What is explainable AI? Use cases, benefits, models, techniques and principles
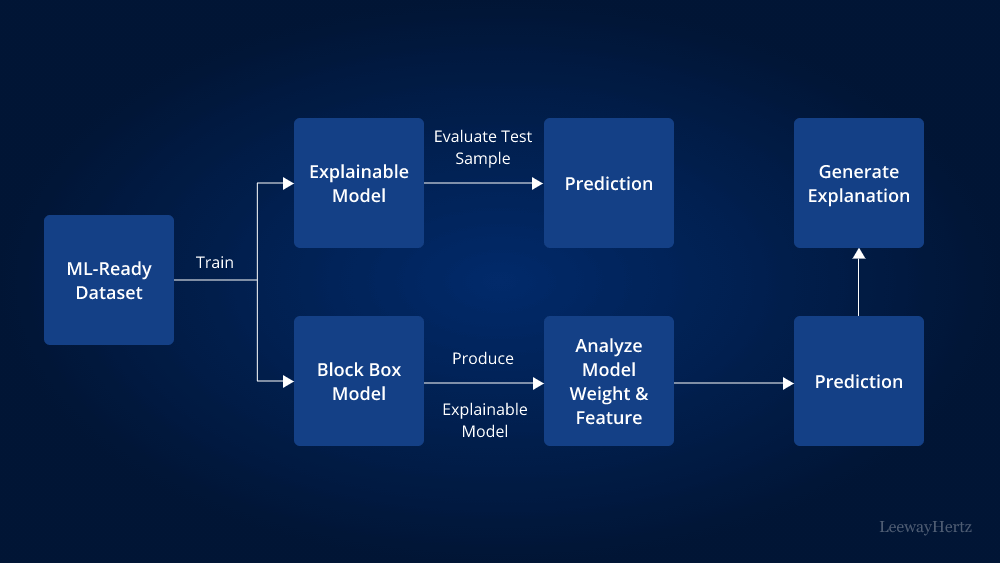
Listen to the article
Artificial Intelligence (AI) has become deeply ingrained in our daily lives, highlighting the substantial interest and reliance on AI technologies. However, despite our dependence on AI, we often find ourselves questioning the decisions made by algorithms in certain situations. Why does a specific algorithm produce a particular outcome? Why does it not consider alternative options? These questions highlight one of the major challenges associated with AI – the lack of explainability, especially in popular algorithms like deep learning neural networks. The absence of explainability hampers our ability to rely on AI systems fully. We need computer systems that not just perform as expected but also transparently explain their decisions.
This lack of explainability causes organizations to hesitate to rely on AI for important decision-making processes. In essence, AI algorithms function as “black boxes,” making their internal workings inaccessible for scrutiny. However, without the ability to explain and justify decisions, AI systems fail to gain our complete trust and hinder tapping into their full potential. This lack of explainability also poses risks, particularly in sectors such as healthcare, where critical life-dependent decisions are involved.
Explainable AI (XAI) stands to address all these challenges and focuses on developing methods and techniques that bring transparency and comprehensibility to AI systems. Its primary objective is to empower users with a clear understanding of the reasoning and logic behind AI algorithms’ decisions. By unveiling the “black box” and demystifying the decision-making processes of AI, XAI aims to restore trust and confidence in these systems. As per reports by Grand View Research, the explainable AI market is projected to grow significantly, with an estimated value of USD 21.06 billion by 2030. It is expected to exhibit a compound annual growth rate (CAGR) of 18.0% from 2023 to 2030. These stats explain the growing popularity of XAI in the ever-growing AI space.
In this article, we delve into the importance of explainability in AI systems and the emergence of explainable artificial intelligence to address transparency challenges. Join us as we explore the methods and techniques to enhance and restore trust and confidence in AI.
- What is explainable AI?
- Explainable AI use cases
- The AI black box concept
- Why is explainability important in AI?
- Explainable models
- Explainability approaches in AI
- Important explainability techniques
- Explainability vs. interpretability in AI
- Principles of explainable AI
- Benefits of explainable AI
What is explainable AI?
Explainable Artificial Intelligence (XAI) refers to a collection of processes and techniques that enable humans to comprehend and trust the outcomes generated by machine learning algorithms. It encompasses methods for describing AI models, their anticipated impact, and potential biases. Explainable AI aims to assess model accuracy, fairness, transparency, and the results obtained through AI-powered decision-making. Establishing trust and confidence within an organization when deploying AI models is critical. Furthermore, AI explainability facilitates adopting a responsible approach to AI development.
As AI progresses, humans face challenges in comprehending and retracing the steps taken by an algorithm to reach a particular outcome. It is commonly known as a “black box,” which means interpreting how an algorithm reached a particular decision is impossible. Even the engineers or data scientists who create an algorithm cannot fully understand or explain the specific mechanisms that lead to a given result.
Understanding how an AI-enabled system arrives at a particular output has numerous advantages. Explainability assists developers in ensuring that the system functions as intended, satisfies regulatory requirements, and enables individuals impacted by a decision to modify the outcome when necessary.
Explainable AI use cases
There are various use cases of explainable AI, some of which are discussed below:
Healthcare
- Understanding anomalies: XAI can explain why an AI system flagged a patient’s X-ray as suspicious, revealing subtle abnormalities undetectable to the human eye. This empowers doctors to prioritize cases and make informed treatment decisions.
- Risk stratification: XAI can explain an AI model’s assessment of a patient’s risk for developing a specific disease. This allows doctors to tailor preventive measures and allocate resources effectively.
- Drug response prediction: XAI can explain why an AI model recommends a specific drug for a patient’s condition. This empowers doctors to consider the rationale behind the suggestion and personalize treatment based on the patient’s response.
- Clinical trial design: XAI can explain how an AI model identifies potential candidates for clinical trials. This knowledge can be used to design more targeted trials with a higher likelihood of success.
Banking
- Loan approvals: Beyond a simple yes/no, XAI can explain the factors influencing loan approval or denial. This empowers banks to address potential biases in models, ensuring fair lending practices and fostering trust with borrowers. Regulatory bodies can also leverage XAI to assess compliance with fair lending regulations.
- Fraud detection: XAI can detail the red flags triggering fraud alerts, enabling banks to improve customer communication. Imagine receiving a notification explaining suspicious activity on your card and the specific details that triggered the alert. This builds trust and fosters collaboration in preventing fraud.
Financial services
- Credit risk assessment: XAI can clarify the factors influencing creditworthiness assessments. This transparency helps financial institutions justify their decisions to borrowers and allows for fairer credit scoring. Additionally, XAI can be used to identify and mitigate potential biases within credit scoring models.
- Robo-advisors: XAI can help with how robo-advisors arrive at investment recommendations. This empowers users to understand the logic behind these recommendations, increasing confidence and trust in automated financial advice. Users can make informed decisions about their investments with greater transparency.
Insurance
- Claims processing: XAI can explain the basis for claim approvals or denials. This transparency fosters trust with policyholders, allowing them to understand the reasoning behind claim decisions. Additionally, XAI can help identify potential biases within claims processing models, ensuring fair treatment for all policyholders.
- Risk pricing: XAI can unveil the factors influencing insurance premiums. This transparency allows insurers to justify their pricing models and address concerns about fairness. Additionally, regulators can leverage XAI to ensure these models are free from bias based on factors like race, gender, or zip code.
- Fraudulent claims detection: XAI can explain how AI identifies fraudulent claims. This transparency allows insurers to communicate effectively with policyholders whose legitimate claims may be flagged as fraudulent. This fosters trust and helps prevent legitimate claims from being denied.
Automobiles
- Autonomous vehicle decision-making: XAI can explain why an autonomous vehicle takes a specific action, such as swerving to avoid an obstacle. This transparency is vital for building trust with passengers and regulators. Imagine receiving an explanation after a near-miss, outlining the factors the car considered during its maneuver.
- Advanced driver-assistance systems (ADAS) functionality: XAI can explain how ADAS features like automatic emergency braking (AEB) or lane departure warning (LDW) identify potential hazards. This empowers drivers to understand the system’s capabilities and limitations, fostering responsible use.
- Predictive maintenance: Based on sensor data, XAI can explain why a vehicle is flagged for a potential issue. The transparency allows drivers to make informed decisions about repairs and maintenance, preventing unnecessary service visits and building trust in the car’s diagnostic capabilities.
Legal
- E-discovery and document review: XAI can explain why AI prioritizes specific documents during e-discovery, a crucial phase of legal proceedings. This transparency allows lawyers to assess the AI’s effectiveness and ensures relevant documents aren’t overlooked.
- Legal research and prediction: XAI can clarify the legal precedents and reasoning behind AI-powered case recommendations. This empowers lawyers to understand the AI’s logic and make informed decisions about their legal cases.
- Algorithmic bias: XAI can help identify potential biases within AI models used for tasks like risk assessment for pretrial release. This ensures fair treatment for all individuals within the legal system and promotes ethical AI use.
Travel
- Personalized itineraries: XAI can illustrate how AI tailors travel itineraries based on user behaviour and past travel behavior. Knowing why a specific destination or activity is recommended can enhance the user experience and build trust.
- Price comparison and optimization: XAI can explain how AI compares travel options and suggests the best deals. This transparency empowers travelers to understand the logic behind price recommendations and make informed booking decisions.
- Chatbots and virtual assistants: XAI can show how AI-powered chatbots and virtual assistants answer user queries and provide travel recommendations. Transparency allows users to understand the limitations of these tools and fosters trust in their accuracy.
The AI black box concept
In machine learning, a “black box” refers to a model or algorithm that produces outputs without providing clear insights into how those outputs were derived. It essentially means that the internal workings of the model are not easily interpretable or explainable to humans.
AI black box model focuses primarily on the input and output relationship without explicit visibility into the intermediate steps or decision-making processes. The model takes in data as input and generates predictions as output, but the steps and transformations that occur within the model are not readily understandable.
Understanding how the model came to a specific conclusion or forecast may be difficult due to this lack of transparency. While black box models can often achieve high accuracy, they may raise concerns regarding trust, fairness, accountability, and potential biases. This is particularly relevant in sensitive domains requiring explanations, such as healthcare, finance, or legal applications.
Explainable AI techniques aim to address the AI black-box nature of certain models by providing methods for interpreting and understanding their internal processes. These techniques strive to make machine learning models more transparent, accountable, and understandable to humans, enabling better trust, interpretability, and explainability.
Why is explainability important in AI?
These are five important reasons why machine learning (ML) explainability, or explainable AI, is important:
Accountability
ML models can make incorrect or unexpected decisions, and understanding the factors that led to those decisions is crucial for avoiding similar issues in the future. With explainable AI, organizations can identify the root causes of failures and assign responsibility appropriately, enabling them to take corrective actions and prevent future mistakes.
Trust
Trust is vital, especially in high-risk domains such as healthcare and finance. For ML solutions to be trusted, stakeholders need a comprehensive understanding of how the model functions and the reasoning behind its decisions. Explainable AI provides the necessary transparency and evidence to build trust and alleviate skepticism among domain experts and end-users.
Compliance
Model explainability is essential for compliance with various regulations, policies, and standards. For instance, Europe’s General Data Protection Regulation (GDPR) mandates meaningful information disclosure about automated decision-making processes. Similar regulations are being established worldwide. Explainable AI enables organizations to meet these requirements by providing clear insights into the logic, significance, and consequences of ML-based decisions.
Performance
Explainability can lead to performance improvements. When data scientists deeply understand how their models work, they can identify areas for fine-tuning and optimization. Knowing which aspects of the model contribute most to its performance, they can make informed adjustments and enhance overall efficiency and accuracy.
Enhanced control
Understanding the decision-making process of ML models uncovers potential vulnerabilities and flaws that might otherwise go unnoticed. By gaining insights into these weaknesses, organizations can exercise better control over their models. The ability to identify and correct mistakes, even in low-risk situations, can have cumulative benefits when applied across all ML models in production.
By addressing these five reasons, ML explainability through XAI fosters better governance, collaboration, and decision-making, ultimately leading to improved business outcomes.
Launch your project with LeewayHertz!
We build robust AI systems powered by explainable AI, a type of AI that addresses crucial aspects of transparency, compliance, and risk mitigation, benefitting your business. Partner with us to experience the future of AI!
Explainable models
Some models in machine learning possess characteristics of explainability. Let’s discuss the models:
Linear models
Linear models, such as linear regression and Support Vector Machines (SVMs) with linear kernels, are inherently interpretable. They follow the principle of linearity, where changes in input features have a proportional effect on the output. The equation y = mx + c exemplifies this simplicity, making it easy to understand and explain the relationship between the features and the outcome.
Decision tree algorithms
Decision tree models learn simple decision rules from training data, which can be easily visualized as a tree-like structure. Each internal node represents a decision based on a feature, and each leaf node represents the outcome. Following the decision path, one can understand how the model arrived at its prediction.
Generalized Additive Models (GAM)
GAMs capture linear and nonlinear relationships between the predictive variables and the response variable using smooth functions. They extend generalized linear models by incorporating these smooth functions. GAMs can be explained by understanding the contribution of each variable to the output, as they have an addictive nature.
Although these explainable models are transparent and simple to comprehend, it’s important to remember that their simplicity may restrict their ability to indicate the complexity of some real-world problems.
Additional techniques and tools are required to make them explainable for more complex models like neural networks. There are two main approaches to achieving explainability for complex models:
- Model-agnostic approach: Regardless of complexity, model-agnostic techniques/tools can be employed in any machine learning model. These methods typically analyze the relationship between input features and output predictions. One popular example is Local Interpretable Model-agnostic Explanations (LIME), which provide explanations by approximating the model locally around specific instances.
- Model-specific approach: Model-specific techniques/tools are tailored to a particular type of model or a group of models. These approaches leverage the specific characteristics and functions of the model to provide explanations. For example, tree interpreters can be used to understand decision trees or random forests.
It’s important to select the most appropriate approach based on the model’s complexity and the desired level of explainability required in a given context.
Explainability Approaches in AI
Explainability approaches in AI are broadly categorized into global and local approaches.
Global interpretations
Global interpretability in AI aims to understand how a model makes predictions and the impact of different features on decision-making. It involves analyzing interactions between variables and features across the entire dataset. We can gain insights into the model’s behavior and decision process by examining feature importance and subsets. However, understanding the model’s structure, assumptions, and constraints is crucial for a comprehensive global interpretation.
Local interpretations
Local interpretability in AI is about understanding why a model made specific decisions for individual or group instances. It overlooks the model’s fundamental structure and assumptions and treats it like AI black box. For a single instance, local interpretability focuses on analyzing a small region in the feature space surrounding that instance to explain the model’s decision. Local interpretations can provide more accurate explanations, as the data distribution and feature space behavior may differ from the global perspective. The Local Interpretable Model-agnostic Explanation (LIME) framework is useful for model-agnostic local interpretation. By combining global and local interpretations, we can better explain the model’s decisions for a group of instances.
Important explainability techniques
Some of the most common explainability techniques are discussed below:
Shapley Additive Explanations (SHAP)
SHAP is a visualization tool that enhances the explainability of machine learning models by visualizing their output. It utilizes game theory and Shapley values to attribute credit for a model’s prediction to each feature or feature value.
The core concept of SHAP lies in its utilization of Shapley values, which enable optimal credit allocation and local explanations. These values determine how the contribution should be distributed accurately among the features, enhancing the interpretability of the model’s predictions. This enables data science professionals to understand the model’s decision-making process and identify the most influential features. One of the key advantages of SHAP is its model neutrality, allowing it to be applied to any machine-learning model. It also produces consistent explanations and handles complex model behaviors like feature interactions.
Overall, SHAP is widely used in data science to explain predictions in a human-understandable manner, regardless of the model structure, ensuring reliable and insightful explanations for decision-making. It can be used both globally and locally.
Local Interpretable Model-agnostic Explanations (LIME)
LIME is a method for locally interpreting AI black-box machine learning model predictions. It creates a transparent model around the decision space of the black-box model’s predictions. LIME generates synthetic data by perturbing individual data points and trains a glass-box model on this data to approximate the behavior of the black-box model. By analyzing the glass-box model, LIME provides insights into how specific features influence predictions for individual instances. It focuses on explaining local decisions rather than providing a global interpretation of the entire model.
Partial Dependence Plot (PDP or PD plot)
A PDP is a visual tool used to understand the impact of one or two features on the predicted outcome of a machine-learning model. It illustrates whether the relationship between the target variable and a particular feature is linear, monotonic, or more complex.
PDP provides a relatively quick and efficient method for interpretability compared to other perturbation-based approaches. In other words, PDP may not accurately capture interactions between features, leading to potential misinterpretations. Furthermore, PDP is applied globally, providing insights into the overall relationship between features and predictions. It does not offer a localized interpretation for specific instances or observations within the dataset.
Morris sensitivity analysis
The Morris method is a global sensitivity analysis that examines the importance of individual inputs in a model. It follows a one-step-at-a-time approach, where only one input is varied while keeping others fixed at a specific level. This discretized adjustment of input values allows for faster analysis as fewer model executions are required.
The Morris method is particularly useful for screening purposes, as it helps identify which inputs significantly impact the model’s output and are worthy of further analysis. However, it must be noted that the Morris method does not capture non-linearities and interactions between inputs. It may not provide detailed insights into complex relationships and dependencies within the model.
Like other global sensitivity analysis techniques, the Morris method provides a global perspective on input importance. It evaluates the overall effect of inputs on the model’s output and does not offer localized or individualized interpretations for specific instances or observations.
Accumulated Local Effects (ALE)
ALE is a method used to calculate feature effects in machine learning models. It offers global explanations for both classification and regression models on tabular data. It overcomes certain limitations of Partial Dependence Plots, another popular interpretability method. ALE does not assume independence between features, allowing it to accurately capture interactions and nonlinear relationships.
Only on a global scale can ALE be applied, and it provides a thorough picture of how each attribute and the model’s predictions connect throughout the entire dataset. It does not offer localized or individualized explanations for specific instances or observations within the data. ALE’s strength lies in providing comprehensive insights into feature effects on a global scale, helping analysts identify important variables and their impact on the model’s output.
Anchors
Anchors are an approach used to explain the behavior of complex models by establishing high-precision rules. These anchors serve as locally sufficient conditions that guarantee a specific prediction with high confidence.
Unlike global interpretation methods, anchors are specifically designed to be applied locally. They focus on explaining the model’s decision-making process for individual instances or observations within the dataset. By identifying the key features and conditions that lead to a particular prediction, anchors provide precise and interpretable explanations at a local level.
The nature of anchors allows for a more granular understanding of how the model arrives at its predictions. It enables analysts to gain insights into the specific factors influencing a decision in a given context, facilitating transparency and trust in the model’s outcomes.
Contrastive Explanation Method (CEM)
The Contrastive Explanation Method (CEM) is a local interpretability technique for classification models. It generates instance-based explanations regarding Pertinent Positives (PP) and Pertinent Negatives (PN). PP identifies the minimal and sufficient features present to justify a classification, while PN highlights the minimal and necessary features absent for a complete explanation. CEM helps understand why a model made a specific prediction for a particular instance, offering insights into positive and negative contributing factors. It focuses on providing detailed explanations at a local level rather than globally.
Global Interpretation via Recursive Partitioning (GIRP)
GIRP is a method that interprets machine learning models globally by generating a compact binary tree of important decision rules. It uses a contribution matrix of input variables to identify key variables and their impact on predictions. Unlike local methods, GIRP provides a comprehensive understanding of the model’s behavior across the dataset. It helps uncover the primary factors driving model outcomes, promoting transparency and trust.
Scalable Bayesian Rule Lists (SBRL)
Scalable Bayesian Rule Lists (SBRL) is a machine learning technique that learns decision rule lists from data. These rule lists have a logical structure, similar to decision lists or one-sided decision trees, consisting of a sequence of IF-THEN rules. SBRL can be used for both global and local interpretability. On a global level, it identifies decision rules that apply to the entire dataset, providing insights into overall model behavior. On a local level, it generates rule lists for specific instances or subsets of data, enabling interpretable explanations at a more granular level. SBRL offers flexibility in understanding the model’s behavior and promotes transparency and trust.
Tree surrogates
Tree surrogates are interpretable models trained to approximate the predictions of black-box models. They provide insights into the behavior of the AI black-box model by interpreting the surrogate model. This allows us to draw conclusions and gain understanding. Tree surrogates can be used globally to analyze overall model behavior and locally to examine specific instances. This dual functionality enables both comprehensive and specific interpretability of the black-box model.
Explainable Boosting Machine (EBM)
EBM is an interpretable model developed at Microsoft Research. It revitalizes traditional GAMs by incorporating modern machine-learning techniques like bagging, gradient boosting, and automatic interaction detection. The Explainable Boosting Machine (EBM) is a generalized additive model with automatic interaction detection, utilizing tree-based cyclic gradient boosting. EBMs offer interpretability while maintaining accuracy comparable to the AI black box models. Although EBMs may have longer training times than other modern algorithms, they are highly efficient and compact during prediction.
Supersparse Linear Integer Model (SLIM)
SLIM is an optimization approach that addresses the trade-off between accuracy and sparsity in predictive modeling. It uses integer programming to find a solution that minimizes the prediction error (0-1 loss) and the complexity of the model (l0-seminorm). SLIM achieves sparsity by restricting the model’s coefficients to a small set of co-prime integers. This technique is particularly valuable in medical screening, where creating data-driven scoring systems can help identify and prioritize relevant factors for accurate predictions.
Reverse Time Attention Model (RETAIN)
RETAIN model is a predictive model designed to analyze Electronic Health Records (EHR) data. It utilizes a two-level neural attention mechanism to identify important past visits and significant clinical variables within those visits, such as key diagnoses. Notably, RETAIN mimics the chronological thinking of physicians by processing the EHR data in reverse time order, giving more emphasis to recent clinical visits. The model is applied to predict heart failure by analyzing longitudinal data on diagnoses and medications.
Launch your project with LeewayHertz!
We build robust AI systems powered by explainable AI, a type of AI that addresses crucial aspects of transparency, compliance, and risk mitigation, benefitting your business. Partner with us to experience the future of AI!
Explainability vs. interpretability in AI
Regarding AI/ML methods, interpretability and explainability are often used interchangeably. To assist organizations in selecting the best AI/ML strategy for their unique use case, it is crucial to distinguish between the two. Let’s compare and see the difference:
Interpretability can be defined as the extent to which a business desires transparency and a comprehensive understanding of why and how a model generates predictions. Achieving interpretability involves examining the internal mechanics of the AI/ML method, such as analyzing the model’s weights and features to determine its output. In essence, interpretability involves interpreting the model to gain insights into its decision-making process.
For instance, an economist is constructing a multivariate regression model to predict inflation rates. The economist can quantify the expected output for different data samples by examining the estimated parameters of the model’s variables. In this scenario, the economist has full transparency and can precisely explain the model’s behavior, understanding the “why” and “how” behind its predictions.
However, high interpretability often comes at the expense of performance. When a company aims to achieve optimal performance while maintaining a general understanding of the model’s behavior, model explainability becomes increasingly important.
Explainability refers to the process of describing the behavior of an ML model in human-understandable terms. When dealing with complex models, it is often challenging to fully comprehend how and why the internal mechanics of the model influence its predictions. However, it is possible to uncover relationships between input data attributes and model outputs using model-agnostic methods like partial dependence plots, Shapley Additive Explanations (SHAP), or surrogate models. This enables us to explain the nature and behavior of the AI/ML model, even without a deep understanding of its internal workings.
For instance, consider a news media outlet that employs a neural network to assign categories to various articles. Although the model’s inner workings may not be fully interpretable, the outlet can adopt a model-agnostic approach to assess how the input article data relates to the model’s predictions. Through this approach, they may discover that the model assigns the sports category to business articles that mention sports organizations. While the news outlet may not completely understand the model’s internal mechanisms, they can still derive an explainable answer that reveals the model’s behavior.
When embarking on an AI/ML project, it is essential to consider whether interpretability is required. Model explainability can be applied in any AI/ML use case, but if a detailed level of transparency is necessary, the selection of AI/ML methods becomes more limited.
When dealing with large datasets related to images or text, neural networks often perform well. In such cases, where complex methods are necessary to maximize performance, data scientists may focus on model explainability rather than interpretability.
Interpretability | Explainability | |
Definition | Interpretability refers to model that are inherently interpretable like small decision tree or linear model with a small no. of input variable. | Explainability refers to the process of applying a method that models the output of a more complex model after training of a complex model. |
Method | It examines inner mechanics, model weights and features. | It uses model agnostic methods like PDP, surrogate tree etc. |
Transparency | It is more transparent since interpretability provides comprehensive understanding of “why” and “how” a model generates prediction. | It provides general understanding of models behaviour in human-understandable terms. |
Principles of explainable AI
Four principles guide explainable AI. The first principle states that a system must provide explanations to be considered explainable. The other three principles revolve around the qualities of those explanations, emphasizing correctness, informativeness, and intelligibility. These principles form the foundation for achieving meaningful and accurate explanations, which can vary in execution based on the system and its context. Let’s discuss them one by one.
Explanation
The explanation principle states that an explainable AI system should provide evidence, support, or reasoning about its outcomes or processes. However, the principle doesn’t guarantee the explanation’s correctness, informativeness, or intelligibility. The meaningful and explanation accuracy principles address these factors. The execution and embedding of explanations can vary depending on the system and scenario, allowing for flexibility. To accommodate diverse applications, a broad definition of an explanation is adopted. In essence, the principle emphasizes providing evidence and reasoning while acknowledging the variability in explanation methods.
Meaningfulness
The meaningful principle in explainable AI emphasizes that an explanation should be understood by its intended recipient. Commonalities across explanations can enhance their meaningfulness. For instance, explaining why a system behaved a certain way is often more understandable than explaining why it did not behave in a particular manner. Individual preferences for a “good” explanation vary, and developers must consider the intended audience and their information needs. Prior knowledge, experiences, and psychological differences influence what individuals find important or relevant in an explanation. The concept of meaningfulness also evolves as people gain experience with a task or system. Different groups may have different expectations from explanations based on their roles or relationships to the system. It is crucial to understand the audience’s needs, level of expertise, and the relevance of the question or query to meet the meaningful principle. Measuring meaningfulness is an ongoing challenge, requiring adaptable measurement protocols for different audiences. However, appreciating the context of an explanation supports the ability to assess its quality. By scoping these factors, the execution of explanations can align with goals and be meaningful to recipients.
Explanation accuracy
The explanation and meaningful principles focus on producing intelligible explanations for the intended audience without requiring a correct reflection of the system’s underlying processes. The explanation accuracy principle introduces the concept of integrity in explanations. It is distinct from decision accuracy, which pertains to the correctness of the system’s judgments. Regardless of decision accuracy, an explanation may not accurately describe how the system arrived at its conclusion or action. While established metrics exist for decision accuracy, researchers are still developing performance metrics for explanation accuracy.
Furthermore, the level of detail in an explanation needs to be considered. Simple explanations may be sufficient for certain audiences or purposes, focusing on critical points or providing high-level reasoning. Such explanations may lack the nuances required to characterize the system’s process fully. However, these nuances may be meaningful to specific audiences, such as system experts. This mirrors how humans explain complex topics, adapting the level of detail based on the recipient’s background.
There is a delicate balance between the accuracy and meaningfulness of explanations. This means providing a detailed explanation can accurately represent the inner workings of the AI system, but it might not be easily understandable for all audiences. On the other hand, a concise and simplified explanation can be more accessible, but it may not capture the full complexity of the system. This principle acknowledges the need for flexibility in determining accuracy metrics for explanations, taking into account the trade-off between accuracy and accessibility. It highlights the importance of finding a middle ground that ensures both accuracy and comprehensibility in explaining AI systems.
Launch your project with LeewayHertz!
We build robust AI systems powered by explainable AI, a type of AI that addresses crucial aspects of transparency, compliance, and risk mitigation, benefitting your business. Partner with us to experience the future of AI!
Knowledge limits
The knowledge limits principle acknowledges that AI systems operate within specific boundaries of design and knowledge. It emphasizes the need for systems to identify cases not designed or approved to operate or where their answers may be unreliable. According to this principle, systems avoid providing inappropriate or misleading judgments by declaring knowledge limits. This practice increases trust by preventing potentially dangerous or unjust outputs.
There are two ways in which a system can encounter its knowledge limits. Firstly, when the operation or query falls outside the system’s domain, it can appropriately respond by indicating its inability to provide an answer. For instance, a bird classification system with an image of an apple would recognize the input as non-bird-related and indicate its inability to respond. This serves as both an answer and an explanation. Secondly, a system may have a confidence threshold, and if the confidence in the most likely answer falls below that threshold, it can acknowledge the limitation. For instance, if a blurry image of a bird is submitted, the system may recognize the bird’s presence but identify the image quality as too low to determine its species. An example output could be: “I found a bird in the image, but the image quality is too low to identify it.”
Benefits of explainable AI
Interest in Explainable AI (XAI) is surging as organizations recognize the imperative for insights into the decision-making processes of “black box” AI models. The key advantages of XAI can be distilled into five main benefits:
Enhanced decision-making: By comprehending how to influence predicted outcomes, XAI provides transparent and interpretable explanations for decisions made by AI models. For instance, using the SHAP explainability tool, one can identify the top features influencing customer churn. This information empowers organizations to make strategic changes to products or services, reducing churn.
Accelerated AI optimization: XAI facilitates faster model optimization by offering visibility into performance metrics, key drivers, and accuracy levels. Unlike black box models, where failures can be elusive, XAI enables organizations to pinpoint issues and enhance model performance efficiently.
Trust building and bias reduction: XAI aids in building trust and mitigating bias in AI systems by allowing scrutiny for fairness and accuracy. Explanations provided by XAI unveil patterns detected by the model, enabling MLOps teams to identify errors and assess data integrity, contributing to a more reliable AI ecosystem.
Increased adoption of AI systems: As understanding and trust in machine learning (ML) and AutoML systems grow, the adoption of AI systems by organizations, customers, and partners increases. Transparent AI models powered by XAI support predictive, prescriptive, and augmented analytics, fostering broader acceptance and utilization.
Regulatory compliance assurance: XAI facilitates regulatory compliance by enabling auditing of the reasoning behind AI-based decisions. Compliance with laws becomes more manageable as XAI allows users to understand conclusions drawn about them and the data used in making those conclusions.
XAI enhances decision-making and accelerates model optimization, builds trust, reduces bias, boosts adoption, and ensures compliance with evolving regulations. This comprehensive approach addresses the growing need for transparency and accountability in deploying AI systems across various domains.
Endnote
Explainable AI is vital in addressing the challenges and concerns of adopting artificial intelligence in various domains. It offers transparency, trust, accountability, compliance, performance improvement, and enhanced control over AI systems. While simpler models like linear models, decision trees, and generalized additive models inherently possess explainability, complex models such as neural networks and ensemble models require additional techniques and tools to make them explainable. Model-agnostic and model-specific approaches enable us to understand and interpret the decisions made by complex models, ensuring transparency and comprehensibility.
Explainable AI empowers stakeholders, builds trust, and encourages wider adoption of AI systems by explaining decisions. It mitigates the risks of unexplainable black-box models, enhances reliability, and promotes the responsible use of AI. Integrating explainability techniques ensures transparency, fairness, and accountability in our AI-driven world.
Embrace transparency, trust, and accountability with our robust AI solutions. Contact LeewayHertz’s AI experts for your next project!
Listen to the article
Start a conversation by filling the form
All information will be kept confidential.