Crafting an effective generative AI strategy: Steps, need, framework, best practices, and checklist
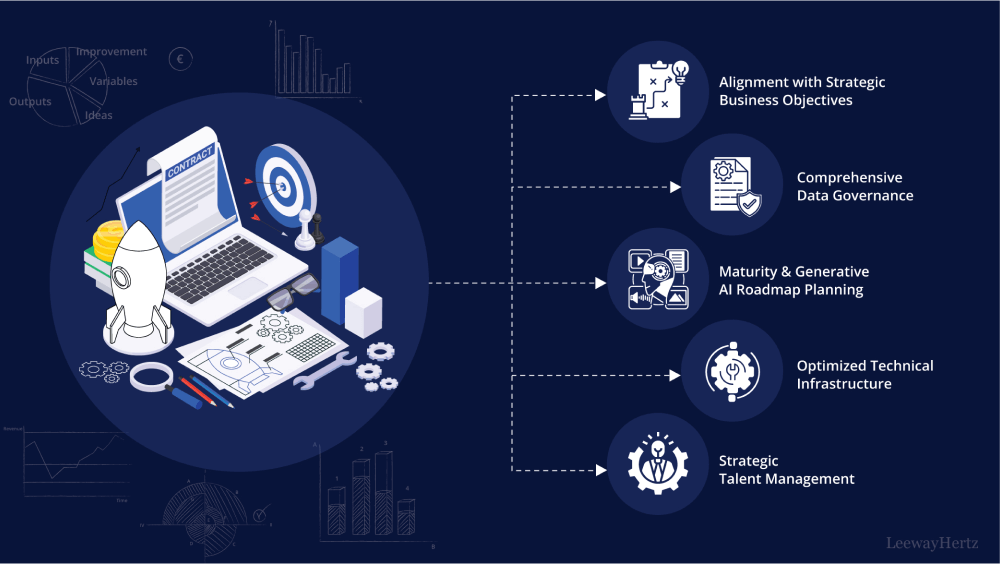
With the rapid advancement and adoption of generative AI, now is the ideal time for businesses to develop and implement a comprehensive generative AI strategy. This urgency is underscored by the competitive landscape, where many organizations are either already utilizing this technology or actively planning to integrate it into their operations.
Recent surveys by industry leaders like Scale AI and Gartner, Inc. have highlighted the widespread interest and investment in generative AI among business executives. For instance, over 80% of business leaders in a Scale AI survey reported having generative AI in production or are planning to experiment with it soon. Similarly, a Gartner poll revealed that the buzz around ChatGPT has spurred 45% of executive leaders to ramp up their AI investments, with 70% exploring and investigating generative AI’s potential.
These statistics are not just numbers; they reflect a transformative shift in how businesses interact with technology. The explosive growth of ChatGPT, which garnered over 100 million users shortly after its launch, underscores the profound impact and the high level of interest that generative AI commands. This technology is reshaping competitive dynamics and driving companies to innovate continuously.
Moreover, the broader adoption statistics from Salesforce’s survey illustrate the global engagement with generative AI technologies. With significant percentages of populations in countries like India, Australia, and the USA leveraging generative AI, the term “super-users” has emerged to describe individuals who frequently use and are mastering these technologies. Looking ahead, the potential for generative AI extends beyond immediate business applications. Bloomberg Intelligence forecasts that generative AI could generate an additional $280 billion in software revenue, driving vast opportunities for growth and innovation across various sectors.
In this context, developing a well-rounded generative AI strategy becomes not just beneficial but essential for businesses aiming to stay ahead in this dynamic and rapidly evolving landscape. This article aims to guide decision-makers through the process of establishing a generative AI strategy that aligns with business objectives and leverages the full potential of this transformative technology.
- Understanding generative AI and crafting an effective strategy
- Why a well-defined generative AI strategy is essential?
- Essential pillars of a winning generative AI strategy
- How to create a generative AI strategy?
- Understanding generative AI strategy framework for organizations
- Best practices for a robust generative AI strategy
- Business challenges in implementing generative AI strategy
- A strategic checklist for enterprise Generative AI strategy and implementation
- How does LeewayHertz help devise generative AI strategies for enterprises and startups?
Understanding generative AI and crafting an effective strategy
Generative AI, or Gen AI, is a transformative subset of artificial intelligence that autonomously generates new content from existing data. It can produce a diverse array of outputs, including text, images, videos, and even complex datasets, without directly copying the training data. Utilizing advanced algorithms and vast computing resources, Gen AI operates primarily on the principle of prediction, learning from broad datasets to create realistic, novel artifacts. This technology has wide-ranging applications, from creating natural language responses without coding to driving innovation in fields like drug development, chip design, and material sciences.
What is a generative AI strategy?
A generative AI strategy is a comprehensive plan tailored to leverage AI technologies to enhance a company’s strategic objectives and operational efficacy. It involves more than just adopting the latest AI tools; it requires integrating AI deeply into the business processes to boost productivity and innovation. Here are the core components of a successful generative AI strategy:
- Alignment with business goals: A generative AI strategy must start with a clear understanding of the company’s goals and how AI can enhance these objectives. It is crucial to identify specific generative AI use cases that complement and improve existing processes.
- Building an actionable roadmap: Assessing an organization’s current data and analytics capabilities is essential. This assessment helps create a prioritized action plan that includes the necessary tools, technologies, and training to advance to higher levels of data maturity and AI integration.
- Optimizing the technology landscape: Instead of focusing solely on new AI tools, the strategy should emphasize building a robust, scalable, and flexible technological infrastructure. This includes adopting cloud-native solutions and managing costs effectively as the company scales its AI operations.
- Establishing effective data governance: Implementing robust data governance is critical to managing the risks associated with generative AI. This includes ensuring the accuracy and security of AI-generated data and maintaining strict data privacy standards.
- Empowering the workforce: A successful generative AI strategy also involves preparing the workforce to utilize these new tools effectively. This preparation includes defining new roles and responsibilities and providing comprehensive training on the ethical use of AI technologies, as well as ensuring adherence to internal policies and external regulations.
By focusing on these strategic areas, businesses can harness the full potential of generative AI to streamline operations, foster innovation and maintain a competitive edge in the rapidly evolving digital landscape.
Why a well-defined generative AI strategy is essential?
In the rapidly evolving world of AI, a strategic approach to generative AI is not just beneficial—it’s essential for sustaining competitive advantage and driving meaningful business transformation. The emergence of generative AI as a powerful business tool highlights its potential to transform industries and redefine how we work. However, without a clear strategy, organizations risk falling behind, misusing resources, and failing to capitalize on AI’s transformative capabilities.
Key reasons to implement a generative AI strategy
Strategic alignment and risk management
- Competitive agility: A well-defined generative AI strategy ensures that a company can swiftly adapt to changes and challenges in the market while seizing emerging opportunities. Early adopters of generative AI have shown that strategic alignment with overarching digital goals can significantly enhance operational efficiencies and market positioning.
- Risk mitigation: Generative AI introduces new complexities, including ethical considerations and cybersecurity risks. A proactive strategy helps establish robust risk management protocols, ensuring that the adoption of AI technologies adheres to regulatory standards and ethical guidelines.
Operational efficiency and innovation
- Enhancing productivity: Companies with a clear AI strategy can better identify and implement AI-driven solutions that improve productivity. By focusing on high-value use cases, these organizations can ensure that their investments in AI yield substantial returns.
- Driving innovation: A strategic approach to generative AI encourages a culture of innovation. Organizations are prompted to experiment and explore new applications of AI across various functions, from product development to customer engagement, thereby unlocking new avenues for growth.
Sustainable growth and scalability
- Scalable solutions: With a generative AI strategy, businesses can build scalable AI solutions that grow with their needs. This scalability is crucial for maintaining efficiency as the company expands.
- Sustainability: A comprehensive AI strategy considers the long-term implications of AI, focusing on sustainable practices that benefit both the company and society.
Culture of innovation
- Encourage creative problem-solving: AI strategy helps cultivate an environment where employees are encouraged to think out of the box and approach challenges with innovative solutions, utilizing AI tools as enablers of creativity.
- Institutionalize experimentation: A comprehensive AI strategy makes experimentation a core part of the business process, allowing employees to test new ideas using AI without fear of failure, fostering a growth mindset throughout the organization.
Clear leadership
- Visible commitment from executives: Ensure that senior leaders endorse AI initiatives and are visibly involved in their development and deployment, reinforcing AI’s strategic importance across the organization.
- Leadership training and development: AI strategy equips leaders with the knowledge and skills necessary to drive AI initiatives, including understanding AI capabilities, strategic implementation, and managing AI-driven change.
Data strategy
- Comprehensive data accessibility: AI thrives on data. A robust data strategy is essential, ensuring you have access to the right data, in the right format, and with the appropriate governance and security measures in place. Develop a strategy to ensure all necessary data is accessible for AI applications, considering both current and future needs while maintaining compliance with data privacy laws.
- Data quality assurance: Implement processes to continuously assess and improve the data quality used for training and operating AI systems, ensuring the outputs are reliable and effective.
The strategic integration of generative AI into business operations is no longer an option but a necessity. Organizations must craft thoughtful, well-rounded AI strategies to navigate the complexities of AI adoption successfully and to leverage AI for substantial business gains. By focusing on alignment, innovation, workforce transformation, and sustainable growth, businesses can ensure they not only keep pace with technological advancements but also lead the way in their respective industries.
Essential pillars of a winning generative AI strategy
In this section, we outline five essential pillars that form the foundation of a robust generative AI strategy. These pillars ensure that AI initiatives are not only aligned with business objectives but also effectively managed and scalable.
Alignment with strategic business objectives
A generative AI strategy must be closely aligned with the organization’s strategic goals. This ensures that AI initiatives drive value where it is most needed and is coherent with the broader business agenda.
- Goal identification: Determine the specific business outcomes that generative AI can influence, such as customer experience enhancements or operational efficiencies.
- Strategic investment: Focus AI investments on areas with the highest potential for return, considering both short-term impacts and long-term transformation.
- Integration into corporate strategy: Embed AI initiatives within the fabric of overall business strategies, ensuring that AI advancements complement and accelerate broader business goals.
Maturity and generative AI roadmap planning
Understanding the current maturity level of your organization’s AI capabilities is essential for setting achievable goals and creating a realistic implementation roadmap.
- Current state analysis: Conduct an in-depth assessment of your existing technology, data infrastructure, and skill sets related to AI.
- Capability roadmap: Develop a phased roadmap that outlines critical milestones and timelines for advancing your AI maturity, tailored to your strategic business objectives.
- Progress tracking: Establish metrics and KPIs to monitor the effectiveness of AI implementations and ensure alignment with strategic goals.
Optimized technical infrastructure
An agile and scalable technical infrastructure is vital to supporting the demands of generative AI applications and adapting to evolving AI technologies.
- Scalable architecture: Build a modular and scalable infrastructure that can grow and adapt as AI needs to evolve.
- Technology selection: Choose technologies that not only meet current needs but also offer flexibility for future expansion and integration.
- Efficiency and cost management: Regularly evaluate and optimize AI operations to balance performance with cost efficiency, ensuring sustainable growth.
Comprehensive data governance
Effective data governance is crucial for managing data quality, security, and ethical use in AI applications.
- Governance framework: Implement a governance framework that addresses data quality, privacy, and security and is tailored to AI’s unique challenges.
- Ethical AI practices: Ensure AI systems operate within ethical boundaries, with mechanisms to address bias, transparency, and accountability.
- Adaptive policies: Develop flexible policies to adapt to new data types and AI models, maintaining robust governance as technologies evolve.
Strategic talent management
The success of AI initiatives also depends heavily on having the right talent equipped with the necessary skills and knowledge.
- Role definition and recruitment: Clearly define roles and responsibilities related to AI and recruit personnel with the desired skills.
- Training and development: Invest in ongoing training and development programs to keep your workforce adept at using AI technologies.
- Innovation culture: Foster a culture that encourages experimentation and innovation within the AI space, allowing employees to explore new ideas and applications.
By focusing on these five pillars, organizations can develop a generative AI strategy that not only aligns with their business goals but also effectively manages the complexities and opportunities presented by AI technologies. This strategic framework facilitates the responsible and effective deployment of AI, driving significant business value and competitive advantage.
How to create a generative AI strategy?
Building an effective generative AI strategy requires a holistic, cross-functional approach that aligns with your organization’s goals and objectives. Here are the key steps to follow:
Step 1: Define strategic objectives and opportunities
Clearly define the strategic objectives you aim to achieve by implementing generative AI. These could include goals such as accelerating product innovation, enhancing customer experiences, optimizing business processes, or boosting employee productivity. Establishing well-defined objectives will guide the subsequent steps of your strategy.
The first step in creating an effective generative AI strategy is identifying where the technology can have the most significant impact. Start by evaluating your organization’s core activities and pinpointing processes that can benefit from automation, enhanced creativity, or improved efficiency. Common areas include:
- Customer service: Implementing AI-driven chatbots can enhance customer interaction and response times.
- Marketing: Use AI to generate creative content or personalized marketing campaigns.
- Product development: Leverage AI for rapid prototyping or to explore new product ideas.
- Operations: Automate routine tasks and data analyses to increase operational efficiency.
Step 2: Conduct an AI readiness assessment
Evaluate your organization’s current state of AI readiness. This includes assessing your data infrastructure, computing resources, AI talent, and organizational culture. Identify gaps and areas that require improvement to support the successful adoption of generative AI technology.
Step 3: Identify high-value use cases
Conduct a comprehensive analysis to identify the specific use cases where generative AI can deliver the most value to your organization. Prioritize these generative AI use cases based on factors such as potential impact, feasibility, and alignment with your strategic objectives.
Step 4: Plotting an adoption roadmap
With potential opportunities identified, the next step is developing a phased adoption roadmap. This roadmap should outline how generative AI will be rolled out across the organization, prioritizing areas with the highest return on investment and the lowest barrier to entry. It’s crucial to take an iterative approach, starting with pilot projects and gradually scaling up as you gain experience and refine your strategy. The roadmap should also include:
- Pilot projects: Start with small-scale projects to gauge the technology’s impact and refine your approach based on these initial experiences.
- Expansion plans: Define criteria for scaling successful initiatives and integrating them into broader business processes.
- Timeline and milestones: Set realistic timelines for each adoption phase, with clear milestones to track progress.
Step 5: Establishing responsible governance
Establishing a governance framework that addresses potential risks such as data privacy, bias, and security is critical. This framework should include:
- Guidelines and standards: Develop comprehensive guidelines for data usage, model training, and output monitoring.
- Oversight mechanisms: Set up oversight committees or designate responsible officers to monitor AI implementations and ensure compliance with ethical standards.
- Continuous monitoring: Implement systems to continually assess the performance and impact of AI applications, ensuring they remain aligned with organizational values and legal requirements.
Step 6: Build AI capabilities and infrastructure
Invest in building the necessary AI capabilities and infrastructure to support your generative AI strategy. This includes hiring or upskilling AI talent, acquiring or developing the appropriate AI tools and platforms, and ensuring sufficient computing resources and data storage capabilities.
Key focus areas should include:
- Technical skills: Ensure your team has the skills to develop, manage, and maintain AI systems.
- Data management: Strengthen your data infrastructure to support the demands of AI, ensuring data quality and accessibility.
- Risk management: Identify potential risks associated with AI projects and develop mitigation strategies.
Step 7: Foster cross-functional collaboration
Encourage cross-functional collaboration and knowledge sharing among different teams and departments within your organization. This will facilitate the integration of generative AI across various business functions and foster a culture of innovation and continuous improvement.
Step 8: Establish performance metrics and monitoring
Define key performance indicators (KPIs) and implement monitoring systems to track the progress and impact of your generative AI initiatives. Regularly evaluate these metrics and adjust your strategy to ensure continuous optimization and alignment with your strategic objectives.
To evaluate the effectiveness of your generative AI strategy, establish clear metrics that can measure success across various dimensions, such as:
- Performance improvement: Assess how AI is improving the efficiency and effectiveness of operations.
- Innovation rate: Monitor the rate of new ideas or products generated through AI.
- ROI: Calculate the return on investment for AI projects to ensure they are delivering value
Step 9: Promote organizational change management
Recognize that adopting generative AI will likely require changes to existing processes, workflows, and organizational structures. Develop a comprehensive change management plan to facilitate a smooth transition and ensure that your workforce is prepared for the transformative impact of this technology.
Step 10: Continuous learning and adaptation
Generative AI is a rapidly evolving field, and your strategy should reflect this dynamic nature. Continuously monitor industry trends, emerging technologies, and best practices. Be prepared to adapt and refine your strategy as new opportunities and challenges arise.
Understanding generative AI strategy framework for organizations
Integrating generative AI into a business strategy effectively demands a comprehensive approach that aligns with the organization’s broader technological and operational frameworks. This section outlines key elements in formulating a business strategy that effectively leverages generative AI capabilities to meet strategic objectives.
Business strategy
The foundation of a robust generative AI strategy begins with clearly defined business objectives and an understanding of how generative AI can meet these goals. Effective use of OKRs (Objectives and Key Results) helps set measurable targets that align with the organization’s vision and operational needs.
Key areas:
- Defining and prioritizing business objectives: Use OKRs to set clear, actionable targets.
- Identifying Gen AI use cases: Map generative AI capabilities to specific business goals for targeted improvements.
- Portfolio management: Balance innovation with financial sustainability to manage GenAI investments effectively.
Technology strategy
Technology strategy involves making informed decisions on the development and deployment of generative AI applications, including whether to build in-house, buy off-the-shelf solutions, or collaborate with external partners.
Key areas:
- To buy or build: Decide between purchasing existing solutions or developing bespoke applications based on cost, customization needs, and strategic importance.
- Infrastructure and MLOps: Establish a robust infrastructure and operational practices to support generative AI’s scalable and efficient use.
- Ensuring security and compliance: Develop secure applications that comply with regulatory standards and ethical guidelines.
Generative AI strategy
A focused generative AI strategy connects business objectives with AI capabilities, ensuring that every initiative delivers tangible results and aligns with broader business goals.
Key areas:
- Mapping use cases to business objectives: Ensure each generative AI project is directly linked to strategic business outcomes.
- Conducting feasibility analysis and pilots: Validate the effectiveness and scalability of GenAI projects through rigorous testing and pilot programs.
- Developing an operating model: Define clear roles, responsibilities, and processes for managing generative AI initiatives.
Organization and people strategy
The success of generative AI also hinges on people and organizational culture. This strategy focuses on securing leadership support, managing change effectively, and developing the necessary skills within the team.
Key areas:
- Leadership and resource allocation: Engage top executives to champion generative AI initiatives and secure necessary resources.
- Change management: Navigate organizational changes with effective communication and support structures.
- Skills development: Identify skill gaps and provide training to prepare the workforce for new technological demands.
Governance strategy
The governance strategy for generative AI ensures that all initiatives align with the organization’s ethical standards, legal requirements, and operational guidelines. Robust governance is critical for maintaining control over AI projects, ensuring they adhere to internal policies and external regulations, and instilling stakeholder trust.
Key areas:
- Developing governance frameworks: Establish clear guidelines and frameworks to oversee the deployment and use of generative AI. This includes setting standards for data usage, model development, and the ethical implications of AI applications.
- Regulatory compliance: Stay updated with changing laws and regulations related to AI and data privacy. Implement compliance mechanisms that are built into the development and deployment processes of AI systems.
- Risk management: Identify potential risks associated with generative AI, including technological, reputational, and operational risks. Develop strategies to mitigate these risks before they impact the business.
- Ethical AI use: Promote the ethical use of AI by incorporating fairness, transparency, and accountability into AI systems. This includes ensuring AI decisions are explainable and non-discriminatory.
Best practices for a robust generative AI strategy
Implementing generative AI requires careful planning and alignment with your business’s unique needs and culture. Here are tailored best practices to ensure your strategy maximizes the technology’s potential and integrates seamlessly with your operational goals.
Leverage generative AI as an augmentative tool
It’s crucial to understand that generative AI is designed to enhance, not replace human capabilities. Automating repetitive tasks frees up employees to focus on higher-value work that requires human attributes such as emotional intelligence, creative thinking, and strategic insight. Emphasize that generative AI is a partner in productivity, helping employees achieve more and innovate faster.
Foster an AI-receptive organizational culture
Adopting generative AI hinges on an organizational culture that embraces continuous improvement and technological innovation. Promote a culture of adaptability where employees are encouraged to experiment with AI tools and contribute ideas on how these technologies can be best utilized. A proactive, curious, and flexible mindset should be fostered at all levels, especially from leadership, to ensure broad acceptance and enthusiasm for new AI-driven processes.
Invest in targeted skills development
Investing in the right skills is essential as AI reshapes roles and tasks. Focus on developing AI literacy across the organization while also enhancing skills that AI complements, such as analytical thinking, decision-making, and creative problem-solving. Moreover, training programs focusing on the ethical use and management of AI technologies will ensure that your team can responsibly implement and oversee AI applications.
Make strategic decisions on building vs. buying AI capabilities
Evaluate the benefits of purchasing existing AI solutions against the potential advantages of custom-developed technologies. This decision should be based on cost, integration ease, and specific business requirements.
Consider strategic AI leadership roles
Depending on the scale and scope of your AI initiatives, appointing a dedicated leadership role, such as a Chief AI Officer (CAIO), can provide clear direction and oversight for AI deployments. This role can facilitate the integration of AI into strategic planning, ensuring that AI initiatives align with broader business objectives and are implemented with ethical considerations at the forefront.
Strategically assess and manage data resources
Data is the foundation of any effective AI system. Develop a comprehensive data strategy that ensures data quality, accessibility, and security. This strategy should include mechanisms for integrating real-time data to enhance responsiveness and agility in decision-making. Also, balance the use of internal data with external data sources to broaden insights and improve AI model robustness.
Integrate AI into business strategy
Review and adjust your business strategy to account for the opportunities and challenges presented by generative AI. This may involve rethinking business models, product lines, or customer engagement strategies to fully leverage AI capabilities. Strategic integration ensures that AI initiatives are purpose-driven and closely tied to achieving competitive advantage.
Establish a scalable technological infrastructure
Prepare your technological infrastructure to support scalable AI deployments. This includes robust data storage and processing capabilities and advanced cybersecurity measures to protect sensitive data and AI models from potential threats. Ensure your infrastructure is flexible enough to adapt as AI technologies and business needs evolve.
Encourage continuous learning and adaptation
Maintain a dynamic learning environment that encourages continuous professional development related to AI. Staying abreast of new AI trends, technologies, and methodologies will help your organization adapt and respond to changes effectively.
- Manage expectations: Educate and set realistic expectations across the organization about what AI can and cannot do to prevent disillusionment and resistance.
- Cultural adaptation: Consider the impact of AI on the organizational culture and actively manage the transition to ensure that employees feel supported and valued.
Strategic monitoring and evaluation
- Continual learning: Keep track of AI and generative AI developments to continuously refine and enhance your strategy.
- Feedback mechanisms: Implement effective feedback mechanisms to learn from successes and failures in AI projects, facilitating continuous improvement.
Plan for future expansion and scalability
Design your generative AI initiatives with future growth in mind. This includes planning for potential expansions in AI applications and ensuring that your systems can handle increased loads and complexity. Scalability planning helps avoid bottlenecks and ensures your AI systems can grow in line with your business.
By implementing these best practices, organizations can create a generative AI strategy that not only addresses immediate operational needs but also positions them for long-term success and innovation in an increasingly AI-driven world.
Business challenges in implementing generative AI strategy
While generative AI offers immense potential, organizations may face several challenges during implementation and adoption. Some of the key business challenges include:
- Data availability and quality: Generative AI models require vast amounts of diverse, high-quality data for training. Acquiring, curating, and preparing suitable data can be a significant challenge, especially in domains with limited data availability or strict privacy and compliance regulations. Issues like data privacy, ethical considerations in data sourcing and the domain-specific data requirement further complicate this scenario. Without the right data, training generative AI models or building relevant generative AI use cases is challenging.
- Computational resources: Training and deploying large generative AI models can be computationally intensive, demanding significant hardware resources such as GPUs, TPUs, or specialized accelerators. Acquiring and maintaining this infrastructure can be costly, particularly for small and medium-sized organizations.
- Ethical and regulatory concerns: Generative AI raises various ethical concerns, including potential biases, disinformation, privacy violations, and misuse for malicious purposes. Navigating these concerns and adhering to evolving regulations and guidelines can be challenging, requiring robust governance frameworks and risk mitigation strategies.
- Scalability and sustainability: Scaling AI solutions from pilot projects to full-scale deployment across an organization can be fraught with difficulties, including inconsistent standards, varying degrees of adoption among teams, and integration issues.
- Talent acquisition and upskilling: Generative AI is rapidly evolving, and there is a shortage of skilled professionals with expertise in developing, deploying, and maintaining these models. Attracting and retaining top talent or upskilling existing teams can be a significant hurdle for many organizations.
- Integration and deployment complexities: Integrating generative AI models into existing systems, applications, and workflows can be complex, requiring careful planning, testing, and adaptation. Deployment considerations, such as scalability, security, and user experience, must be addressed to ensure successful adoption.
- Interpretability and explainability: Many generative AI models, particularly deep learning models, can be perceived as “black boxes,” making it challenging to understand their decision-making processes and ensure transparency and accountability. Achieving interpretability and explainability is crucial for building trust and meeting regulatory requirements.
- Continuous monitoring and adaptation: Generative AI models can exhibit performance drift or biases over time due to changes in data distributions or external factors. Implementing robust monitoring systems and model retraining or updating processes is essential to maintain model accuracy and reliability.
- Organizational alignment and change management: Adopting generative AI requires significant organizational changes and shifts in processes, culture, and mindsets. Organizational culture and employee perceptions about AI can significantly impact the adoption and success of AI initiatives. Resistance to change, fear of job displacement, and lack of understanding about AI’s benefits are common. Aligning stakeholders, managing change effectively, and fostering a data-driven culture can be demanding for organizations.
- ROI and value realization: While generative AI promises significant benefits, quantifying and demonstrating its tangible business value can be challenging, especially in the early stages of adoption. Establishing clear metrics and measuring ROI is crucial for justifying investments and securing buy-in from stakeholders.
Overcoming these challenges requires a strategic approach involving cross-functional collaboration, robust governance frameworks, and a commitment to continuous learning and adaptation. Organizations that successfully navigate these obstacles will be well-positioned to harness the transformative potential of Generative AI.
A strategic checklist for enterprise generative AI strategy and implementation
- Align objectives and prioritize use cases
- Identify key business objectives and prioritize Generative AI use cases based on potential impact and ROI.
- Involve stakeholders: Executives, business analysts, and product managers.
- Evaluate model options and fit
- Assess the trade-offs between building in-house, buying a ready-to-use solution, or adapting a pre-trained model.
- Consider factors like customization needs, resources, and expertise.
- Involve stakeholders: Data scientists, technical architects, and procurement teams.
- Assess technical compatibility and vendor credibility
- Ensure the chosen generative AI model and solution support the required algorithms and use cases.
- Evaluate vendor track record, reputation, and customer success stories.
- Involve stakeholders: Technical leads, legal teams, and procurement teams.
- Plan for seamless integration and infrastructure
- Assess the ease of integrating the generative AI solution into existing systems and workflows.
- Identify and provision the necessary hardware, software, and infrastructure resources.
- Involve stakeholders: IT teams, system administrators, and technical architects.
- Identify capabilities, limitations, and knowledge gaps
- Evaluate the model’s capabilities for tasks like data generation, content creation, and simulations.
- Recognize and document the solution’s limitations and potential knowledge gaps.
- Involve stakeholders: Data engineers, content teams, department heads, and compliance officers.
- Develop a comprehensive skill development plan
- Appoint internal champions and advocates to facilitate organizational buy-in and adoption.
- Create a detailed onboarding and training plan for upskilling teams.
- Involve stakeholders: HR managers, training teams, and project managers.
- Establish a risk-aware implementation strategy
- Estimate the time required for successful implementation and full adoption.
- Identify and mitigate potential risks like data security, algorithmic biases, and compliance issues.
- Involve stakeholders: Project managers, security teams, compliance officers, and legal teams.
- Ensure compliance, safety, and clear communication
- Establish guidelines and protocols to meet all relevant safety and compliance standards.
- Implement transparent communication channels for stakeholder engagement and change management.
- Involve stakeholders: Compliance officers, legal teams, and internal communications teams.
- Prioritize adaptability, scalability, and future-proofing
- Evaluate the generative AI solution’s ability to adapt to evolving needs and technological advancements.
- Assess the solution’s scalability to grow with the organization’s future requirements.
- Involve stakeholders: Technical leads, CTO, IT managers, and executives.
- Plan for rollout, feedback, and ongoing support
- Develop a phased or full-scale rollout strategy based on organizational readiness.
- Implement mechanisms for user feedback, continuous improvement, and ongoing support.
- Involve stakeholders: Project managers, department heads, customer support teams, and UX designers.
This strategic checklist provides a comprehensive framework for organizations to navigate the complexities of generative AI implementation, ensuring alignment with business objectives, technical compatibility, risk management, and long-term adaptability.
How does LeewayHertz help devise generative AI strategies for enterprises and startups?
As a leading AI consultancy organization, LeewayHertz offers a comprehensive suite of services to help enterprises unlock the transformative potential of generative AI (GenAI) technologies. Our end-to-end solutions encompass strategy, development, deployment, and ongoing support, ensuring seamless integration and maximum value realization. Our holistic approach ensures that organizations can harness the full potential of this transformative technology while mitigating risks and aligning with their strategic objectives.
- Generative AI consulting: Our consultants work closely with your team to develop a customized GenAI adoption strategy aligned with your business objectives. We leverage deep technical expertise in generative models and technologies to recommend suitable solutions for your specific use cases.
- Technology evaluation and selection: LeewayHertz maintains a deep understanding of the latest generative AI models, frameworks, and platforms available in the market. We evaluate the strengths, limitations, and suitability of different GenAI solutions based on your specific requirements, ensuring optimal fit and performance. Our vendor-agnostic approach ensures unbiased recommendations, enabling you to select the most appropriate technology stack.
- Domain-specific generative AI model development: We build domain-specific GenAI models to harness the full potential of AI in a way that is relevant and impactful for your business. Our process includes a comprehensive assessment, foundational model selection, fine-tuning with proprietary data, and rigorous testing to meet your requirements.
- Generative AI solutions development: LeewayHertz develops innovative GenAI solutions, including chatbots, AI copilots, recommendation systems, and predictive analytics tools. We leverage state-of-the-art foundational models like GPT, Llama, PaLM, Gemini, Mistral, and Claude as building blocks for intelligent, creative, and adaptable systems.
- Generative AI integration: We seamlessly integrate GenAI solutions into your existing tech infrastructure, streamlining internal workflows and customer-facing systems. Our integration process ensures minimal disruption to ongoing operations, enabling a smooth transition to AI-powered processes.
- Upgrade and maintenance: LeewayHertz provides post-deployment maintenance and upgrade services to keep your GenAI solutions operating smoothly and delivering consistent value. Regular maintenance and timely upgrades ensure your solutions remain competitive and aligned with your evolving business needs.
- End-to-end project lifecycle support: Our services cover the entire project lifecycle, from requirement assessment and strategy building to design, development, testing, deployment, and ongoing support. We follow a rigorous process to ensure the GenAI solution meets stakeholder expectations and delivers high-quality outputs.
By partnering with LeewayHertz, enterprises gain access to a comprehensive range of services tailored to their generative AI adoption journey. Our expertise, coupled with a deep understanding of industry-specific challenges, enables us to deliver innovative and impactful solutions that drive business growth and competitive advantage.
Endnote
As we conclude our exploration of creating and implementing a generative AI strategy, it’s clear that this technology is not just a transformative force but a strategic imperative for organizations aiming to thrive in an increasingly digital landscape. Generative AI offers unparalleled opportunities for innovation and efficiency, but its successful deployment hinges on thoughtful planning, ethical considerations, and strategic alignment with broader business goals.
Organizations that approach generative AI with a holistic, integrated strategy—considering both the technological and human aspects—will be best positioned to harness its benefits. By fostering a culture of continual learning, experimentation, and adaptability, businesses can not only anticipate but also shape the future of their industries.
The journey towards effective AI implementation is ongoing. It requires vigilance, flexibility, and a commitment to ethical practices to ensure that as technologies evolve, they do so in a manner that benefits all. In this dynamic environment, the ultimate competitive advantage will belong to those who view generative AI not just as a series of tools but as a core component of their strategic vision.
The journey through the AI-driven transformation landscape is complex yet filled with substantial opportunities for those equipped to meet its challenges with strategic foresight and innovative thinking. The road ahead is as promising as it is challenging, and the right strategy will ensure that your organization not only keeps pace but sets the pace in the transformative age of generative AI.
Ready to transform your business with generative AI? Explore LeewayHertz’s generative AI development services and take the first step toward innovative solutions tailored to your needs. Let’s innovate together!
Start a conversation by filling the form
All information will be kept confidential.
Insights
GenAI in marketing: Unleashing unprecedented creativity and precision
From enhancing content creation and SEO optimization to driving customer engagement and reshaping market research, generative AI empowers marketers to leverage data-driven insights and automation for more effective and personalized campaigns.
AI in gaming: Transforming gaming worlds with intelligent innovations
Artificial Intelligence finds extensive applications in the gaming industry, transforming virtually every aspect of game development and player experiences.
AI in portfolio management: Use cases, applications, benefits and development
AI is reshaping portfolio management by offering powerful tools that enhance investment strategies and decision-making.