AI in knowledge management: Paving the way for transformative insights
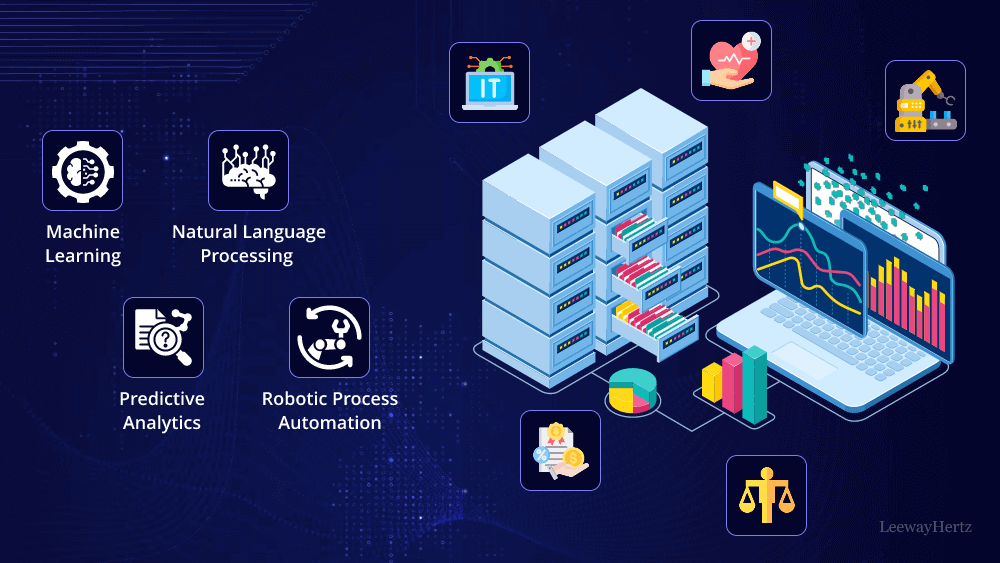
Knowledge management is crucial for operational excellence in most companies. The foundational essence of any successful enterprise lies in its ability to harness, organize, and seamlessly access the wealth of information at its disposal, making knowledge management not just a necessity but a linchpin for success.
Yet, as companies grapple with the deluge of information, the challenge intensifies. The sheer volume of data, coupled with the perpetual need to keep knowledge organized and accessible, forms a daunting hurdle. It’s not merely about managing data; it’s about navigating the web of information to glean insights, make informed decisions, and maintain a competitive edge in the ever-evolving business landscape.
The integration of Artificial Intelligence (AI) stands as a game-changer. AI-powered knowledge management systems offer a sophisticated approach to capturing, organizing, and leveraging information effectively. By harnessing advanced AI technologies such as machine learning, generative AI, neural networks, natural language processing, etc., these systems automate the management of vast amounts of data and information. This not only streamlines the process but also enhances accuracy and efficiency.
AI-powered knowledge management systems excel in their ability to sift through massive volumes of data, identify patterns, and learn from user interactions. By doing so, they provide valuable insights that humans might overlook, ultimately driving better decision-making and organizational success.
This article explores the intricacies of knowledge management, encompassing its definition and types. It emphasizes the significance of AI in knowledge management, explores applications and technologies, outlines steps for implementation, and offers insights into future trends in AI for knowledge management.
- What is knowledge management?
- Types of knowledge management
- Understanding AI’s role in the knowledge management process
- Applications of AI in knowledge management
- Information retrieval
- Automated content curation
- Knowledge discovery and insights generation
- Automated tagging and classification
- Automated knowledge base maintenance
- Intelligent search
- Personalized user experiences
- Content gap analysis
- Automated question answering
- Semantic analysis and tagging
- Ethical and compliance monitoring
- Steps to implement an AI-based knowledge management system
- How LeewayHertz’s generative AI platform transform knowledge management processes?
- How AI-powered knowledge management systems transform organizations?
- The benefits of AI in knowledge management
- AI technologies used in knowledge management
- Future trends in AI for knowledge management
What is knowledge management?
Knowledge management (KM) is a systematic and strategic approach to acquiring, organizing, storing, and sharing an organization’s intellectual assets to enhance efficiency, innovation, and decision-making. It encompasses recognizing, capturing, and leveraging both implicit and explicit knowledge within an organization, aiming to establish a competitive edge and nurture a culture of ongoing learning.
Knowledge management encompasses the following:
- Knowledge identification : KM begins with identifying relevant knowledge assets within an organization. This includes explicit knowledge, which is documented and codified, as well as tacit knowledge, which resides in the minds of individuals and may be more challenging to articulate. The goal is to recognize and leverage the diverse forms of knowledge that contribute to organizational success.
- Knowledge capture : Once identified, knowledge needs to be captured and documented. This process involves creating databases, repositories, and systems to store explicit knowledge, such as documents, manuals, and relational databases. Capturing tacit knowledge often involves facilitating interactions, discussions, and collaborative platforms that allow individuals to share their expertise and experiences.
- Knowledge organization : Effective knowledge management involves organizing information in a structured manner. Taxonomies, ontologies, and knowledge maps help categorize and connect different pieces of information, making it easier for individuals to access relevant knowledge when needed. This organization enhances the discoverability and usability of knowledge assets.
- Knowledge storage and retrieval : Knowledge is stored in various formats, including documents, databases, and multimedia. An essential aspect of KM is establishing systems that enable efficient retrieval of information. This may involve the implementation of search engines, content management systems, and other tools that facilitate quick and accurate access to knowledge resources.
- Knowledge sharing and collaboration : KM underscores the significance of cultivating a culture where knowledge sharing and collaboration thrive within an organization. This involves fostering an environment where employees are encouraged to share their expertise, experiences, and insights. Collaboration platforms, forums, and communication tools play a crucial role in facilitating the exchange of knowledge among team members.
- Knowledge application : The ultimate goal of KM is to apply knowledge strategically to achieve organizational objectives. This involves using knowledge to solve problems, make informed decisions, innovate, and adapt to changes in the business environment. KM ensures that knowledge becomes a valuable resource that contributes directly to the success and competitiveness of the organization.
- Continuous learning and improvement : KM is a dynamic process that recognizes the evolving nature of knowledge. Organizations engaged in knowledge management foster a culture of continuous learning and improvement. This involves regularly updating knowledge repositories, incorporating feedback, and adapting knowledge management strategies to align with changing organizational needs and goals.
Knowledge management is a holistic and proactive approach that recognizes the importance of leveraging intellectual assets to enhance organizational performance. By systematically managing knowledge throughout its lifecycle, from identification to application, organizations can gain a competitive edge, promote innovation, and foster a resilient and adaptive workplace culture.
Types of knowledge management
Knowledge management encompasses various approaches and strategies tailored to suit different organizational needs. Understanding the diverse types of knowledge management is essential for selecting the most suitable model. Here are some prominent types:
Knowledge management encompasses various approaches and strategies tailored to suit different organizational needs. Understanding the diverse types of knowledge management is essential for selecting the most suitable model. Here are some prominent types:
Explicit knowledge management
Explicit knowledge refers to information easily documented and shared systematically, typically in written form. When effectively managed, explicit knowledge can enhance decision-making, streamline processes, and improve overall performance within a company.
This explicit knowledge is typically stored in a knowledge base and consists of formalized documentation for tasks, decision-making, or audience education.
Examples of explicit knowledge include:
- FAQs (Frequently Asked Questions): These documents answer common inquiries, helping users quickly find solutions to their queries.
- Instructions: Step-by-step guides or manuals outline procedures and processes, facilitating task completion.
- Raw data and related reports: Data sets and analytical reports offer insights into trends, patterns, and performance metrics, aiding in informed decision-making.
- Diagrams: Visual representations such as flowcharts or organizational charts convey complex information clearly and concisely.
- One-sheets: Concise documents summarizing key information or concepts, often used for reference or informational purposes.
- Strategy slide decks: Presentations outlining strategic initiatives, goals, and plans provide stakeholders with a comprehensive overview of organizational strategies.
By maintaining well-documented explicit knowledge within their knowledge base, companies can leverage these resources to enhance efficiency, effectiveness, and knowledge dissemination across the organization.
AI aids in managing explicit knowledge by automating organization and retrieval processes within knowledge bases. AI enhances search capabilities through natural language processing and machine learning algorithms, allowing faster access to relevant information. Additionally, AI can assist in content creation and curation by identifying patterns and suggesting updates, ultimately improving the effectiveness of explicit knowledge utilization.
Implicit/tacit knowledge management
Implicit or tacit knowledge refers to learned skills or know-how gained through applying explicit knowledge to specific situations. It involves synthesizing learned information with experience to solve new problems or perform tasks efficiently.
Implicit knowledge has traditionally been challenging to document and capture in formal knowledge bases due to its experiential nature. However, AI technologies can be crucial in managing implicit knowledge by facilitating its documentation and dissemination. Here’s how:
- AI-powered NLP algorithms can analyze unstructured data such as text documents, emails, and chat transcripts to identify implicit knowledge embedded within them. By recognizing patterns and interpreting contextual cues, AI possesses the capacity to extract tacit knowledge from various sources and make it accessible to users.
- ML algorithms can learn from past interactions and experiences to infer implicit knowledge. By analyzing user behaviors, preferences, and decision-making processes, AI can identify implicit knowledge patterns and suggest solutions or best practices tailored to specific scenarios.
- AI possesses the capability to sift through extensive datasets, uncovering implicit knowledge concealed within organizational repositories, collaboration platforms, and communication channels. AI automatically identifies relevant insights and expertise, enabling organizations to leverage implicit knowledge more effectively.
One example of documented implicit knowledge is an employee’s ability to prioritize tasks and manage multiple projects effectively to meet deadlines. AI-driven knowledge management systems can capture this implicit knowledge through employee performance data, project management tools, and communication platforms.
By leveraging AI technologies, organizations can bridge the gap between explicit and implicit knowledge, enabling more comprehensive knowledge management strategies. AI facilitates the sharing of tacit knowledge through collaboration tools, forums, and platforms that encourage interaction and knowledge exchange among team members. Implicit knowledge, acquired through real-life experiences, further enriches teams, especially in aiding new employee onboarding.
Declarative knowledge management
Declarative or propositional knowledge refers to static information and facts specific to a given topic, easily accessible and retrievable. It represents a conscious awareness of an individual’s understanding of a subject matter.
This form of knowledge is typically stored in documentation or databases, emphasizing the ‘who,’ ‘what,’ ‘where,’ and ‘when’ aspects of information rather than the ‘how’ or ‘why.’ When documented, it forms the foundation for understanding the subject matter and aids companies in sharing procedural and explicit knowledge more effectively.
AI technologies can manage declarative knowledge by automating and enhancing processes related to its storage, retrieval, and utilization:
- AI-powered NLP algorithms can analyze and extract relevant information from unstructured data sources like documents, emails, and reports. This enables organizations to more efficiently identify and capture declarative knowledge embedded within textual content.
- AI-driven semantic search algorithms enhance the accuracy and relevance of search results by understanding the context and meaning behind queries. This allows users to access declarative knowledge more effectively, even when using natural language queries or ambiguous terms.
- AI-driven knowledge graph technology organizes declarative knowledge into interconnected data structures, facilitating intuitive navigation and exploration of related concepts. Knowledge graphs enable users to discover new insights and connections within declarative knowledge repositories by representing relationships between entities, attributes, and events.
- AI algorithms can automatically curate and categorize declarative knowledge based on predefined criteria, such as relevance, importance, or user preferences. This streamlines organizing and presenting declarative knowledge to users, ensuring that relevant information is readily accessible.
Some examples of declarative knowledge include:
- Knowing the company goals for the year, as communicated through official documents or announcements.
- Based on information shared in company newsletters or internal communications, understanding on what basis performance will be measured.
By leveraging AI technologies, organizations can enhance the management and utilization of declarative knowledge, enabling more efficient decision-making, knowledge sharing, and organizational learning.
Procedural knowledge management
Procedural knowledge delves into the ‘how’ behind the operation of things and is demonstrated through one’s ability to perform tasks or actions. Unlike declarative knowledge, which focuses on the ‘who, what, where, or when,’ procedural knowledge is less articulated and is often demonstrated through action or documented in manuals.
AI technologies play a vital role in managing procedural knowledge by facilitating its documentation, dissemination, and execution:
- AI-driven workflow automation tools streamline procedural knowledge execution by automating repetitive tasks and standardizing processes. By analyzing patterns in task execution, AI can identify optimization and efficiency improvement opportunities.
- AI-driven process mining techniques analyze digital traces of operational activities to uncover procedural knowledge embedded within organizational systems and workflows. By visualizing process flows and identifying bottlenecks or inefficiencies, AI assists organizations in optimizing procedural knowledge for enhanced performance.
- AI algorithms can dynamically update procedural knowledge documentation based on real-time data and user feedback. This ensures that procedural knowledge remains current and relevant, reflecting changes in organizational processes or best practices.
Examples of procedural knowledge include:
- Standard Operating Procedures (SOPs) outline step-by-step instructions for performing specific tasks or using equipment in an organization.
- Training manuals guide how to execute complex procedures or workflows effectively.
By leveraging AI technologies, organizations can enhance the management and utilization of procedural knowledge, enabling more efficient task execution, training, and process optimization.
By exploring these types of knowledge management, organizations can tailor their approaches to align with specific objectives, fostering a more efficient and effective knowledge-sharing environment.
Launch your project with LeewayHertz!
Streamline and simplify knowledge management with AI! Organize data, extract insights, and empower decision-making with LeewayHertz’s custom AI solutions tailored to your business.
Understanding AI’s role in the knowledge management process
Integrating AI in knowledge management processes transforms how organizations create, store, share, and apply knowledge. Here’s how AI enhances each aspect of the knowledge management process:
- Knowledge creation: AI systems facilitate predictive analytics by autonomously analyzing vast amounts of data to identify patterns and trends. They can uncover previously unknown insights and generate new knowledge. For example, AI can forecast sales probabilities by analyzing customer data and market trends or identify organizational inefficiencies by analyzing CRM records.
- Knowledge storing and retrieving: AI-powered systems excel at collecting, classifying, organizing, storing, and retrieving explicit knowledge. They can quickly analyze and filter various content channels to provide relevant information, enabling efficient knowledge reuse by teams and individuals. For instance, AI can organize and summarize legal precedents relevant to a new case or retrieve dispersed information for troubleshooting situations.
- Knowledge sharing: AI fosters knowledge sharing by connecting individuals with relevant expertise and facilitating collaborative intelligence. It helps create a comprehensive perspective on knowledge sources and promotes coordination across organizational silos. For example, AI can facilitate feedback and peer review on communication platforms or enable real-time sharing between marketing channels and sales pipelines.
- Knowledge application: AI enhances the application of knowledge by offering intuitive interfaces and facilitating access to relevant information. Voice-based assistants and smart search functionalities make it easier for users to find and utilize knowledge effectively. Additionally, AI promotes equitable access to knowledge by removing barriers like social costs or fear of reprisal. For instance, AI-powered systems can search and prepare knowledge sources to support decision-making processes or provide real-time assistance in performing tasks.
AI transforms knowledge management by leveraging advanced analytics, NLP, and machine learning to enhance knowledge creation, storage, sharing, and application across organizations.
Applications of AI in knowledge management
AI has a transformative impact on knowledge management, offering various applications that enhance efficiency, decision-making, and collaboration within organizations. Here are several key applications of AI in knowledge management:
Intelligent search and information retrieval
Information retrieval represents a pivotal application of AI in knowledge management. By harnessing NLP and machine learning algorithms, AI-powered search engines significantly elevate the accuracy and relevance of search results. This transformative capability enables users to swiftly and efficiently retrieve the most pertinent information from extensive knowledge repositories. The utilization of NLP ensures that the search process becomes more context-aware and aligned with the user’s intent, thereby enhancing the overall effectiveness of information retrieval within organizational databases.
Automated content curation
Automated content curation, a fundamental application of AI in knowledge management, redefines how organizations deliver information. By leveraging advanced algorithms, AI automates the curation process by analyzing user preferences, behaviors, and historical data. This analytical approach enables the system to generate personalized content recommendations, ensuring that users receive information that aligns precisely with their individual needs and interests. This personalized content delivery not only enhances user engagement but also streamlines the consumption of relevant information, contributing to a more efficient and tailored knowledge-sharing experience within the organization.
Knowledge discovery and insights generation
Knowledge discovery and insights generation are transformative applications of AI in knowledge management, unlocking valuable perspectives within vast datasets. AI, through machine learning algorithms, facilitates the identification of hidden patterns, trends, correlations, and anomalies. This capability empowers organizations to extract meaningful insights, fostering continuous learning and informed decision-making. By navigating through extensive datasets, AI enhances the analytical capacity of knowledge management systems, allowing organizations to stay ahead of industry trends and make strategic decisions based on a thorough comprehension of their data landscape.
Automated tagging and classification
Automated tagging and classification, a core application of AI in knowledge management, streamlines the organization of information within repositories. Through sophisticated algorithms, AI automates the tagging and categorization of documents, ensuring that each piece of content is appropriately labeled. This automation not only expedites the organization process but also enhances the efficiency of information retrieval. By assigning accurate labels, AI ensures that users can effortlessly navigate knowledge repositories, retrieving precisely the information they seek. This application significantly contributes to the overall accessibility, organization, and usability of vast knowledge databases within an organization.
Automated knowledge base maintenance
Automated knowledge base maintenance emerges as a pivotal application of AI in knowledge management, reshaping the upkeep of organizational knowledge repositories. Leveraging advanced algorithms, AI automates the identification of outdated or redundant information within knowledge bases. By suggesting timely updates, AI ensures that these repositories evolve dynamically, staying accurate, relevant, and aligned with the ever-changing needs of the organization. This proactive maintenance not only enhances the quality of information but also contributes to the overall effectiveness and adaptability of knowledge management systems, reflecting a continuous commitment to precision and relevance in the organizational knowledge landscape.
These applications collectively demonstrate the diverse ways AI is redefining knowledge management, making information more accessible, actionable, and valuable for organizations.
Intelligent search
By merging semantic comprehension with conventional search algorithms, AI can decipher the context behind user queries, ensuring that search results correspond to user intent rather than relying solely on literal keyword matches. This advancement enables employees to access precise and contextually relevant data, even when using vague or commonly used terms in their searches. As a result, knowledge retrieval becomes more efficient and accurate, enhancing overall productivity and decision-making within the organization.
Personalized user experiences
AI-driven knowledge management systems personalize user experiences by recommending content tailored to each individual’s role, past interactions, and specific queries. By providing relevant information proactively, these systems enhance efficiency and engagement, allowing users to access the most pertinent knowledge quickly and easily. This personalized approach fosters a more productive and satisfying user experience, ultimately driving better outcomes for the organization.
Content gap analysis
AI analyzes user queries that produce no or low-quality results within existing knowledge bases, signaling potential gaps in information coverage. By identifying these gaps, AI prompts organizations to create new content or update existing resources to address user needs comprehensively. This proactive approach ensures that knowledge bases remain robust and relevant, improving user satisfaction and productivity.
Automated question answering
AI-powered chatbots and virtual assistants offer immediate responses to frequently asked questions, minimizing the reliance on manual search and accelerating productivity. By leveraging NLP capabilities, these intelligent systems can understand and address user inquiries swiftly and accurately. This streamlined communication process enhances efficiency and the overall user experience within knowledge management systems.
Semantic analysis and tagging
AI utilizes advanced semantic analysis techniques to thoroughly analyze textual content, allowing for precise and contextually relevant information tagging. This enhances the accuracy and relevance of information retrieval within knowledge management systems. By understanding the nuanced meaning and relationships within text, AI enables more effective categorization and organization of content, improving user experiences and decision-making processes.
Ethical and compliance monitoring
AI is crucial in monitoring knowledge management processes and ensuring compliance with ethical standards and regulatory requirements. By continuously analyzing data and identifying potential breaches or inconsistencies, AI can flag issues for review before they escalate. This proactive approach helps organizations maintain integrity and avoid legal consequences associated with non-compliance. Additionally, AI-powered monitoring enhances transparency and accountability within knowledge management systems.
These applications collectively demonstrate the diverse ways AI is redefining knowledge management, making information more accessible, actionable, and valuable for organizations.
Steps to implement an AI-based knowledge management system
Implementing an AI-based knowledge management system involves a strategic and systematic approach. Here are the key steps to the implementation process:
- Define objectives and goals: Clearly outline the objectives and goals of implementing an AI-based knowledge management system. Identify specific challenges or areas where AI can add value, such as improving information retrieval, enhancing decision-making, or automating routine tasks.
- Assess current knowledge management practices: Conduct a thorough assessment of existing knowledge management processes, technologies, and content. Identify strengths, weaknesses, and areas for improvement. Understand how AI can complement or enhance the current system.
- Select appropriate AI technologies: Determine which AI technologies align with your goals. Consider technologies such as Natural Language Processing (NLP), Machine Learning (ML), Cognitive Computing, or Robotic Process Automation (RPA) based on the specific needs of your knowledge management system.
- Data preparation and integration: Ensure that your data is clean, structured, and ready for AI analysis. Integrate AI technologies seamlessly with existing databases and knowledge repositories. Address data privacy and security considerations.
- Choose a knowledge management platform: Select a knowledge management platform that supports AI integration. The platform should provide features for content organization, collaboration, and user-friendly interfaces. Ensure that it aligns with your organization’s size, structure, and requirements.
- Implement AI algorithms: Work with AI experts or data scientists to implement algorithms tailored to your knowledge management objectives. This may include developing recommendation engines, search algorithms, or automation scripts based on your specific needs.
- User training and change management: Train users on the new AI-based knowledge management system. Provide guidance on how to interact with AI features, leverage automation, and make the most of the enhanced functionalities. Implement change management strategies to facilitate a smooth transition.
- Pilot testing: Conduct a pilot test with a smaller group of users to gather feedback, identify potential issues, and fine-tune the system. Use this phase to validate the effectiveness of AI features and make necessary adjustments.
- Scale implementation: Once the pilot is successful, scale the implementation to the entire organization. Monitor system performance, user satisfaction, and the impact on knowledge management processes.
- Continuous improvement: Establish a feedback loop for continuous improvement. Regularly assess the performance of the AI-based knowledge management system, gather user feedback, and implement updates or enhancements as needed. Stay informed about advancements in AI technology for potential future improvements.
- Data governance and compliance: Implement robust data governance policies to ensure data quality, integrity, and compliance with regulations. Establish protocols for data updates, security measures, and access control to maintain the integrity of the knowledge management system.
By following these steps, organizations can effectively implement an AI-based knowledge management system that aligns with their goals, enhances efficiency, and fosters a culture of continuous learning and innovation.
Launch your project with LeewayHertz!
Streamline and simplify knowledge management with AI! Organize data, extract insights, and empower decision-making with LeewayHertz’s custom AI solutions tailored to your business.
How LeewayHertz’s generative AI platform transform knowledge management processes?
LeewayHertz’s generative AI platform, ZBrain, is a vital tool helping enhance and streamline various aspects of knowledge management within businesses across industries. By creating custom LLM-based applications tailored to clients’ proprietary data, ZBrain optimizes knowledge management workflows, ensuring operational efficiency and enriched organizational knowledge. The platform processes diverse data types, including text, images, and documents, and utilizes advanced language models like GPT-4, Vicuna, Llama 2, and GPT-NeoX to build context-aware applications that can improve decision-making, deepen insights, and boost productivity, all while maintaining strict data privacy standards, making it indispensable for modern knowledge management operations.
Challenges like information overload, disparate data sources, and the need for real-time knowledge sharing are prevalent in knowledge management. ZBrain effectively addresses these challenges through its distinctive feature called “Flow,” which provides an intuitive interface that allows users to create intricate business logic for their apps without the need for coding. Flow’s easy-to-use drag-and-drop interface enables the seamless integration of prompt templates, large language models, and other generative AI models into your app’s logic for its easy conceptualization, creation, or modification.
ZBrain builds sophisticated apps capable of converting complex knowledge data into actionable insights, enhancing operational efficiency, minimizing errors, and improving the overall knowledge-sharing experience.
For a comprehensive exploration of ZBrain’s capabilities, delve into this resource that outlines a variety of industry-specific Flow processes. This compilation underscores the platform’s resilience and adaptability, showcasing how ZBrain efficiently tackles various use cases across different industries.
How AI-powered knowledge management systems transform organizations?
Knowledge management has been a cornerstone of organizational success, encompassing the systematic process of capturing, organizing, and utilizing information for informed decision-making. In recent times, the integration of AI has redefined the landscape of knowledge management, ushering in a new era of efficiency and innovation.
- Integrating AI with knowledge management
AI redefines knowledge management, enhancing efficiency and innovation. The integration introduces adaptability and autonomy in managing organizational knowledge.
- Continuous learning and adaptation
AI systems learn from data patterns and user interactions, continuously refining their understanding. This adaptability is vital in the fast-paced, ever-changing business environment.
- Handling complex information
AI-powered systems adeptly manage the growing volume and complexity of information. They offer intelligent and proactive management solutions for diverse data types.
- Predictive analytics and forecasting
AI algorithms analyze historical data to forecast future trends and insights. This capability aids in anticipating organizational needs and supports strategic decision-making.
- Smart knowledge repositories
AI facilitates the creation of evolving knowledge repositories. These repositories ensure information stays relevant and aligns with changing organizational goals.
- Transformative impact on knowledge management
AI elevates knowledge management into a dynamic, interactive system. It ensures the system is forward-thinking, self-optimizing, and continually adds value to the organization.
The benefits of AI in knowledge management
The integration of AI in knowledge management yields numerous benefits for organizations. Here are the key advantages that AI brings to knowledge management:
- Efficient information retrieval: AI-powered search algorithms enhance information retrieval by providing more accurate and relevant results. Natural Language Processing (NLP) enables users to pose queries in a more conversational manner, improving the efficiency of finding specific information within vast datasets.
- Decision support and insights: AI enhances decision-making processes by providing valuable insights derived from data analysis. Machine learning (ML) algorithms play a pivotal role in discerning patterns and trends within datasets, empowering organizations to make informed and data-driven decisions.
- Adaptive learning and improvement: AI systems continually learn from user interactions, feedback, and changes in data patterns. This adaptive learning capability ensures that knowledge management systems evolve, becoming more accurate, relevant, and aligned with organizational goals.
- Workflow integration and automation: AI seamlessly integrates into existing workflows, automating routine tasks and streamlining knowledge management processes. This integration enhances user adoption, as employees can incorporate AI-driven tools without significant disruptions to their daily routines.
- Enhanced collaboration: AI-driven collaboration tools facilitate improved knowledge sharing among team members. These tools offer real-time communication, content suggestions, and collaboration platforms that foster a more connected and collaborative work environment.
- Predictive analytics: Within the context of AI in knowledge management, predictive analytics emerges as a powerful capability. AI enables the forecasting of future trends by analyzing historical data. This empowers organizations to anticipate market shifts, changes in customer behavior, and industry trends, facilitating proactive decision-making and strategic planning in the dynamic knowledge management landscape.
- Personalization and user engagement: AI tailors knowledge delivery to individual user preferences, enhancing user engagement. Personalized content recommendations, adaptive learning paths, and user-specific insights contribute to a more user-centric and engaging knowledge management experience.
- Risk mitigation and compliance: AI aids in risk management by recognizing and addressing potential risks linked to knowledge management. Additionally, AI contributes to compliance efforts by ensuring that organizational knowledge is managed in accordance with industry regulations and standards.
- Cost reduction and efficiency: In knowledge management, AI significantly impacts cost reduction and efficiency. Automation streamlines tasks that traditionally require substantial time and resources, enabling organizations to allocate resources more effectively, thus enhancing overall efficiency in knowledge processes.
- Strategic innovation: AI-powered knowledge management fosters a culture of innovation by providing a platform for creative thinking and idea generation. Harnessing the collective intelligence of the organization, AI contributes to strategic innovation and adaptability.
The integration of AI in knowledge management brings a host of benefits, ranging from improved information retrieval and automated content curation to enhanced decision support and strategic innovation. These advantages contribute to organizational efficiency, competitiveness, and the ability to harness knowledge as a strategic asset.
AI technologies used in knowledge management
Several AI technologies are used in knowledge management to enhance various aspects of information processing, retrieval, and decision-making. Here are the key AI technologies applied in knowledge management:
Natural Language Processing (NLP)
Natural Language Processing (NLP) plays a pivotal role in knowledge management by transforming how organizations interact with and derive insights from textual information. NLP enables machines to understand, interpret, and generate human-like language, facilitating more intuitive and efficient communication between users and knowledge repositories. In knowledge management, NLP enhances search functionalities by allowing users to pose queries in a natural, conversational manner, improving the precision and relevance of search results. It also aids in the categorization and organization of content, making information more accessible. Additionally, NLP supports the extraction of meaningful insights from unstructured data, such as documents, emails, and articles, contributing to a more comprehensive understanding of organizational knowledge. By bridging the gap between human language and machine comprehension, NLP empowers knowledge management systems to streamline processes, enhance user experiences, and unlock the full potential of textual information within an organization.
Machine Learning (ML)
Machine Learning significantly enhances knowledge management by automating tasks, recognizing patterns, and providing intelligent insights. In the context of knowledge management, ML algorithms analyze vast datasets to identify trends, relationships, and anomalies, enabling organizations to uncover valuable insights from their information repositories. ML plays a crucial role in content recommendation systems, predicting user preferences and suggesting relevant knowledge resources, thereby personalizing the user experience. Additionally, ML contributes to the automation of repetitive tasks, such as document categorization and tagging, streamlining the organization and retrieval of information. Its adaptive learning capabilities enable knowledge management systems to evolve over time, continually improving their accuracy and relevance. By harnessing the power of ML, organizations can transform their knowledge management processes, making them more efficient, data-driven, and aligned with the dynamic needs of the modern business landscape.
Cognitive computing
Cognitive computing redefines knowledge management by simulating human thought processes and augmenting the capabilities of traditional systems. In knowledge management, cognitive computing systems excel at understanding unstructured data, reasoning, and problem-solving. These systems enhance decision support by analyzing complex datasets and providing context-aware insights, facilitating more informed decision-making. Furthermore, cognitive computing contributes to adaptive learning within knowledge repositories, continuously refining their understanding based on user interactions and feedback. Natural Language Processing (NLP) capabilities within cognitive computing enable more advanced and intuitive interactions with knowledge systems, improving communication and accessibility. By integrating cognitive computing, organizations can elevate their knowledge management practices, fostering a more intelligent and responsive environment that adapts to the ever-evolving landscape of information and user needs.
Knowledge graphs
Knowledge graphs are instrumental in transforming knowledge management by creating interconnected representations of information, facilitating more nuanced and comprehensive insights. In knowledge management, knowledge graphs organize data into a structured format, highlighting relationships and dependencies between various entities. This enhances semantic understanding, allowing for more efficient navigation and exploration of knowledge repositories. By mapping the connections between different pieces of information, knowledge graphs enable users to uncover contextual relationships, aiding in the discovery of relevant content. Moreover, they contribute to personalized recommendations and adaptive learning within knowledge systems, ensuring that users receive information aligned with their preferences and needs. Knowledge graphs thus play a pivotal role in making knowledge management more dynamic, interconnected, and responsive to the intricate web of relationships inherent in organizational information.
Robotic Process Automation (RPA)
Robotic Process Automation (RPA) enhances knowledge management by automating repetitive and rule-based tasks, leading to increased efficiency and accuracy. In knowledge management, RPA can be applied to tasks such as data entry, document categorization, content updating, streamlining workflows and reducing manual effort. By automating routine processes, RPA ensures that knowledge repositories are consistently maintained and updated, minimizing the risk of errors. This technology not only enhances the organization and accessibility of information but also allows human resources to focus on more strategic and value-added aspects of knowledge management. RPA thus plays a crucial role in optimizing knowledge management processes, making them more agile, error-resistant, and capable of handling large volumes of data with precision.
Predictive analytics
Predictive analytics transforms knowledge management by leveraging historical data to forecast future trends, behaviors, and insights. In knowledge management, predictive analytics algorithms analyze patterns within vast datasets, enabling organizations to anticipate changes, user preferences, and knowledge trends. This proactive approach assists in strategic decision-making by providing foresight into potential challenges and opportunities. For example, predictive analytics can forecast emerging topics or areas of interest within an organization, allowing for preemptive knowledge curation and preparation. By harnessing predictive analytics, knowledge management systems become more anticipatory and strategic, aligning the organization with future needs and ensuring that the knowledge repository evolves in tandem with changing dynamics in the business environment.
Augmented analytics
Augmented analytics redefines knowledge management by integrating artificial intelligence and machine learning into analytics tools, enhancing the extraction of valuable insights from data. In the context of knowledge management, augmented analytics goes beyond traditional data analysis, automating the process of uncovering patterns, correlations, and actionable insights within knowledge datasets. By automating complex analytical tasks, augmented analytics enables users to quickly derive meaningful conclusions from vast amounts of information, contributing to informed decision-making. This technology also facilitates natural language generation, translating analytical findings into easily understandable narratives. By making analytics more accessible and insights more comprehensible, augmented analytics empowers users to derive deeper value from their knowledge repositories, fostering a data-driven and strategically informed organizational culture.
These AI technologies collectively contribute to the evolution of knowledge management, making information systems more intelligent, adaptive, and capable of extracting valuable insights from the vast amount of data available to organizations.
Launch your project with LeewayHertz!
Streamline and simplify knowledge management with AI! Organize data, extract insights, and empower decision-making with LeewayHertz’s custom AI solutions tailored to your business.
Future trends in AI for knowledge management
The field of AI for knowledge management is dynamic and continually evolving. Several trends are shaping the future of AI in knowledge management, contributing to more intelligent, adaptive, and user-centric systems. Here are some key future trends:
- Explainable AI (XAI): As AI systems become more complex, there’s a growing need for transparency and interpretability. Explainable AI, which provides clear explanations of how AI models make decisions, will become crucial in knowledge management to build trust and facilitate understanding among users.
- Knowledge virtualization: Future knowledge management systems will likely incorporate knowledge virtualization, creating dynamic and interconnected representations of information. This allows users to explore and navigate knowledge in more immersive and visually intuitive ways, enhancing the overall user experience.
- Personalized learning paths: AI will increasingly tailor learning paths and content recommendations based on individual user preferences, performance, and job roles. This personalization fosters continuous learning within organizations, ensuring that knowledge management systems adapt to the unique needs of each user.
- Knowledge graphs evolving into knowledge networks: Knowledge graphs will evolve into more dynamic knowledge networks, capturing complex relationships and dependencies in a broader organizational context. This shift will enable a more holistic representation of knowledge, supporting better decision-making and strategic planning.
- Context-aware AI: AI systems will become more context-aware, understanding the specific context in which users seek information. This will lead to more accurate and relevant responses, improving the overall effectiveness of knowledge management systems.
- Ethical AI practices: The future of AI in knowledge management will involve a growing emphasis on ethical AI practices. Organizations will prioritize responsible AI deployment, ensuring fairness, transparency, and compliance with ethical standards in managing knowledge and user interactions.
Keeping abreast of these trends will be essential for organizations seeking to leverage the full potential of AI in enhancing their knowledge management practices in the future.
Final words
The integration of artificial intelligence with knowledge management marks a pivotal shift for enterprises aiming to maximize their intellectual assets. In an era overwhelmed by vast information volumes, AI stands as a key solution to challenges in data organization, retrieval, and utilization. This synergy enhances information processing efficiency and fosters innovative decision-making.
AI, with its ability to emulate human intelligence and manage large datasets, streamlines workflows and supports informed decision-making. The advancements in natural language processing, predictive analytics, and knowledge virtualization highlight AI’s role in simplifying complex data, making knowledge both accessible and actionable.
The adoption of AI-driven knowledge management systems signals a move towards a more adaptable, intelligent, and collaborative information environment. These systems offer more than just efficiency; they drive innovation, strategic decisions, and a resilient response to ever-changing business scenarios. Despite ongoing challenges, the predominant narrative is one of empowerment, where AI is a key enabler for enterprises to not only manage knowledge effectively but also to excel in an information-centric era. The interaction between AI and knowledge management is shaping a future where organizations transform from mere data holders to dynamic centers of intelligence and creativity.
Elevate your knowledge landscape with AI! Leverage the expertise of Leewayhertz AI experts to transform your enterprise’s knowledge management. Let’s redefine efficiency together!
Start a conversation by filling the form
All information will be kept confidential.
Insights
Fine-tuning pre-trained models for Generative AI applications
Fine-tuning involves training pre-trained models with a specific data set to adapt them to particular domains or tasks, like cancer detection in healthcare.
How to build a generative AI solution: From prototyping to production
With generative AI, companies can unlock unprecedented levels of innovation, efficiency, speed, and accuracy, creating an unbeatable advantage in today’s hyper-competitive marketplace.
How to create a generative video model?
Generative video models are machine learning algorithms generating new video data based on patterns learned from training datasets. Explore more about it!