.
Data security
- Predictive threat analytics: ML models can predict potential security threats by analyzing patterns and strengthening defenses.
- Encryption & authentication: AI can be used to develop more sophisticated encryption techniques and ensure data access only by authenticated users.
- Real-time anomaly detection: By continuously monitoring data traffic, AI can instantly detect and alert any suspicious activities, ensuring immediate response.
Data innovation
- Generative models: ML models, especially Generative Adversarial Networks (GANs), can be used to create synthetic datasets, useful in scenarios where real data is limited.
- Personalization: Recommender systems driven by ML can offer users highly personalized product or content suggestions based on past behaviors and preferences.
- Advanced visual recognition: Advanced ML models can recognize not just objects but also emotions, activities, and more, offering deeper insights and innovative applications.
Incorporating AI and ML into the data analytics workflow results in faster, more accurate insights, transforming how businesses understand and leverage their data.
Optimize Your Operations With AI Agents
Optimize your workflows with ZBrain AI agents that automate tasks and empower smarter, data-driven decisions.
Benefits of AI in analytics
Streamlined automation
By harnessing AI within analytics, tasks that once required manual intervention from data specialists can now be seamlessly automated. This diminishes the risk associated with human errors and biases and conserves valuable time. By taking over routine analysis, AI empowers analysts to channel their expertise towards more intricate analytical endeavors and meaningful data narration.
Enhanced operational speed
Thanks to the inherent capabilities of AI, vast volumes of data can be parsed and scrutinized at a pace unattainable by human efforts alone. This rapidity ensures that actionable insights are generated promptly. Furthermore, AI’s real-time data processing prowess facilitates quick business reactions, giving companies a competitive edge.
Augmented data precision
AI’s advanced algorithms can delve deep into data sets, recognizing intricate correlations or patterns that might elude manual analysis. This depth of analysis ensures reduced human error during data processing and fosters the generation of sharper insights. Moreover, these algorithms are designed to evolve, refining their accuracy as they ingest new data continuously.
Forward-looking insights
With AI-powered predictive analytics, businesses are positioned to forecast future trends, empowering them to strategize proactively. Such foresight elevates risk management, enabling potential challenges to be identified and addressed preemptively to curb potential setbacks. Furthermore, AI-driven predictive models can be tailored to discern specific patterns or behaviors, amplifying their precision and bolstering the robustness of their foresight.
Incorporating AI into analytics creates a paradigm shift, transforming data into a potent tool that drives actionable and accurate business strategies.
How does AI data analysis differ from traditional data analysis?
The evolution of data analysis has seen a significant shift with the introduction of AI. Here’s how AI-based data analysis varies from its traditional counterpart:
- Manual versus automated processes: Traditional data analysis methods predominantly require human intervention. Data analysts manually sift through and interpret data patterns, a process that can be challenging, especially with expansive data sets. AI, augmented with machine learning, streamlines this process, offering automation that quickens data processing and often does so with enhanced precision.
- Data processing capacity: Traditional systems have their limitations when dealing with extensive data due to constraints in processing speed and storage capabilities. AI-driven analytics, in contrast, thrives on big data, processing vast quantities rapidly, making it apt for real-time or near-real-time evaluations.
- Type of data handled: The conventional analytics landscape is primarily equipped to handle structured data neatly categorized into rows and columns. AI’s forte lies in its capability to manage both structured and unstructured data, encompassing text, images, videos, and audio. This gives AI an edge in delivering a holistic data analysis.
- Predictive insights: While traditional methods can identify existing patterns, AI goes a step further. It recognizes intricate patterns and offers predictive insights that might elude manual scrutiny.
- Self-reliance of systems: Traditional data systems are largely dependent on continuous human inputs. AI is designed to be self-sufficient, especially when complemented by machine learning. After the initial setup and training, these systems can operate autonomously, interpreting fresh data and providing insights. This autonomy ensures consistent analysis and frees up human resources for more strategic roles.
While traditional data analysis has its merits and applications, AI augments these capabilities, pushing the boundaries of what’s possible in data interpretation and application.
Real-world applications: Giants like Walmart and Amazon have tapped into AI-powered analytics to refine customer experiences. Walmart Global Tech, for instance, harnesses AI to fine-tune various facets of its operations, from inventory management to personalizing shopping encounters. Similarly, Amazon leverages AI to craft product recommendations that resonate with individual user preferences, factoring in aspects like buying history and product reviews.
Use cases of AI in analytics
Sentiment analysis
Within the spectrum of data analytics, sentiment analysis is pivotal in understanding the public’s feelings and opinions on a specific subject. This involves processing vast volumes of textual data to extract discernible sentiments, enabling businesses to gain insights into their users’ perceptions and experiences. Data analytics is the linchpin that drives the success of sentiment analysis. At its core, sentiment analysis is a specialized subset of data analytics tailored to interpret and classify emotions within textual data.
Technically, advanced algorithms trained on extensive datasets enable this function. These algorithms are adept at differentiating sentiments, categorizing them as positive, negative, or neutral based on linguistic patterns and contexts.
Take the case of platforms like Netflix. User feedback, reviews, and comments provide a goldmine of data. These textual interactions are systematically parsed through sentiment analysis, breaking down words, phrases, and their inherent sentiments. The aim is not merely to identify emotions but to translate them into quantifiable metrics to drive decision-making.
For instance, recurring negative sentiments about a particular feature on Netflix could be indicative of its inefficiency or unpopularity. Conversely, frequent positive sentiments suggest successful implementations or popular content.
By employing sentiment analysis, companies can pinpoint specific areas of improvement and act accordingly, ensuring an optimized user experience. In this manner, sentiment analysis transcends mere emotion recognition, representing a powerful tool for strategic refinement in the digital landscape.
Predictive analytics and forecasting
Predictive analytics stands at the confluence of data analytics and artificial intelligence. It leverages the capabilities of AI to process vast amounts of historical and real-time data to make informed predictions about future events.
Predictive analytics, underpinned by AI, commences with aggregating and preprocessing data, ensuring it’s cleaned and structured optimally for analysis. Crucial features or variables that significantly impact future outcomes are then identified through feature selection and engineering. Following this, machine learning algorithms are trained on historical data to comprehend the relationships between these features and past events. To guarantee the accuracy of predictions, the models are rigorously validated using separate datasets, often employing techniques like cross-validation. Once fine-tuned, these models sift through current data, recognizing patterns to anticipate future trends. Ultimately, the forecasts made are transformed into actionable insights, aiding businesses in informed decision-making.
Using the case of Bank of America as an illustration, AI-powered predictive analytics delves deep into the intricate dynamics between Equity Capital Markets (ECM) deals and investor behaviors. The AI system can predict which pitches are most likely to strike a chord with investors by analyzing past interactions, market sentiments, and deal outcomes. This technical prowess offers a competitive advantage and streamlines efforts to resonate more effectively with the target audience.
Anomaly detection and fraud prevention
Using AI in anomaly detection and fraud prevention is a multi-step, intricate process. Initially, vast datasets are collated that undergo preprocessing to ensure they are structured for effective analysis. Within this data, certain pivotal features, such as transaction frequencies or IP addresses, are extracted by AI algorithms. Machine learning models are then trained on past datasets, where known fraudulent activities are clearly labeled, equipping them to discern deviations from typical behaviors. These AI systems vigilantly scrutinize incoming data in real-time operations against their trained knowledge. Any patterns that deviate beyond set thresholds are immediately flagged as potential anomalies. This proactive detection becomes more refined over time, thanks to a feedback loop where confirmed fraud instances are continually integrated into the model’s learning process.
Using Spotify as an illustrative example: To safeguard artists’ legitimate earnings, the platform employs AI to analyze streaming data, focusing on listening patterns and IP addresses. When the AI detects patterns reminiscent of bot-driven streams or suspiciously high plays from certain IPs, it takes preventive measures. Thus, through a combination of data analytics and AI, Spotify ensures genuine plays are acknowledged, upholding the platform’s integrity and fairness.
AI can process and analyze visual content, determine patterns, identify objects, or even detect abnormalities. For example, Walmart uses AI to scrutinize videos and images for inventory management and prevention of theft. Their systems can recognize products on store shelves, monitor stock levels, and identify potential shoplifting.
Discovering new insights
In the vast landscape of data analytics, one of the most transformative applications of artificial intelligence is its unparalleled ability to uncover new insights. Modern data’s sheer volume and complexity often contain submerged patterns and subtleties that, while elusive to human analysts, are readily discernible to well-tuned AI systems.
At the outset, the process begins with acquiring and preprocessing massive datasets. During this phase, redundant, irrelevant, or erroneous data points are filtered out, and the remaining data is structured in a manner accommodating to deep analysis. Once prepared, advanced machine learning models, especially those equipped with deep learning capabilities, dive into this data. These models have been trained, validated, and tested on diverse datasets, enabling them to recognize and interpret intricate patterns.
When posed with specific queries, such as analyzing the effectiveness of different marketing channels, these AI algorithms sift through the data, assessing metrics, correlations, and causative factors. They don’t just identify the channel with the highest conversion rate but can also provide context around why that might be the case. Is it due to the time of day? The demographic being targeted? Or perhaps a recent event that boosted that channel’s visibility?
Moreover, AI’s predictive capabilities can foresee emerging trends, allowing businesses to stay a step ahead. These systems not only answer questions but can proactively offer suggestions. By analyzing past and present data, they might detect a rising interest in a particular product category or a shift in user behavior. These insights can then be translated into actionable recommendations, such as adjusting marketing strategies, reallocating resources, or introducing new offerings.
To illustrate, consider an AI tool analyzing website traffic. While a human analyst might see increased traffic and higher conversions, the AI system delves deeper. It identifies nuances like the correlation between a recent social media campaign and a surge in website visits or how a change in website layout led to better user engagement. By highlighting these underlying factors, AI ensures that data-driven decisions aren’t just informed but also strategic and forward-thinking.
Predicting business and marketing outcomes
In the dynamic realm of business and marketing, having the ability to foresee outcomes can be a significant competitive advantage. With its robust data analysis capabilities, artificial intelligence is transforming this predictive landscape.
The journey begins with data acquisition. In today’s digital age, businesses generate and have access to vast amounts of diverse data. This data can range from customer interaction logs, purchase histories, and social media metrics to external market trends and competitor analyses. Given the heterogeneity and volume of this data, preprocessing becomes a pivotal first step. This involves cleaning, normalizing, and structuring the data to make it suitable for analysis.
Once the data is primed, AI algorithms take center stage, particularly those leveraging machine learning. These algorithms are trained on historical data where past outcomes are known, learning intricate relationships between different variables and their impact on desired results. This training involves techniques such as regression analysis, clustering, and classification. For instance, a machine learning model might analyze how past marketing campaigns influenced sales or how changes in product features affected customer satisfaction.
However, AI’s true power lies in its capacity to not just understand past events but to use this understanding to predict future scenarios. AI in data analytics can provide valuable predictions by evaluating current data and contrasting it with historical patterns. It can forecast how a new product might be received in the market, which marketing channels are likely to yield the highest ROI in the upcoming quarter, or even how shifts in global trends might influence local consumer behaviors.
Take, for instance, an organization looking to launch a new product. An AI-driven system can predict potential reception scenarios by analyzing past product launches, market sentiment, competitor positioning, and current consumer preferences. It can provide insights into optimal pricing strategies and marketing channel allocation and even suggest tweaks in product features to better cater to the target demographic.
AI doesn’t just crunch numbers; it synthesizes a holistic understanding from diverse data sources, offering businesses a forward-looking lens. With such insights, companies can make strategic decisions, mitigating risks and maximizing opportunities, ensuring that their business and marketing ventures are data-informed and future-ready.
Unifying analytics and customer data
In today’s fragmented digital landscape, businesses often grapple with data sprawled across various platforms and sources. Enter artificial intelligence, a game-changer in synthesizing disparate data into a coherent narrative.
The process begins with data integration. Given the diverse nature of sources, data comes in different formats – from structured databases like CRM systems to unstructured data such as social media interactions or telephonic communication logs. The first challenge, therefore, is to standardize and cleanse this data, ensuring compatibility and accuracy. AI, equipped with advanced preprocessing tools, aids in this conversion, turning discordant data into a consistent format.
Once standardized, AI systems employ data fusion and feature extraction techniques. Data fusion involves the combination of data from multiple sources, ensuring there’s no redundancy. Feature extraction, on the other hand, pinpoints critical attributes from this unified data set, allowing for the creation of comprehensive customer profiles. Machine learning algorithms, especially clustering and classification models, further categorize these profiles, identifying common patterns or segments.
One of the notable capabilities of AI in this domain is its ability to handle and make sense of challenging data sources. Consider telephonic communication logs. While to a human, these logs might seem like a simple record of calls, AI systems, using NLP, can delve deeper. They can transcribe, analyze, and even derive sentiment from these conversations, integrating these insights with data from other sources, such as purchase histories or website visits.
The outcome of this intricate process is a holistic view of a customer. For instance, a business using an AI-driven data unification system can tell if a customer who frequently interacts on their social media also recently called their helpline and expressed dissatisfaction. Such a consolidated view is invaluable. It gives businesses a 360-degree understanding of their customers, enabling personalized marketing strategies, improved customer service, and more informed business decisions.
Hence, AI’s ability to unify analytics and customer data isn’t just about merging information. It’s about transforming isolated data points into actionable insights, ensuring businesses are better equipped to cater to their customer’s evolving needs and preferences.
Demand forecasting
Understanding future demand in the contemporary business environment is pivotal for efficient operations and strategic planning. Through the lens of AI, demand forecasting transforms from a mere extrapolation of trends to a sophisticated analysis rooted in multiple facets of data.
The journey of AI-enabled demand forecasting commences with data aggregation. Comprehensive datasets encompassing past sales, current stock levels, customer inquiries, and other relevant metrics are pooled together. Given the diversity of these sources, data preprocessing becomes indispensable. During this phase, inconsistencies, outliers, or missing values within the datasets are addressed, ensuring a robust foundation for subsequent analyses.
Post-preprocessing, AI employs machine learning models tailored for time series forecasting. Techniques such as ARIMA (Auto Regressive Integrated Moving Average), LSTM (Long Short-term Memory Networks), and Prophet, among others, are utilized. These models are adept at recognizing patterns, seasonality, and trends in historical data and extrapolating them into the future. But the sophistication doesn’t end there. To fine-tune their predictions, these algorithms can simultaneously factor in external variables, such as promotional activities, economic indicators, or even meteorological data.
Moreover, AI’s neural networks can analyze the non-linear relationships between variables, such as how a combination of seasonal trends and promotional activities might affect demand. By assessing these intricate interactions, AI ensures that the generated forecasts are accurate and nuanced.
For instance, consider a retail business aiming to optimize its inventory. By harnessing AI-driven demand forecasting, it can anticipate the quantity and the variety of products that will likely be in demand. Based on predictions, decisions can be made regarding which products to stock up on, which ones to offer promotions, or even which products might need repositioning in the market.
Furthermore, these forecasts can influence broader business strategies. Suppose an AI model predicts a surge in demand for a particular product category. In that case, resources can be reallocated, manufacturing can be ramped up, and marketing campaigns can be designed to capitalize on this trend. Conversely, measures can be taken to diversify offerings or invest in customer engagement activities if a dip is anticipated.
Demand forecasting has evolved into a strategic tool through the synergy of data analytics and AI. It predicts future demand and empowers businesses with actionable insights, enabling them to navigate the market landscape proactively and efficiently.
How does LeewayHertz’s generative AI platform elevate data analytics processes?
LeewayHertz’s generative AI platform, ZBrain, plays a transformative role in optimizing data analytics processes that empower organizations to develop actionable and precise business plans. As a comprehensive, enterprise-ready platform, ZBrain empowers businesses to design and implement applications tailored to their specific operational requirements. The platform uses clients’ data, whether in the form of text, images, or documents, to train advanced LLMs like GPT-4, Vicuna, Llama 2, or GPT-NeoX for developing contextually aware applications capable of performing diverse tasks.
In the dynamic field of data analytics, enterprises face challenges that range from the manual interpretation of data patterns to limitations in processing speed and storage capabilities, dealing exclusively with structured data, and a heavy reliance on continuous human inputs. ZBrain effectively addresses these challenges through its distinctive feature called “Flow,” which provides an intuitive interface that allows users to create intricate business logic for their apps without the need for coding. Flow’s easy-to-use drag-and-drop interface enables the seamless integration of large language models, prompt templates, and other generative models into your app’s logic for its easy conceptualization, creation, or modification.
To comprehensively understand how ZBrain Flow works, explore this resource that outlines a range of industry-specific Flow processes. This compilation highlights ZBrain’s adaptability and resilience, showcasing how the platform effectively meets the diverse needs of various industries, ensuring enterprises stay ahead in today’s rapidly evolving business landscape.
ZBrain apps enable organizations to streamline automation, heighten operational speed, enhance data precision, gain predictive insights, and make rapid business decisions, all while upholding stringent data privacy standards. This leads to mitigated risks associated with human errors, reduced bias, time efficiency, rapid parsing of extensive data volumes, and improved risk management, enabling proactive trend forecasting and strategy formulation.
The impact of AI in data analytics across major industries
Healthcare and pharma
Incorporating AI in data analytics has instigated transformative advancements in the healthcare sector. One of the most striking aspects of this transformation is how AI can sift through vast volumes of healthcare data, presenting possibilities from drug discovery to enhanced diagnostic methods.
One prominent application is in the realm of medical imaging. AI-powered systems can scrutinize images—be it X-rays, MRIs, or CT scans—and pinpoint irregularities that may suggest a developing ailment. This broadens the scope of identifying diseases at an early stage and offers a more efficient process. Thanks to AI’s computational prowess, these analyses can be done quickly and precisely, often surpassing human capabilities. This accelerated and enhanced diagnostic capability aids clinicians in making swift and accurate decisions. A testament to this capability is a company like Clearly, which leverages AI to aid physicians in detecting potential cardiac anomalies.
Beyond diagnostics, the pharmaceutical realm is also undergoing a seismic shift due to AI in data analytics. Many of the leading pharmaceutical entities are redirecting their investments towards AI-driven research. Such systems have the capability to delve into intricate datasets, examining aspects like disease pathways, interactions of drugs, and specific patient profiles. This deep dive can unearth potential drug candidates or even discover novel applications for pre-existing drugs. Such methodologies expedite the drug discovery process, potentially leading to more effective treatments and cures for various ailments.
As AI and data analytics continue to integrate more closely with healthcare, the industry benefits immensely in terms of enhanced patient care and innovative medical breakthroughs.
Optimize Your Operations With AI Agents
Optimize your workflows with ZBrain AI agents that automate tasks and empower smarter, data-driven decisions.
Finance and banking
The intersection of AI and data analytics is reshaping the landscape of the finance and banking sectors. With the vast amount of data that these industries grapple with daily, the integration of AI offers a pathway to enhanced efficiency and accuracy in many of their operations.
A major application of AI in data analytics in finance is in the domain of fraud detection. Sophisticated fraud schemes continually threaten modern banking systems, but AI can analyze transaction patterns in real time, identifying anomalous behaviors that might indicate fraudulent activity. This proactive approach can mitigate potential financial losses and protect customer assets.
Credit assessment, which traditionally demands an in-depth exploration of an individual’s financial history, has been streamlined by AI. The sheer volume of data involved in these assessments made the process time-consuming in the past. However, solutions like the Lender Toolkit utilize AI-driven algorithms to expedite the underwriting process. By parsing and analyzing financial histories more efficiently, these tools can speed up loan approvals, benefiting both lenders and borrowers.
Moreover, when it comes to investment, AI is a game-changer. It can rapidly sift through vast repositories of market data, including historical trends and real-time market fluctuations, to offer insights into potential investment avenues. AI can preemptively identify risks or opportunities that might elude human analysts by evaluating a broader spectrum of economic indicators.
AI’s presence in finance and banking data analytics is not just evolutionary but transformative. It is ushering in an era where processes are accelerated and significantly more accurate, ensuring that financial institutions can offer optimized services to their clients.
E-commerce and retail
Integrating AI with data analytics is reshaping the outlines of the retail and e-commerce sectors. Amidst the vast amounts of data generated daily, businesses in these domains are turning to AI to capture, process, and extract actionable insights. Maintaining an in-depth, real-time understanding of industry dynamics and consumer behaviors has become necessary for these industries, and AI is the key facilitator. For instance, once a challenging domain fraught with risks of overstocking or stockouts, inventory management is now made more efficient with tools like C3 AI. These tools employ AI-driven algorithms to forecast demand, ensuring retailers have just the right stock levels.
Another crucial dimension AI in data analytics brings to the table is sentiment analysis. By systematically processing consumer reviews and feedback, AI can identify patterns that inform retailers about customer perceptions, preferences, and concerns, enabling them to tailor their strategies accordingly.
Beyond these, AI enables enhanced customer segmentation by analyzing datasets to categorize consumers based on behavior, preferences, and demographics. This granular segmentation aids in crafting targeted marketing strategies, thereby improving engagement and conversion rates.
Additionally, the era of personal shopping experiences has been supercharged by AI. By harnessing this technology, retailers can offer product recommendations rooted in a customer’s browsing history and past purchases.
Furthermore, the predictive prowess of AI gives retailers an edge by forecasting sales trends, potential demand surges, or even upcoming consumer preferences, ensuring businesses remain agile and ahead of the curve.
In the vast realm of e-commerce, where transactional risks abound, AI stands as a sentinel by monitoring and analyzing transaction behaviors, effectively detecting and countering fraudulent activities. As AI continues to intertwine with retail and e-commerce analytics, it promises a future of optimized processes, efficient operations, and enhanced consumer experiences.
Manufacturing
In the intricate world of manufacturing, optimizing processes and ensuring the seamless functioning of equipment is paramount. The advent of AI in data analytics has ushered in a shift in this sector, enabling businesses to harness vast amounts of data to glean critical insights, thereby refining their operations.
One of the predominant challenges in manufacturing has always been equipment maintenance. While machinery is bound to degrade over time, determining the optimal moment for maintenance has historically been challenging. However, the rise of predictive maintenance tools, such as those presented by Augury, is changing this narrative. By leveraging AI-driven algorithms, these tools offer real-time insights into the health and performance of machinery, allowing businesses to anticipate potential failures well in advance and schedule timely interventions. This not only minimizes downtime but also ensures consistent quality and output.
Further enhancing the manufacturing landscape is AI’s role in optimizing supply chain operations. Comprehensive platforms like IBM Sterling Supply Chain Business Network empower businesses to understand their supply chains better. By analyzing multifaceted datasets, these tools can guide companies in areas ranging from inventory management to route planning. One of the most invaluable offerings is demand forecasting. By predicting future product demand based on historical data and current market trends, AI aids manufacturers in aligning their production schedules and inventory levels accordingly. This predictive capability ensures manufacturers can promptly meet market demands, reducing wastage and optimizing storage costs.
AI in data analytics transforms the manufacturing sector from the ground up. It promises a future where equipment runs optimally, supply chains are streamlined, and manufacturers can respond agilely to market demands, setting the stage for increased efficiency and profitability.
Marketing and CPG
In the dynamic realm of marketing and the Consumer Packaged Goods (CPG) industry, deriving actionable insights from customer data is crucial. While rich in functionality, traditional tools often grapple with the vastness of data, especially when campaigns scale up. The magnitude of information can become overwhelming, thereby obscuring critical insights.
Enter the transformative world of AI-driven solutions like Symantec. These platforms are designed to delve deep into the intricacies of big data, decoding customer preferences, spotting emerging trends, pinpointing customer challenges, and providing a comprehensive view of the competitive landscape. Armed with such profound insights, companies can refine their marketing strategies, ensuring they remain agile and responsive to evolving market dynamics.
Segmentation is a clear beneficiary of these newfound capabilities. Instead of deploying generic marketing initiatives, companies can now tailor their strategies. Leveraging AI-driven insights ensures that product promotions resonate powerfully with the intended audience. By keenly understanding the unique tastes and preferences of diverse customer segments, businesses can optimize engagement, maximize conversions, and ensure their marketing spend is both efficient and effective.
Further amplifying the potential of AI in marketing is the potent combination of machine learning and natural language processing. These technologies are especially adept at sifting through diverse and often unstructured data sources. Whether it’s feedback from e-commerce platforms, chatter on social media, or interactions across various omnichannel touchpoints, AI can distill meaningful insights, paving the way for more informed decisions.
In the CPG sector, the repercussions are multifaceted. Predictive analytics, supercharged by AI, provides brands with a foresight into product demand. This capability ensures that they can fine-tune their product offerings, optimizing inventory levels to match consumer expectations. The refined data analytics approach also helps craft targeted marketing campaigns, ensuring resonation with the desired audience segment. The personalization journey doesn’t end there. AI enhances the overall shopping experience by offering tailored product suggestions, fostering deeper customer loyalty. Lastly, determining the right price becomes pivotal in a marketplace that’s in perpetual flux. With its ability to gauge market conditions and consumer demand, AI guides brands in setting prices that drive sales while ensuring healthy profit margins.
Telecom
The telecommunications landscape is undergoing a paradigm shift spurred by the integration of artificial intelligence. With its unparalleled ability to deploy machine learning algorithms, AI has become a linchpin for telecom providers aiming to distill insights from massive datasets. Such advanced analytical techniques enable telecom companies to delve deeper into diverse areas, from user behaviors to the intricacies of network operations.
A paramount aspect of this AI-driven transformation is the rise of predictive analytics. The ability of AI to discern latent patterns in vast data repositories means that telecom providers can now anticipate and understand customer behaviors with heightened precision. This proactive recognition of trends empowers companies to refine their strategies, tailoring their services to align more closely with anticipated user needs and preferences.
Network efficiency is another sphere where AI’s impact is palpable. A meticulous analysis facilitated by AI brings to light any potential network performance issues or bottlenecks. This insight enables telecom operators to undertake targeted interventions, enhancing network performance. The ripple effect of these enhancements resonates with the end-users, who enjoy robust connectivity, diminished service disruptions, and an overall improved quality of service.
The arena of data collection has also seen revolutionary changes, all thanks to AI. Gone are the days when manual data collection was the norm. Today, AI-driven automated mechanisms ensure rapid and precise data gathering from various sources. This data, rich in volume and superior in accuracy, sets the stage for telecom providers to make more nuanced and informed decisions backed by big data engineering methodologies.
Furthermore, AI’s capabilities extend to optimizing customer outreach through sophisticated segmentation techniques. Telecom entities can design bespoke marketing strategies by categorizing users based on diverse metrics such as demographics, consumption patterns, and transactional histories. Such tailor-made campaigns invariably lead to heightened customer engagement, laying the foundation for sustained revenue growth.
Safety and security are at the forefront of AI’s offerings. With its razor-sharp ability to swiftly identify discrepancies, AI plays a pivotal role in fraud prevention. Whether it’s unauthorized data breaches or anomalies in billing, AI’s vigilance ensures these are promptly flagged. This proactive detection not only secures the telecom companies’ financial health but also instills confidence among users regarding their data safety.
Travel and hospitality
The travel and hospitality sector stands at the cusp of a transformation, largely driven by Artificial Intelligence (AI) innovations. As the industry grapples with the complexities of ever-evolving customer preferences and operational challenges, AI emerges as the beacon, offering tools rooted in sophisticated data analytics. This digital overhaul is not just limited to amplifying operational efficiencies; it promises to redefine customer experiences and catalyze business expansion.
At the heart of this transformation is the potential of AI to tailor customer experiences. In an industry where personalization can spell the difference between a loyal customer and a lost opportunity, AI’s ability to analyze vast swathes of customer data translates to experiences that resonate on a personal level. Whether it’s offering hotel room suggestions based on past preferences or curating travel itineraries that align with a traveler’s interests, AI-driven insights ensure that offerings are not just generic but meticulously crafted to individual tastes.
Marketing, too, is undergoing a renaissance in the hands of AI. Gone are the days of broad-spectrum campaigns that cast wide nets. AI-enabled data analytics allows travel and hospitality enterprises to segment their audience with surgical precision. By discerning patterns in travel habits, booking trends, and even online interactions, AI helps create marketing strategies that resonate deeply with targeted audience segments.
Operational efficiency, another cornerstone of the travel and hospitality industry, is being bolstered by AI’s analytical prowess. From optimizing booking systems to forecasting demands for specific travel periods, AI ensures businesses operate at peak efficiency. The insights generated can also guide logistical decisions, from staffing to inventory management in hotels, ensuring optimal resource utilization and cost efficiencies.
Endnote
In today’s rapidly evolving business ecosystem, the importance of AI-powered data analytics can’t be overemphasized. As industry leaders recognize the transformative potential of AI in shaping decisions and driving growth, investments in data analytics firms have surged. Such firms play a pivotal role, distilling actionable insights from the overwhelming data torrent enterprises grapple with daily. With AI at the helm, data gets seamlessly organized and becomes a potent tool for forecasting strategic pathways, ensuring businesses remain a step ahead in their quest for growth and enhanced returns on investment.
The beauty of AI-driven data analysis lies in its remarkable efficiency. Leveraging machine learning algorithms alleviates the hefty analytical burdens traditionally shouldered by human analysts. The resultant analyses are delivered at unprecedented speeds, scales, and depths, heralding an era where human limitations no longer confine data understanding.
Moreover, AI offers an unbiased lens unfettered by human assumptions or predispositions. This ensures an untainted and comprehensive data assessment, amplifying the accuracy of insights garnered. Couple this with the agility of machine learning algorithms, which adapt in real-time to shifting business terrains, and it’s evident that AI is not merely an analytical tool; it’s a dynamic partner continuously attuned to business rhythms.
As we stand at the cusp of this transformative phase in data analytics, it’s worth pausing and marveling at the AI-driven future we are ushering in— a future teeming with possibilities, precision, and unparalleled potential.
Unlock the future of data with AI! Trust LeewayHertz’s AI experts to transform your data analytics game with deep and meaningful data insights.
Start a conversation by filling the form
All information will be kept confidential.
Insights
AI for financial planning: Use cases, benefits and development
The integration of AI in financial planning is not just about automation but also about the sophisticated interplay of advanced technologies and data.
AI in treasury management: Applications, implementation, and future trends
AI has become an indispensable tool for modern treasury management, transforming the way organizations handle their financial operations.
Conversational AI: Use cases, types and solution
Conversational AI is a subset of artificial intelligence that enables human-like interactions between computers and humans using natural language.